Low-Dimensional Text Representations for Sentiment Analysis NLP Tasks
SN Comput. Sci.(2023)
摘要
Natural Language Processing (NLP) is presently among the hottest scientific fields with an enormous growth rate of the relevant research. Sentiment analysis is a popular NLP problem that aims at the automatic identification of the polarity in user reviews, tweets, blog posts, comments, forum discussions and so on. Unfortunately, the natural sparseness of text, along with its intimate high dimensionality renders the direct application of machine/deep learning models problematic. For this reason, the relevant literature contains a wealth of state-of-the-art dimensionality reduction methods that confront these issues. In this paper, we conduct an experimental study on the effects of dimensionality reduction in the area of sentiment classification. More specifically, we consider multiple feature selection and feature extraction techniques and we investigate their impact on the effectiveness and the efficiency of seven state-of-the-art classifiers. The experimental evaluation includes accuracy and execution time measurements on four benchmark datasets with various degrees of reduction aggressiveness. The results indicate that, in most cases, dimensionality reduction has indeed a beneficial impact on the running times, whereas the accuracy sacrifices are usually small. However, we also indicate several exceptions where this observation is not valid. These exceptions are appropriately highlighted and discussed.
更多查看译文
关键词
Sentiment analysis,Opinion mining,Sentiment classification,Text classification,Dimensionality reduction,Feature selection,Feature extraction
AI 理解论文
溯源树
样例
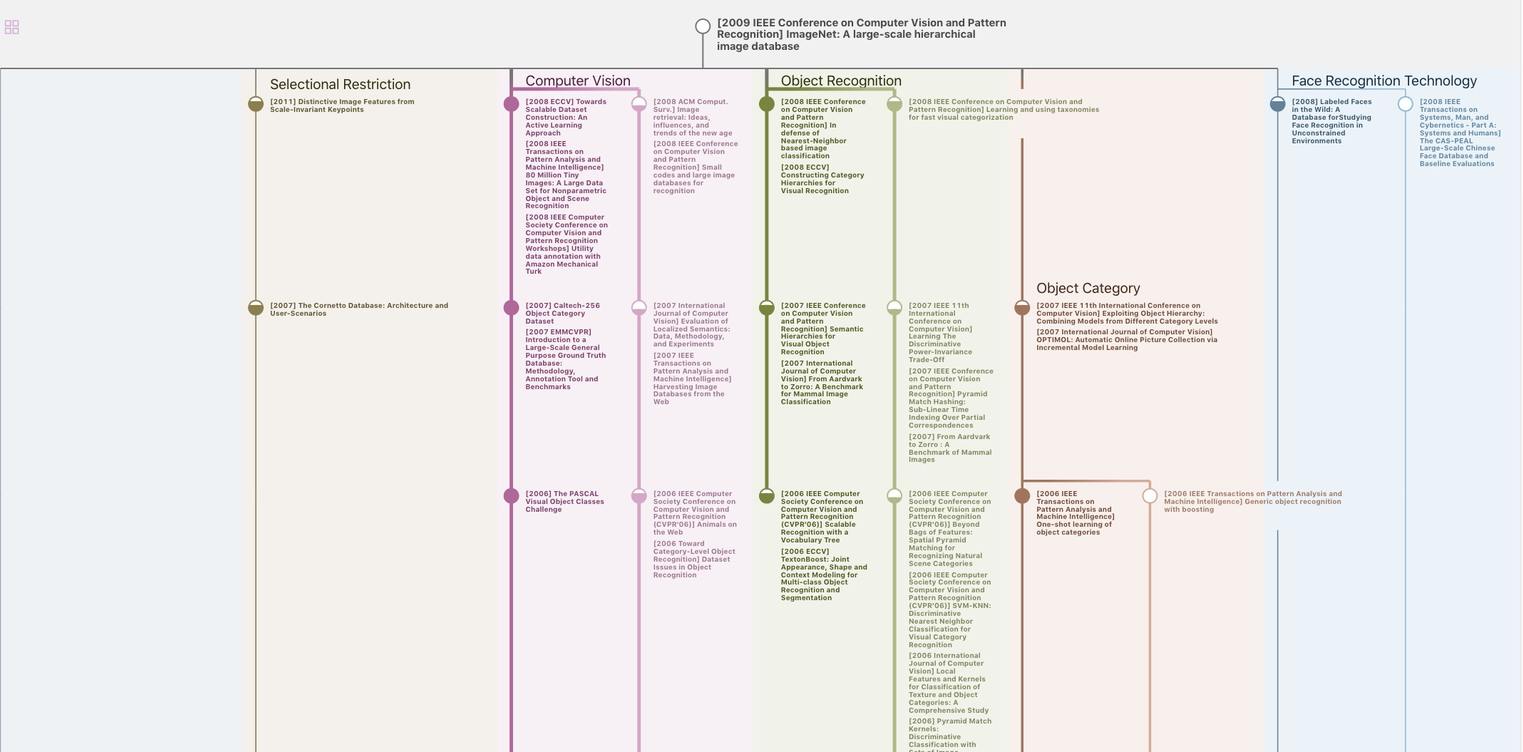
生成溯源树,研究论文发展脉络
Chat Paper
正在生成论文摘要