Strategies for bilingual intent classification for small datasets scenarios
PROCESAMIENTO DEL LENGUAJE NATURAL(2023)
摘要
This paper explores various approaches for implementing bilingual (Spanish and Basque) intent classifiers in cases where limited annotated data is available. Our study examines which fine-tuning strategy is more appropriate in such resource-limited scenarios: bilingual fine-tuning on a small number of manually annotated examples; a monolingual fine-tuning that relies on data augmentation via paraphrasing; or a combination of both. We explore two data augmentation strategies, one based on paraphrasing language models and the other based on back translation. Experiments are conducted on multiple pre-trained language models in order to evaluate the suitability of both monolingual and multilingual language models. The different approaches have been evaluated on two scenarios: i) a real use case over procedures associated with municipal sports services; and ii) a simulated scenario from the multi-domain Facebook Multilingual Task-Oriented dataset. Results show that data augmentation based on back translation is beneficial for monolingual classifiers that rely on pre-trained monolingual language models. Combining bilingual fine-tuning of the multilingual model with the data augmented by back translation outperforms the monolingual model-based approaches for Basque.
更多查看译文
关键词
Neural language models,dialog systems,less-resourced languages,intent classification,data augmentation
AI 理解论文
溯源树
样例
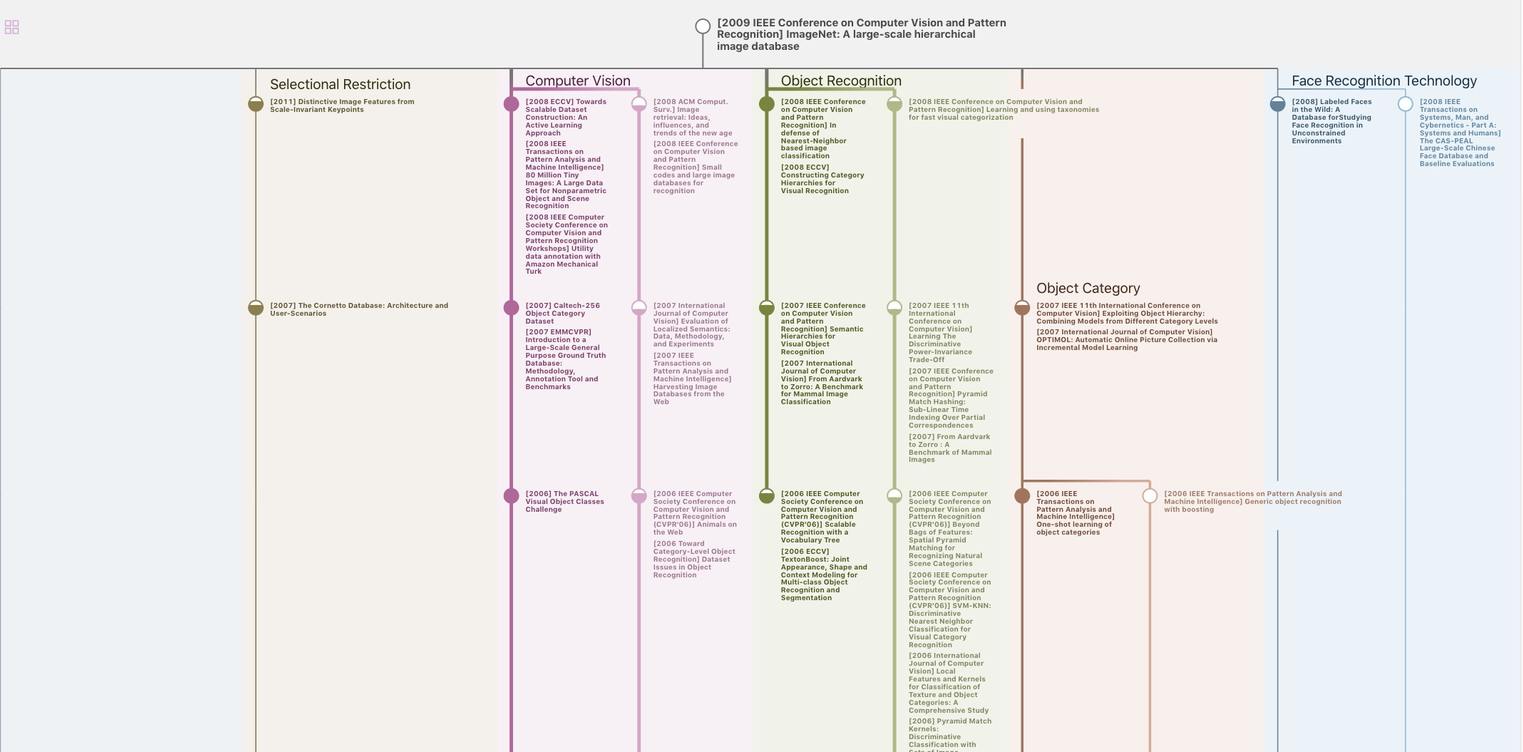
生成溯源树,研究论文发展脉络
Chat Paper
正在生成论文摘要