Radio-Frequency Identification for Drones With Nonstandard Waveforms Using Deep Learning.
IEEE Trans. Instrum. Meas.(2023)
摘要
Identifying noncooperative drones has attracted much attention in recent years, which is critical to public safety. Extensive research has demonstrated that state-of-the-art deep learning (DL) is well-suited to address this problem. This article aims to improve the performance of the DL-based radio-frequency identification (RFI) systems for drones considering some major challenges faced in practical applications, including the unknown drone operating channels, the applicability of drone signal representations to the DL models, and the variation of wireless channels. First, a morphological-filtering-based carrier frequency offset estimation and compensation is proposed to adapt to the switchable operating channel of drones. Second, the applicability of typical drone signal representations revealing different signal features and typical DL models possessing different learning capabilities is investigated, in terms of classification accuracy, training time, and number of parameters. Third, a data augmentation scheme is proposed to generate training signals by injecting various channel distortions, including additive white Gaussian noise (AWGN), multipath, and Doppler shift. Finally, we set up a universal software radio peripheral-based experimental platform to identify 12 types of drones in a real wireless environment. Experimental results validate the improvement of the proposed schemes. The spectrogram combined with the real-valued CNN is recommended for drone classification, which achieves an accuracy of 97% with the least training time and parameters.
更多查看译文
关键词
Carrier frequency offset (CFO) compensation,deep learning (DL),drone classification,signal representation,the variation of wireless channel
AI 理解论文
溯源树
样例
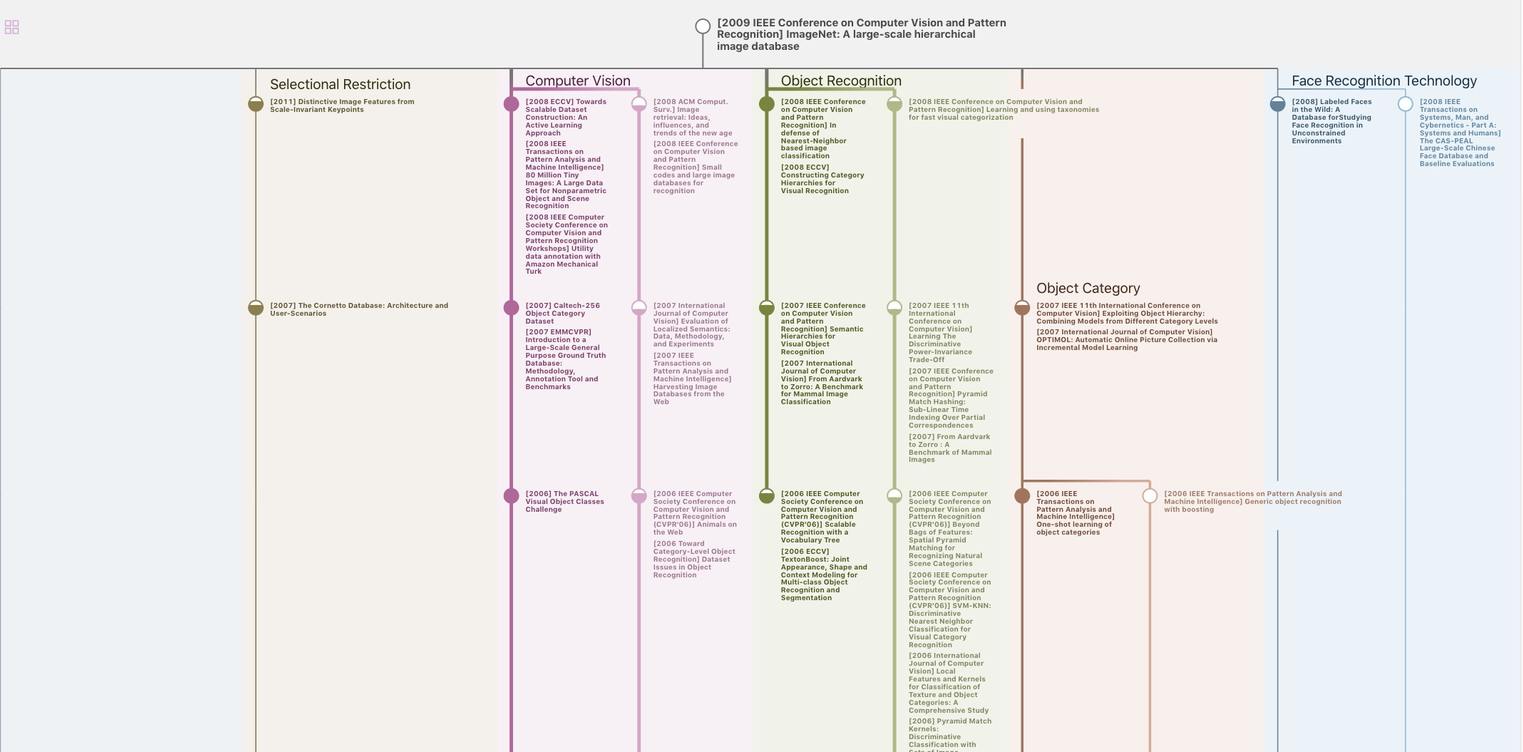
生成溯源树,研究论文发展脉络
Chat Paper
正在生成论文摘要