Semantic Similarity Distance: Towards better text-image consistency metric in text-to-image generation
Pattern Recognit.(2023)
摘要
Generating high-quality images from text remains a challenge in visual-language understanding, with text-image consistency being a major concern. Particularly, the most popular metric R-precision may not accurately reflect the text-image consistency, leading to misleading semantics in generated images. Albeit its significance, designing a better text-image consistency metric surprisingly remains under-explored in the community. In this paper, we make a further step forward to develop a novel CLIP-based metric, Semantic Similarity Distance (SSD), which is both theoretically founded from a distributional viewpoint and empirically verified on benchmark datasets. We also introduce Parallel Deep Fusion Generative Adversarial Networks (PDF-GAN), which use two novel components to mitigate inconsistent semantics and bridge the text-image semantic gap. A series of experiments indicate that, under the guidance of SSD, our developed PDF-GAN can induce remarkable enhancements in the consistency between texts and images while preserving acceptable image quality over the CUB and COCO datasets.
更多查看译文
关键词
Text-to-image,Image generation,Generative adversarial networks,Semantic consistency
AI 理解论文
溯源树
样例
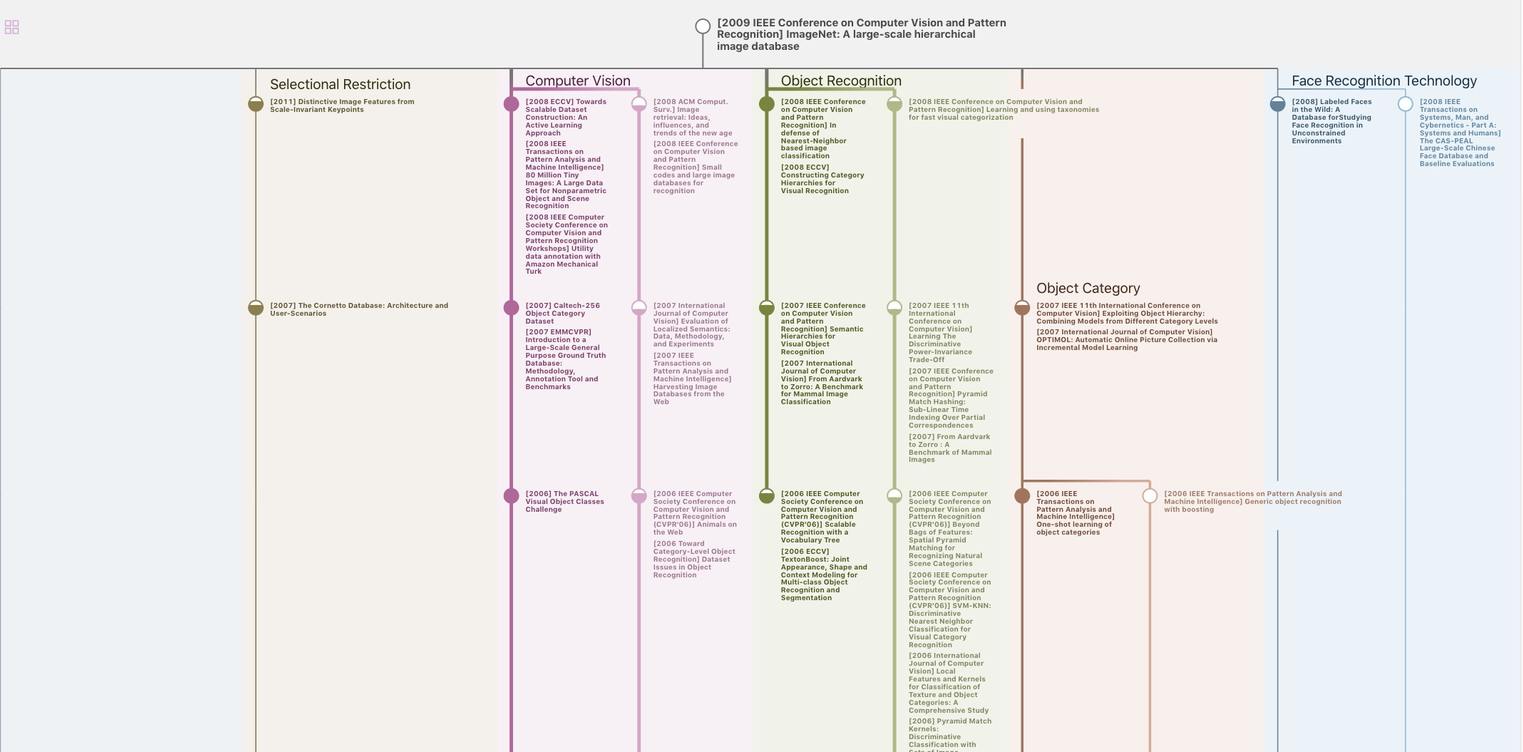
生成溯源树,研究论文发展脉络
Chat Paper
正在生成论文摘要