Improving the Efficiency of Deep Learning Models Using Supervised Approach for Load Forecasting of Electric Vehicles
IEEE Access(2023)
摘要
This research work proposes an Improved Supervised Learning (ISL)-based Deep Neural Network (DNN) for accurately forecasting the load demand of Electric Vehicles (EVs). This work incorporates Gated Recurrent Unit (GRU), Long Short Term Memory (LSTM), Recurrent Neural Network (RNN), Fully Connected (FC), and Convolutional Neural Network (CNN) architectures. The proposed ISL technique enhances prediction performance by refining the training process with additional features and information. Using a real-world EV charging dataset from Boulder City, USA, the simulations demonstrate consistent improvements in the GRU, LSTM, RNN, FC, and CNN models with the proposed ISL technique. Further, the proposed technique reduces the Normalised Root Mean Square Error (NRMSE) and Normalised Mean Absolute Error (NMAE) values. The accurate load demand predictions facilitated by the proposed models with ISL have significant implications for the planning and management of EV charging stations. This enables stakeholders to optimize resource allocation, effectively plan infrastructure capacity, and ensure the sustainable and reliable operation of grids in the face of increasing EV adoption. By leveraging deep learning architectures and incorporating the ISL technique, this research contributes to advancing load forecasting models for EVs, providing practical solutions for efficient management and planning in the evolving electric mobility landscape.
更多查看译文
关键词
Artificial neural network,deep learning,electric vehicles (EV),load forecasting,supervised learning
AI 理解论文
溯源树
样例
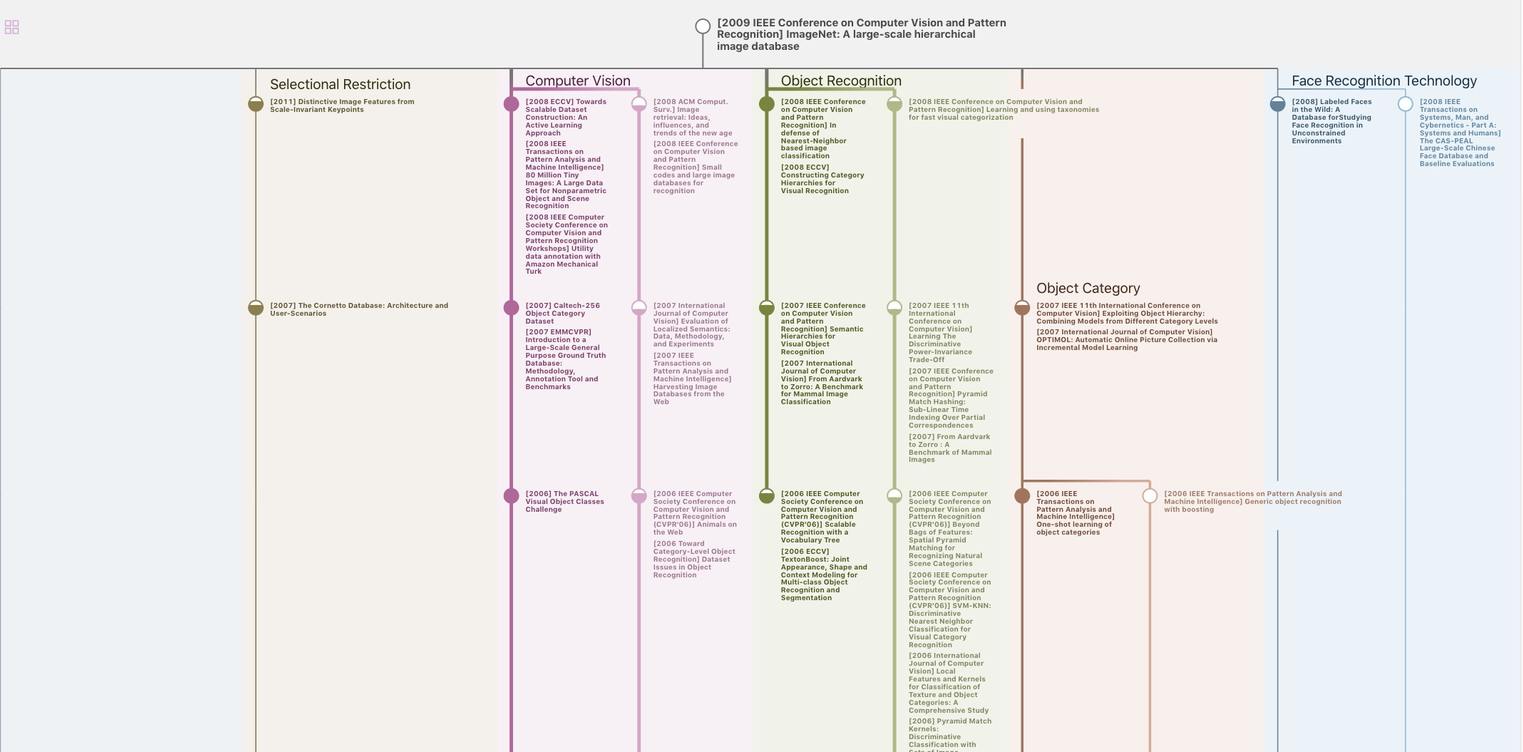
生成溯源树,研究论文发展脉络
Chat Paper
正在生成论文摘要