Learning consistent region features for lifelong person re-identification
PATTERN RECOGNITION(2023)
摘要
The lifelong person re-identification (LRe-ID) model retrieves a person across multiple cameras in continuous data streams and learns new coming datasets incrementally. However, there are two well-known challenges: catastrophic forgetting and generalization loss, which arise due to parameter updates and domain shifts. While there has been encouraging progress in balancing these challenges, few existing methods have addressed them from a unified feature perspective. Inspired by the Complementary Learning Systems theory, an effective framework is proposed to share consistent features and extract discriminative features for each sample. Specifically, this framework consists of Property Region Features, Feature Adaption and Feature Perspicacity. Property Region Features are the parametric representation of consistent region features, Feature Adaption and Feature Perspicacity are responsible for diversity features generation and discriminative features extraction, respectively. Moreover, a cascade knowledge distillation structure is introduced to guarantee Property Feature consistency, and correspondingly, a weighted distillation loss function is designed to prevent generalization loss on current domains caused by overlapping historical knowledge. Extensive experiments conducted on twelve Re-ID datasets, including both rehearsal and no-rehearsal settings, clearly validate the superiority of our method over state-of-the-art competitors, with significantly improved performance.
更多查看译文
关键词
Person re-identification,Lifelong learning,Feature sharing
AI 理解论文
溯源树
样例
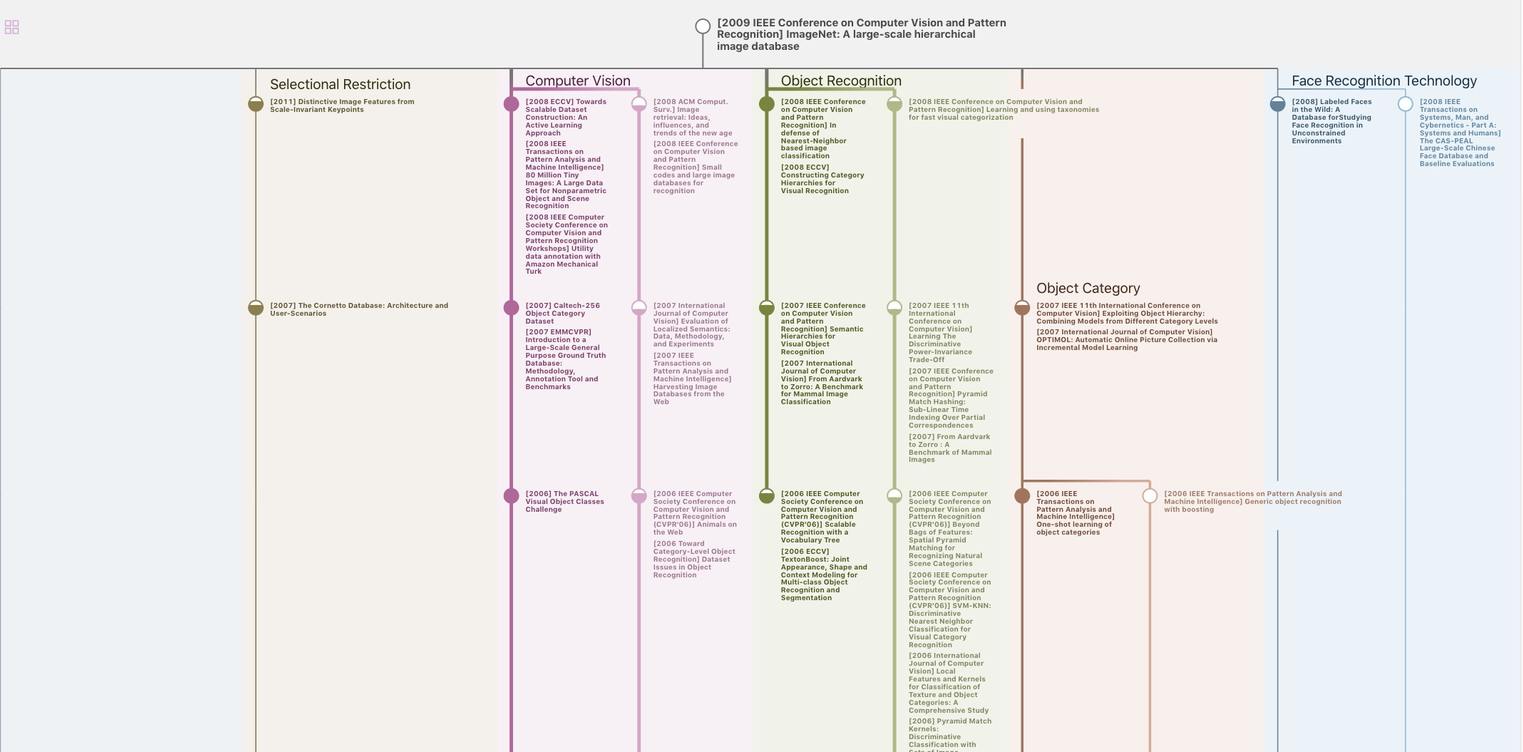
生成溯源树,研究论文发展脉络
Chat Paper
正在生成论文摘要