Estimation of interlayer textural relationships to discriminate the benignancy/malignancy of brain tumors
Pattern Recognit.(2024)
摘要
A computer-aided diagnosis system is a popular tool to predict the risk factors of brain tumors. However, the existing techniques are unable to provide high detection accuracy due to the inability of capturing the hidden features of brain tumors. In this view, the present work decomposes the MR modality images into different layers using non-sub-sampled shearlet transformation (NSST). Following this, the proposed detection pipeline employs adaptive pulse-coupled neural network (A-PCNN) module and a fuzzy c-means (FCM) clustering algorithm for enhancement and segmentation of suspicious regions respectively. Interlayer textural relationships of tumors are estimated in terms of the layer-wise difference of the gray-level cooccurrence matrix (GLCM), similarity indices, and entropy distributions. Thenceforth, those feature coefficients are coded with binary sequences to express the textural homogeneity/randomness of tumors. Finally, these handcrafted multi-scale feature quantifiers are fed to some standard classifiers such as the k-nearest neighbor (kNN) technique, the linear square support vector machine (LS-SVM), and the decision tree (DT) for discriminating the state of benignancy/malignancy of tumors. Experimental results ensure that the proposed detection model shows superior performance compared to existing methods.
更多查看译文
关键词
Advanced PCNN module,Classification,FCM clustering algorithm,Interlayer feature quantifiers,NSST based decomposition
AI 理解论文
溯源树
样例
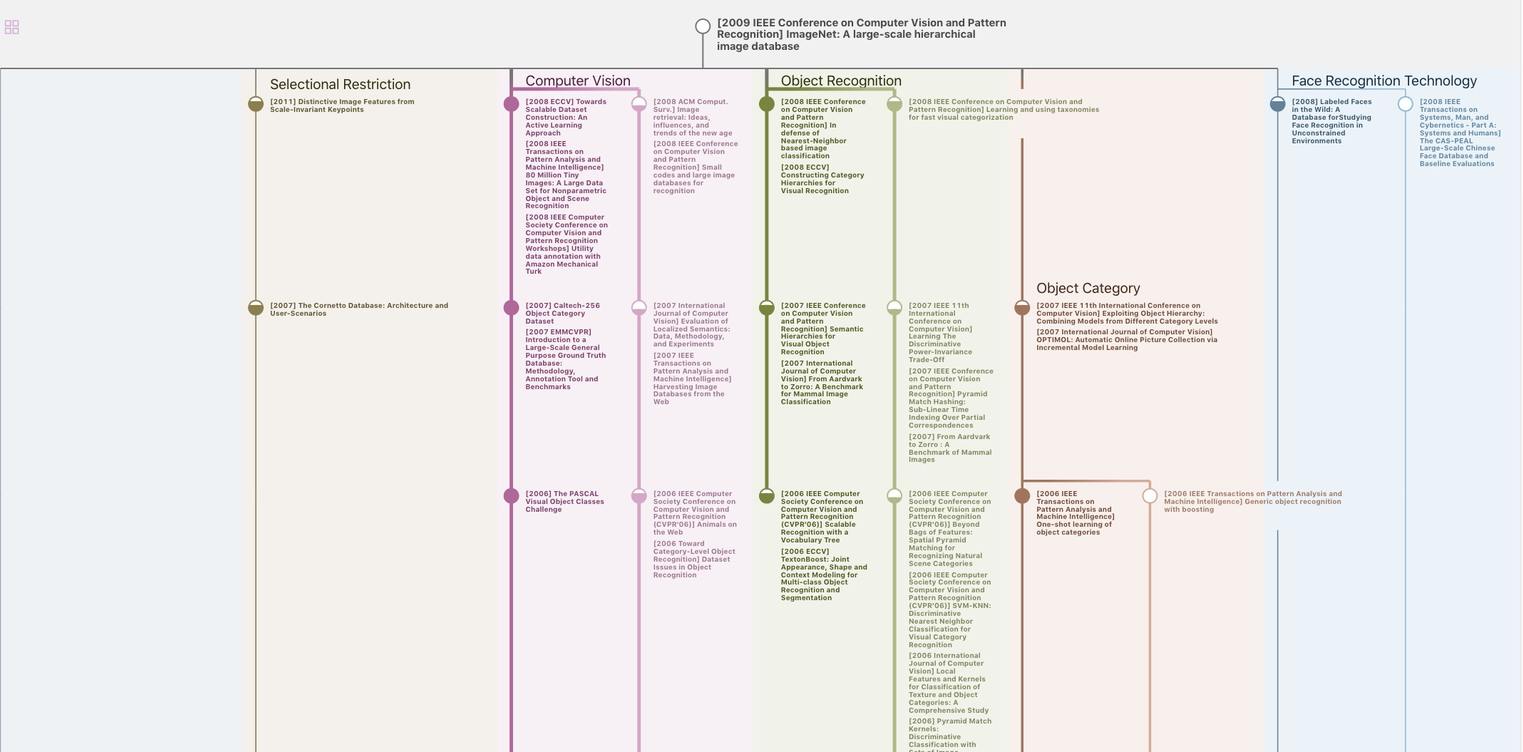
生成溯源树,研究论文发展脉络
Chat Paper
正在生成论文摘要