Deep Learning-Based Resource Allocation Scheme for Heterogeneous NOMA Networks.
IEEE Access(2023)
Abstract
In this paper, we consider downlink power-domain non-orthogonal multiple access (NOMA) in heterogeneous networks (HetNets) and propose resource allocation algorithms for subchannels and transmit powers to improve the sum rate performance while satisfying a minimum data-rate requirement. The proposed subchannel allocation scheme is an iterative algorithm to achieve NOMA gain by selecting the best subchannel from the viewpoint of each user, without the constraint of the number of NOMA users on each subchannel. The proposed power allocation scheme for NOMA is a deep neural network (DNN)-based unsupervised learning algorithm, where the output of the subchannel allocation scheme is used, and unsupervised learning is adopted to reduce the training complexity, as compared to supervised learning. Through simulation, we show that the proposed subchannel allocation scheme provides better sum rates compared to the conventional two-sided matching scheme, and the proposed power allocation scheme achieves a comparable sum rate to the interior point method (IPM).
MoreTranslated text
Key words
Non-orthogonal multiple access,heterogeneous network,deep neural network,subchannel allocation,power allocation,sum rate
AI Read Science
Must-Reading Tree
Example
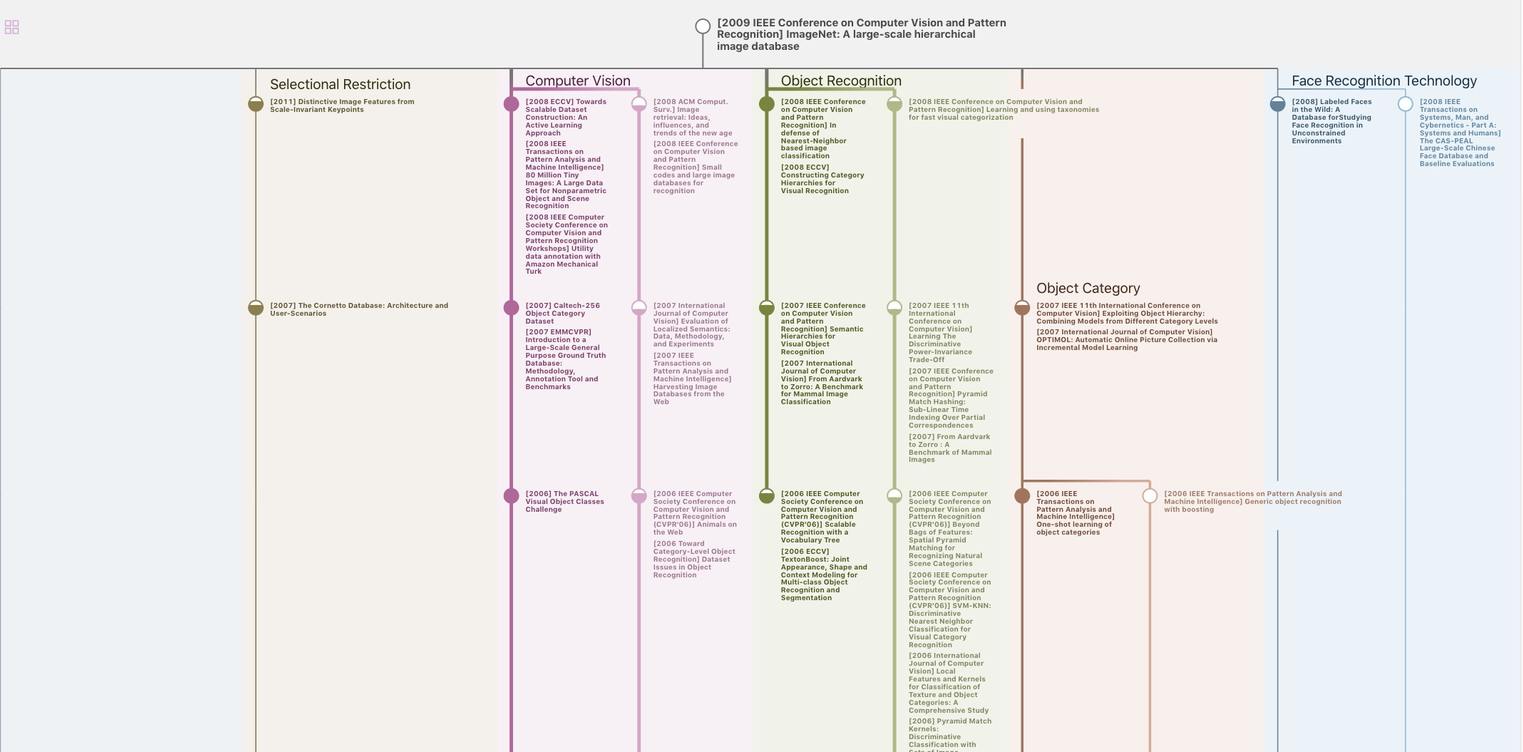
Generate MRT to find the research sequence of this paper
Chat Paper
Summary is being generated by the instructions you defined