A Geometric Structure Based Non Local Mean Image Denoising Algorithm
IEEE Access(2023)
摘要
With the widespread application of image recognition technology, the commercial application value of image denoising is gradually increasing. To optimize the performance of non local mean image denoising algorithms, the similarity of image blocks in this algorithm is now integrated into the evaluation calculation steps of image block direction similarity and geometric information similarity. A neural network structure is used to optimize the mapping function between image blocks, assisting the NLM algorithm in finding the similarity of image blocks. The denoising experimental results for the improved algorithm show that when the noise variance is 25, the normalized peak signal-to-noise ratios of the improved algorithm are 0.58, 0.62, and 0.43, respectively. The improved non local mean image denoising algorithm outperforms other comparative algorithms in terms of information entropy, peak signal-to-noise ratio, and average gradient, which represent the denoising effect. When the noise variance is 45, the normalized structural similarity, mutual information, root mean square error, and absolute error of the algorithm are 0.88, 0.78, 8.2, and 9.7, respectively. The improved non local mean image denoising algorithm performs better than other algorithms in terms of evaluation indicators such as structural similarity, mutual information, root mean square error, and absolute error in preserving original information. It can be seen that the improved algorithm designed in this study has better denoising performance than common methods, and can retain the core information of the original image. It can provide higher quality image denoising services for meteorological prediction, agricultural planting, and other fields.
更多查看译文
关键词
Non local mean image denoising,similarity,noise,directional structure
AI 理解论文
溯源树
样例
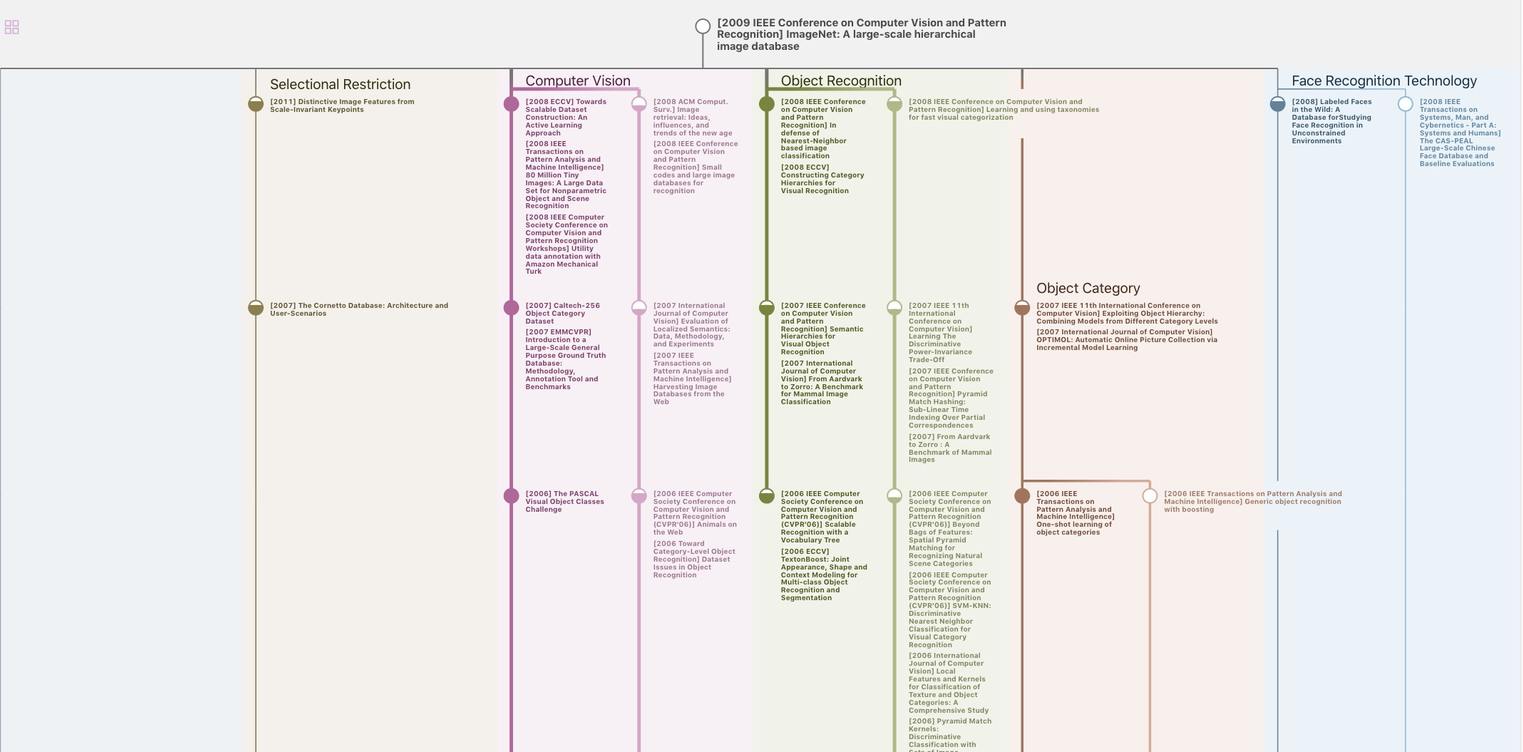
生成溯源树,研究论文发展脉络
Chat Paper
正在生成论文摘要