DA-FSOD: A Novel Data Augmentation Scheme for Few-Shot Object Detection
IEEE ACCESS(2023)
摘要
Deep learning techniques continue to be used in various applications in recent years. However, when it is difficult to obtain adequate training samples, the performance of the depth model will degrade. Although few-shot learning and data enhancement techniques can relieve this dilemma, the diversity of real data is too large to simulate. To tackle this challenge, we study a novel method, Data Augmentation Scheme For Few-Shot Object Detection (DA-FSOD), to improve the efficiency of model training on visual tasks. Specifically, to expand data augmentation space, we build a data augmentation operation pool (DAOP) based on several common-applied image process operations. Then we propose a novel data augmentation scheme, the series and parallel connection scheme, which superimposes the effects of different operations to generate diverse variants. To further explore and utilize the deep feature information, we leverage the semantic information of input image in model and propose imposed semantic data augmentation which augments training set semantically via deep features of augmented variants. The proposed method successfully enhanced the model performance. We validated our approach using extensive experiments on the domain of few-shot object detection. The results showed remarkable gains compared to state-of-the-art methods.
更多查看译文
关键词
Few-shot learning,object detection,data augmentation,semantic information,image processing
AI 理解论文
溯源树
样例
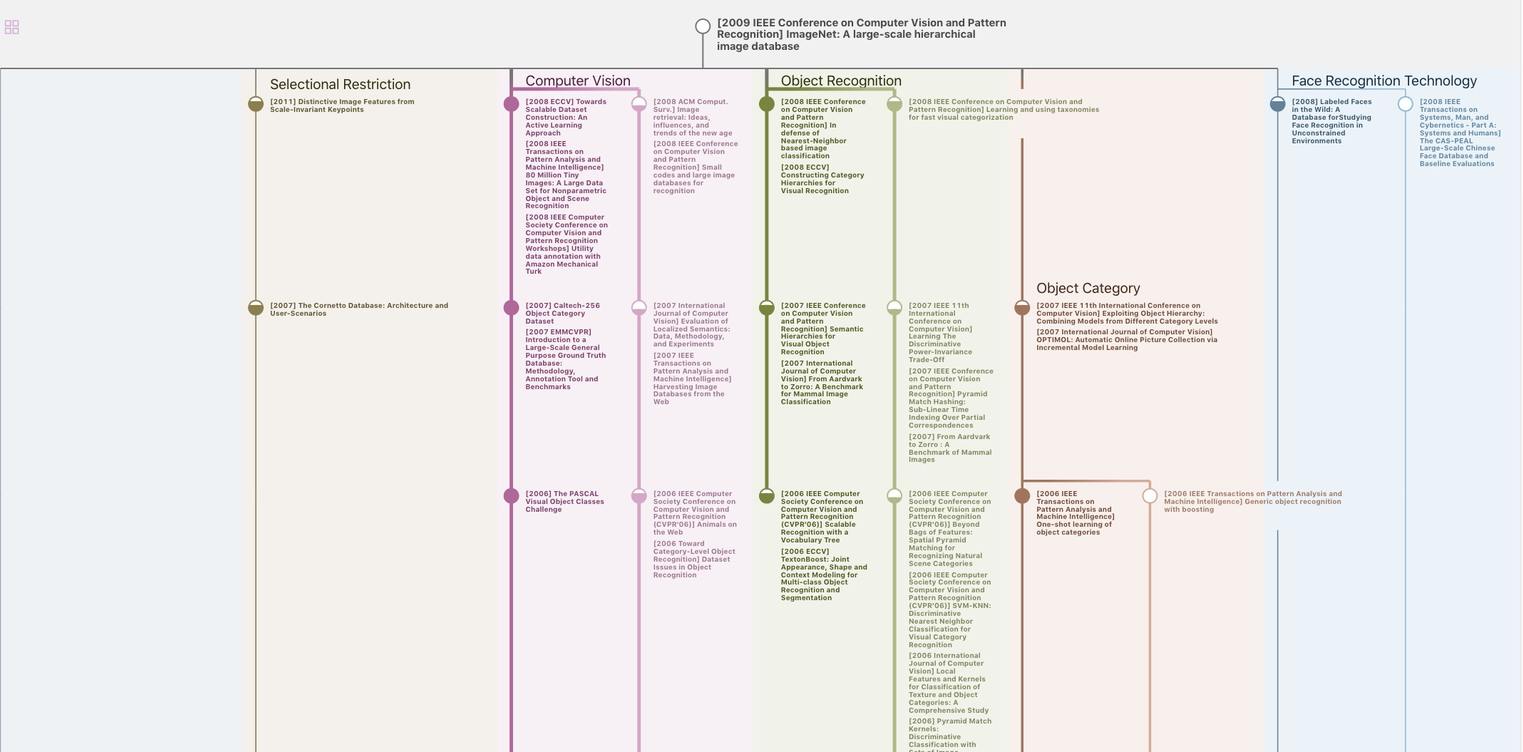
生成溯源树,研究论文发展脉络
Chat Paper
正在生成论文摘要