Velocity-Adaptive Irregular Point Spread Function Deconvolution Imaging Using X-Shaped Denoising Diffusion Filtering.
IEEE Trans. Geosci. Remote. Sens.(2023)
摘要
Most least-squares migration (LSM) imaging methods use iterative gradient update methods to approximate the inverse of the Hessian matrix, but such approximations are not accurate and require a large amount of computational time. The point spread function (PSF) is an optical imaging method, defined as the response of an imaging system to a point source of light. In optics, the image of a complex object is considered to be a degenerate result obtained by convolving the PSF with the real object, which is analogous to the definition of seismic imaging using the Hessian operator. To accurately calculate the inverse of the Hessian matrix, solve the PSF sparsity selection problem, and eliminate migration noise, we propose a velocity-adaptive irregular PSF deconvolution imaging using a Gaussian smoothing X-shaped denoising diffusion filtering operator. The velocity-adaptive irregular grid selection strategy allows for adaptive selection of the grid size based on the velocity of the different layers, ensuring maximum imaging accuracy and avoiding the risk of interference among PSFs. The Gaussian smoothing X-shaped denoising diffusion filtering operator eliminates migration noise and also prevents the introduction of deconvolution noise. We use the Marmousi model and a field dataset to compare the performance of our proposed method with other common methods, demonstrating that our proposed method has clearer seismic events, more balanced amplitude, higher resolution, and higher signal-to-noise ratio than others.
更多查看译文
关键词
imaging,diffusion,velocity-adaptive,x-shaped
AI 理解论文
溯源树
样例
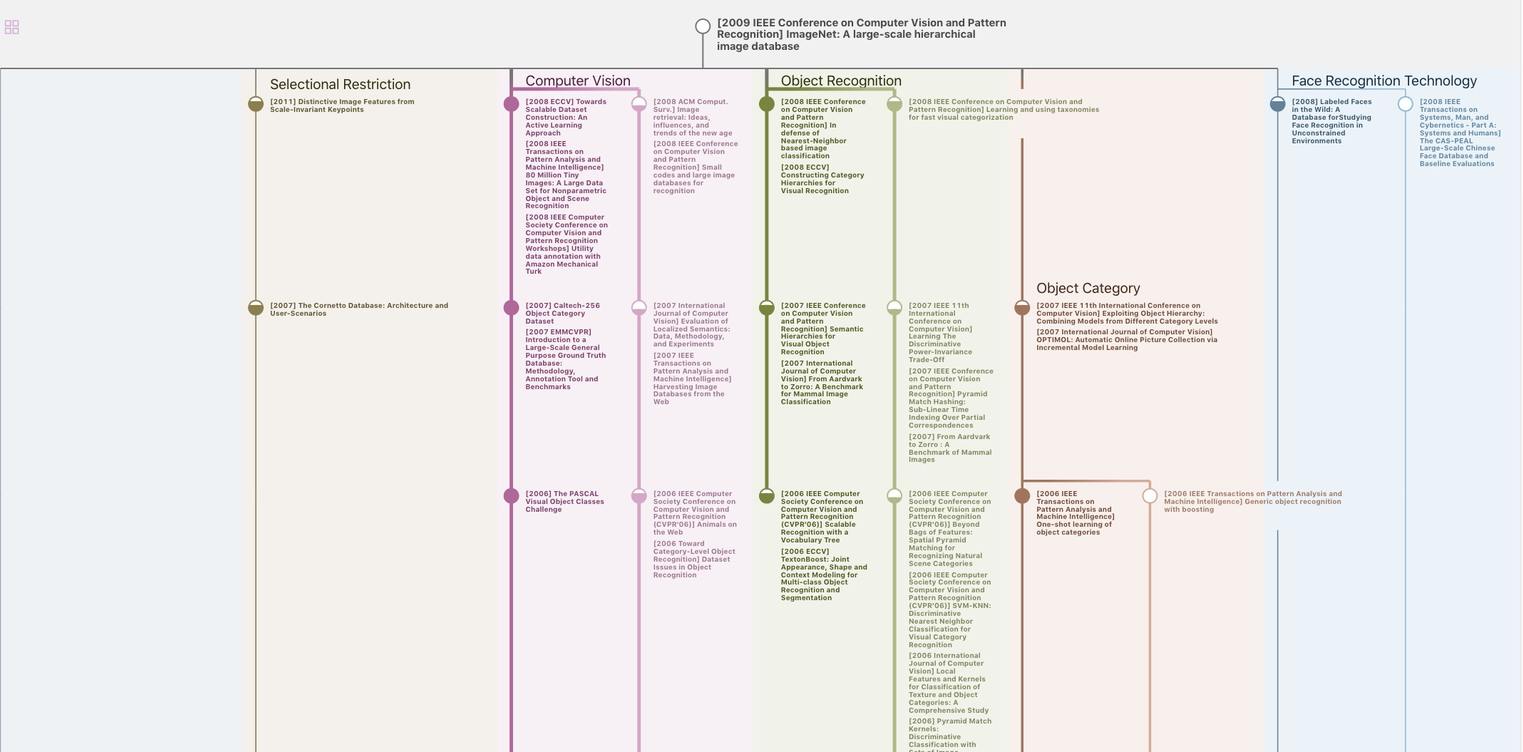
生成溯源树,研究论文发展脉络
Chat Paper
正在生成论文摘要