Seismic Internal Multiple Attenuation Based on Unsupervised Deep Learning With a Local Orthogonalization Constraint.
IEEE Trans. Geosci. Remote. Sens.(2023)
摘要
Internal multiples seriously affecting seismic inversion and imaging need to be suppressed. To suppress internal multiples, we propose an unsupervised deep learning method based on a local orthogonalization constraint (UDL-LOC). The unsupervised deep learning (UDL) consists of one multiattention-based U-net (MA-net), two input data, and one output data. The predicted internal multiples (PIMs), obtained by the adaptive virtual event (AVE) method, and the original data are used as input data. UDL utilizes the excellent nonlinear optimization capability of MA-net to transform PIMs into the estimated true internal multiples that are the output data of UDL. Finally, the de-multiple result can be obtained by subtracting the output data from the original data. The proposed UDL-LOC uses the local orthogonalization constraint (LOC) function as part of the total loss function. The LOC function can help UDL to correctly map PIMs into the true internal multiples with the right amplitudes and phases and prevent the output data from containing residual primaries or leaked internal multiples. Our proposed UDL-LOC method does not require true primaries or true internal multiples as the training dataset. Therefore, our proposed method has a wide range of applications and can solve the problem of missing training datasets. We use the synthetic and land field data examples to demonstrate that our proposed method has a good internal multiple suppression effect.
更多查看译文
关键词
deep learning,self-supervised
AI 理解论文
溯源树
样例
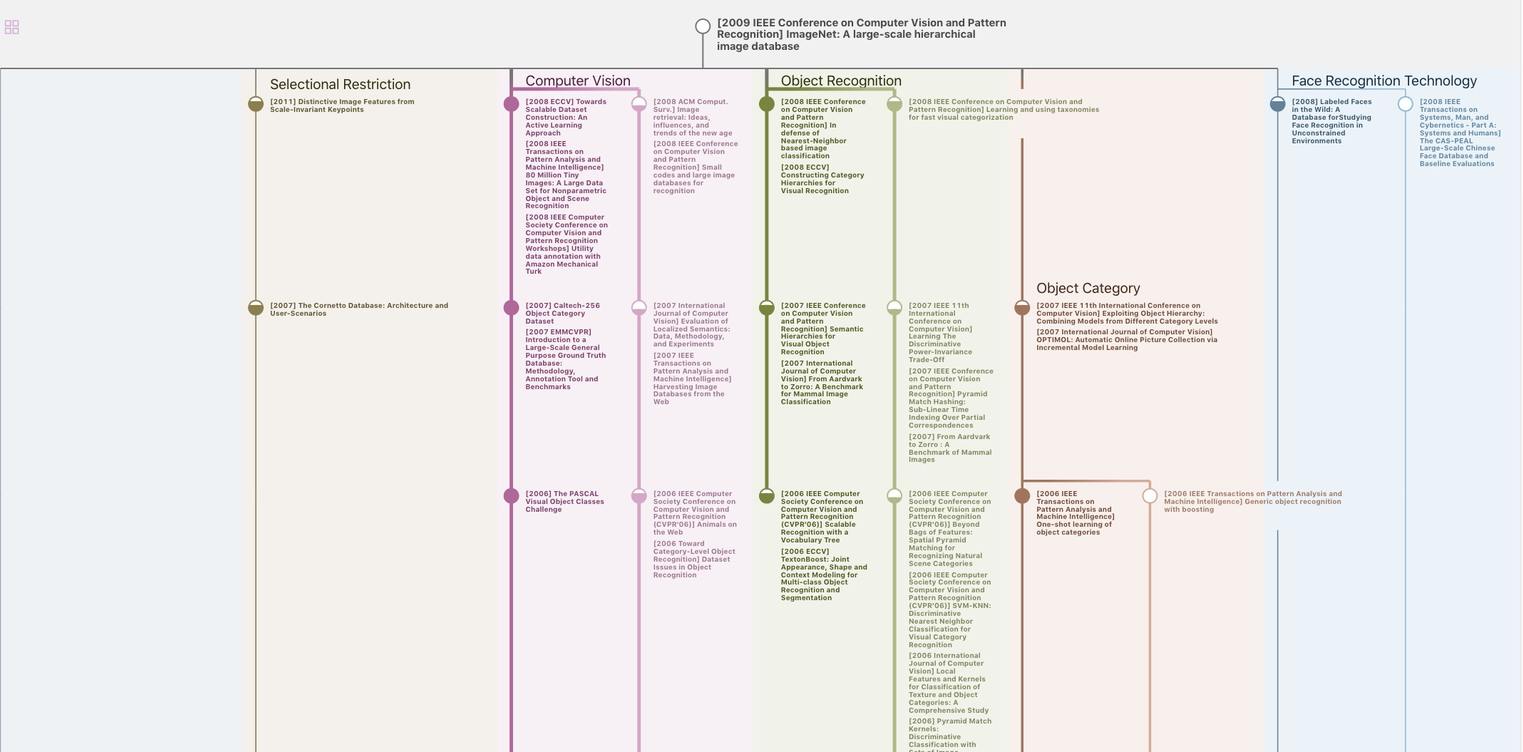
生成溯源树,研究论文发展脉络
Chat Paper
正在生成论文摘要