Intralayer-Connected Spiking Neural Network with Hybrid Training Using Backpropagation and Probabilistic Spike-Timing Dependent Plasticity.
Int. J. Intell. Syst.(2023)
摘要
Spiking neural networks (SNNs) are highly computationally efficient artificial intelligence methods due to their advantages in having a biologically plausible computational framework. Recent research has shown that SNN trained using backpropagation (SNN-BP) exhibits excellent performance and has shown great potential in tasks such as image classification and security detection. However, the backpropagation method limits the dynamics and biological plausibility of the neural models in SNN, which will limit the recognition and simulation performance of SNN. In order to make neural models more similar to biological neurons, this study proposes a leaky integrate-and-fire (LIF) neuron model with dense intralayer connections, as well as efficient forward and backward processes in BP training. The new model will make the interaction between neurons within the layer more frequent, enhancing the intrinsic information exchange capability of SNN. An effective probabilistic spike-timing dependent plasticity (STDP) method is also proposed to reduce the overweighted connections between neurons, as well as a hybrid training method using BP and probabilistic STDP. The training method combines the advantages of BP and STDP to improve the performance of SNN models. An intralayer-connected SNN with hybrid training (ISNN-HY) is proposed with the combination of these improvements. The proposed model was evaluated on three static image datasets and one neuromorphic dataset. The results showed that the performance of ISNN-HY is superior to that of other SNN-BP models. The proposed method also makes it possible to accurately simulate biological neural systems.
更多查看译文
关键词
spiking neural network,backpropagation,hybrid training,intralayer-connected,spike-timing
AI 理解论文
溯源树
样例
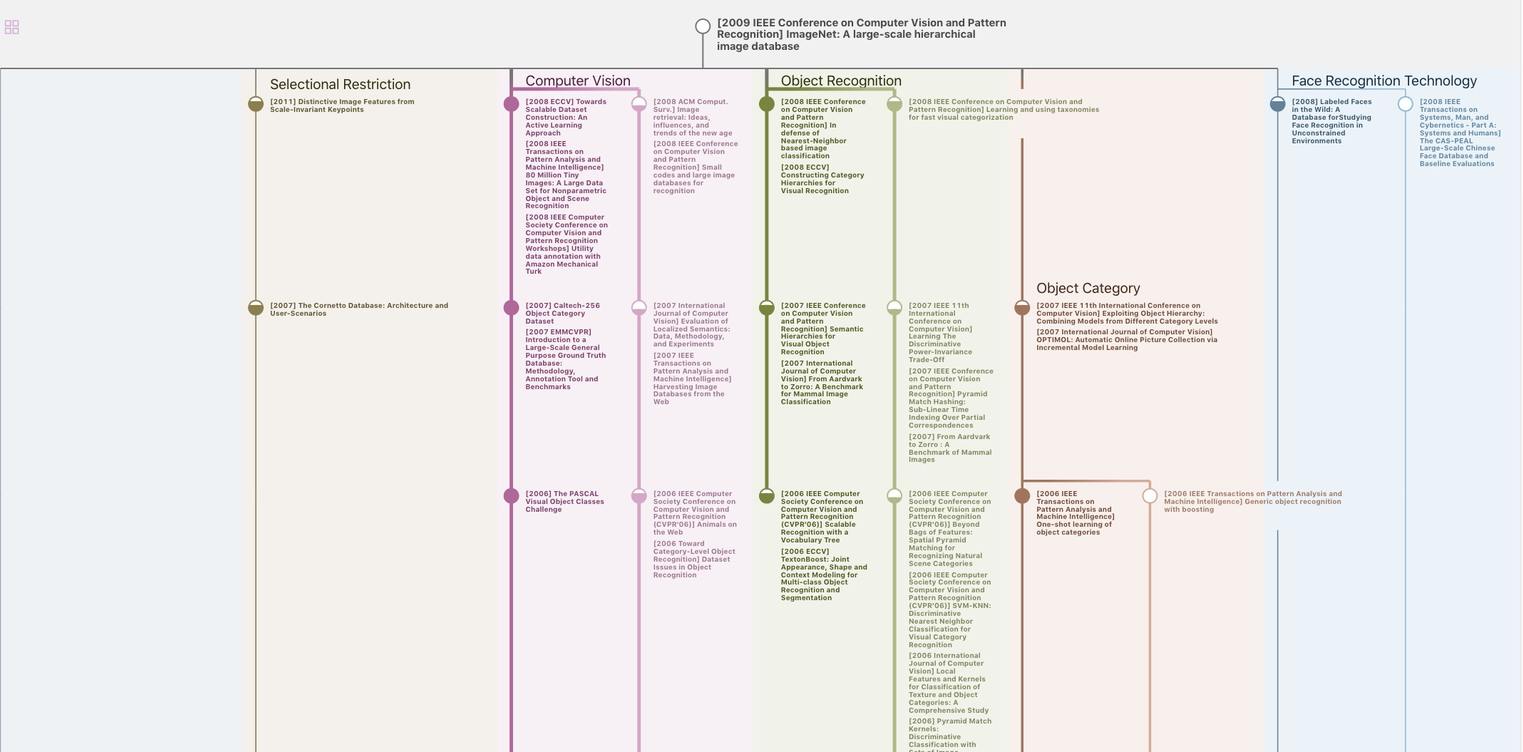
生成溯源树,研究论文发展脉络
Chat Paper
正在生成论文摘要