Approximate fusion of probability density functions using Gaussian copulas.
FUSION(2023)
摘要
Subjective Bayesian estimation perceives probability density functions as expert opinions. Among various rules for combining the opinions, the product and the weighted geometric mean of densities are prominent. Nevertheless, closed-form representations are scarce and non-parametric approaches often suffer from the curse of dimensionality. This paper prospects the fusion of densities represented by non-parametric marginal densities and a parametric Gaussian copula. The explicit reconstruction of the joint densities followed by an optimisation step is avoided. A cheap approximate combination is proposed instead. The combination of marginal densities is tuned by a Gaussian term, while the proposed copula parameter uses moments of the marginal densities. The presented examples illustrate the approximative nature of the approach for non-Gaussian densities and highlight some numerical issues.
更多查看译文
关键词
Gaussian copulas,subjective Bayes,information fusion,nonlinear filtering,analytical approximation
AI 理解论文
溯源树
样例
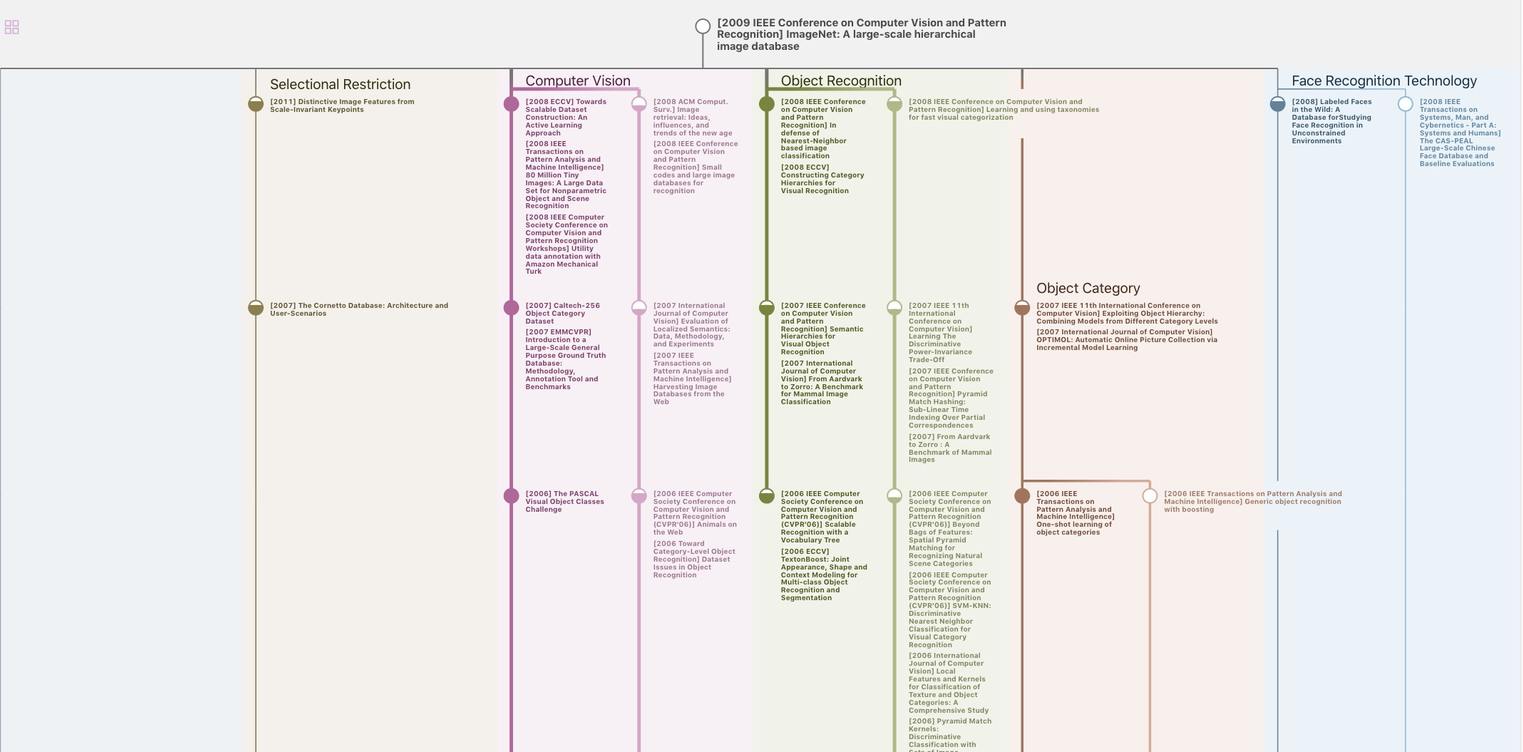
生成溯源树,研究论文发展脉络
Chat Paper
正在生成论文摘要