On-Sensor Online Learning and Classification under 8 KB Memory.
FUSION(2023)
关键词
intelligent sensor processing unit,on-device learning,tinyML,inertial measurement unit,classification,rep counting,inference,template matching,segmentation
AI 理解论文
溯源树
样例
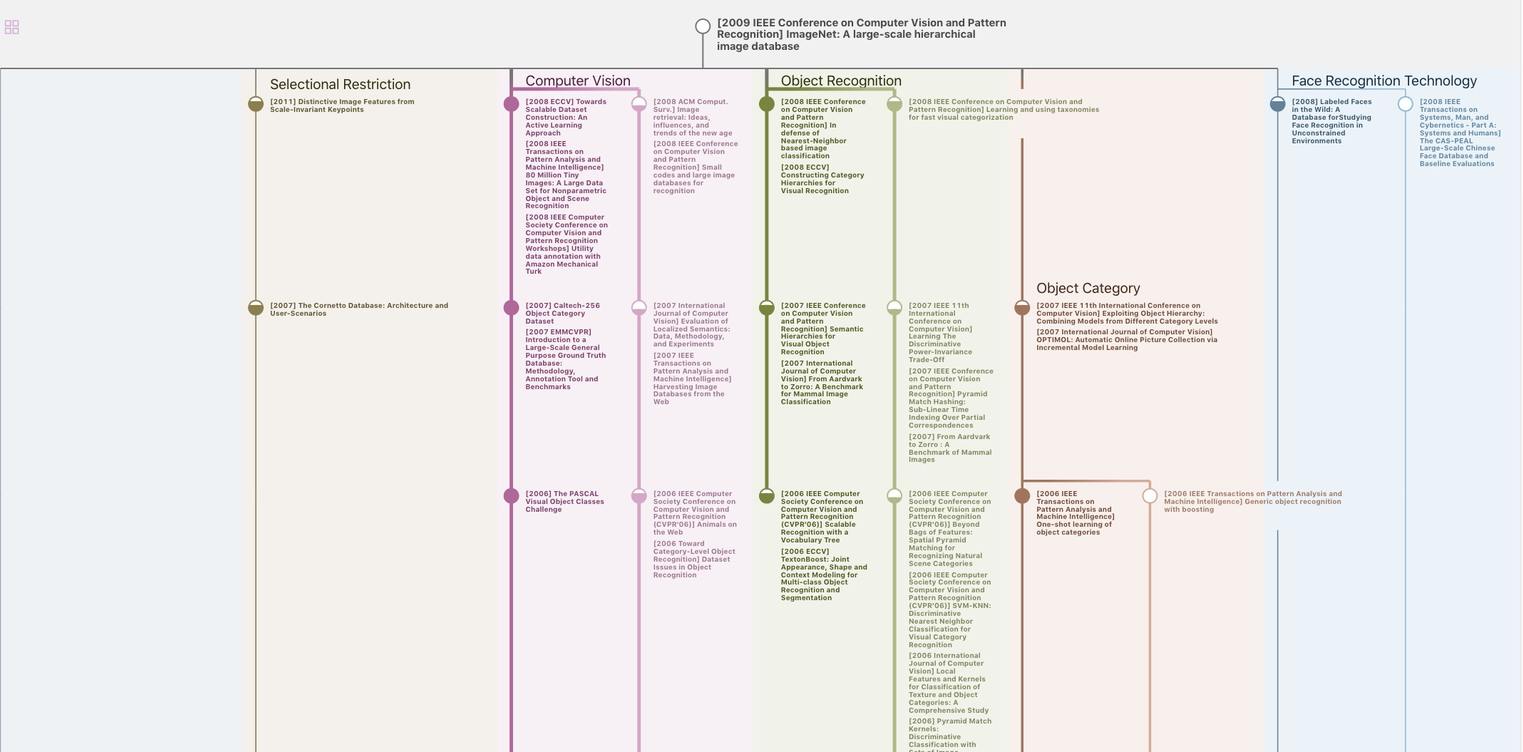
生成溯源树,研究论文发展脉络
Chat Paper
正在生成论文摘要