Assessment and Prediction of a Cyclonic Event: A Deep Learning Model.
ICACDS(2023)
Abstract
Deep learning models based on long short-term memory (LSTM) and bi-directional short-term memory (BLSTM) networks are applied in this present study to predict ocean wave heights for different lead times in the Arabian Sea, during tropical cyclones (TC). The BLSTM model can serve as a computationally effective prediction technique for significant wave height data with higher accuracy in combination with the conventional numerical wave models. In the Arabian Sea, two grids namely G1 (72°E, 9°N) and G2 (71°E, 15.5°N) have been chosen through which OCKHI (29 November-06 December 2017) and NISARGA (01–04 June 2020) cyclones passed and the model computed wave heights are generated. The wave heights are subjected to prediction during extreme conditions using the deep learning models and the accuracy is compared in terms of root mean square error. For grid G1 a time-series is considered from 1979–2017 and for grid G2 a shorter time-series from 2010–2020. The LSTM and BLSTM models are trained with 80% data and tested with various hidden units and epoch values to achieve a lesser error. For the training set, the longer time-series including or not including the TC conditions gave lesser error compared to the shorter time-series. For the 20% testing set, the shorter time-series including the cyclonic data gave more error. Further predictions with different delays or lead times are carried out resulting in an increase in error. This is related to the fact that the power of the model diminutes as the forecast horizon increases.
MoreTranslated text
Key words
cyclonic event,deep learning,deep learning model,prediction
AI Read Science
Must-Reading Tree
Example
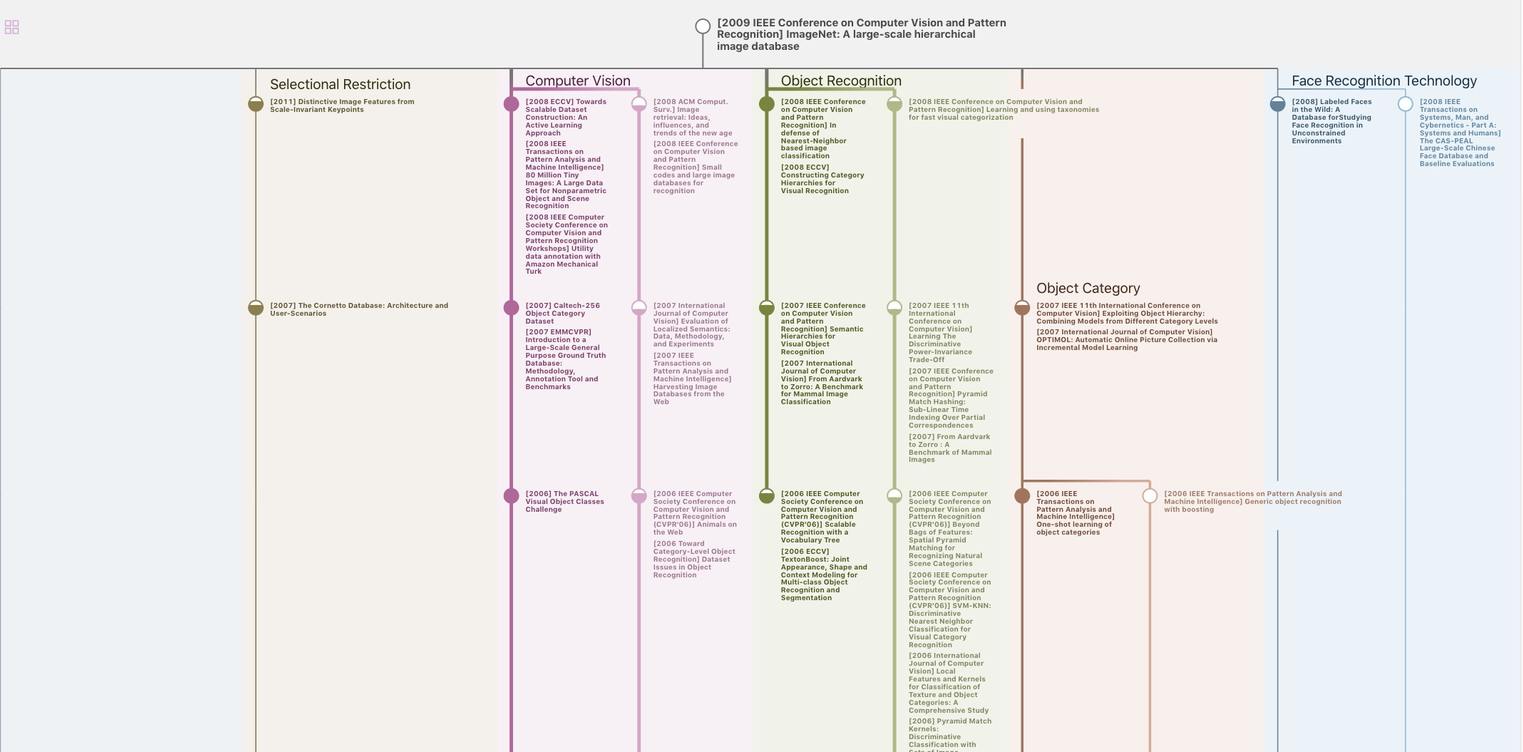
Generate MRT to find the research sequence of this paper
Chat Paper
Summary is being generated by the instructions you defined