A Robust Agatston Score for Coronary Artery Calcium Scoring from Non-ECG-Gated CT with Different Reconstruction Kernels.
ISBI(2023)
摘要
A coronary artery calcium score (CACS) is a vital measure to screen individuals at risk for early coronary heart disease. However, as the main evaluation system of CACS, the Agatston score computed from CT images via HU-thresholding may vary significantly even for the same individual as the protocol of image acquisition changes (e.g., reconstruction kernels). This may harm the compatibility of CACS, when evaluated in different health facilities at different times. To tackle this issue, we propose the robust Agatston score (RAS), wherein we predict the calcification level per pixel via deep learning, rather than directly thresholding the HU value from CT images, as we do for the classic Agatston score. In this way, we make the CACS more robust to the change of acquisition protocols, and let the comparison among CACS from various sources easier. Experimental results show that our method can improve the CACS level accuracy from 64.21% to 95.78%. Code is available at https://github.com/lucas-dw/ras.
更多查看译文
关键词
Coronary Artery Calcium Score, reconstruction kernel, deep learning, CT image, Agatston score
AI 理解论文
溯源树
样例
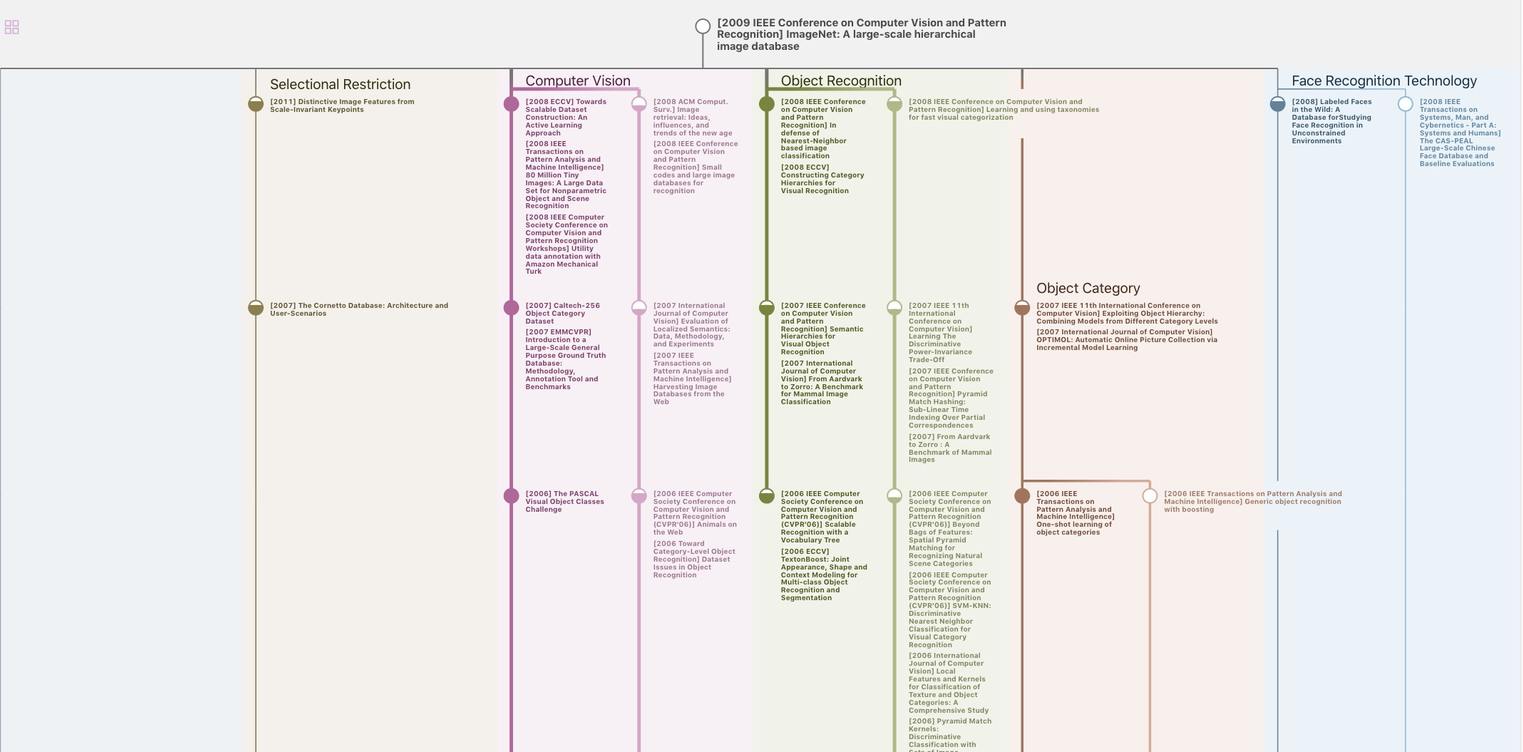
生成溯源树,研究论文发展脉络
Chat Paper
正在生成论文摘要