Local Sequential Features Coupling Global Representation of Dynamic Functional Connectivity Network for Brain Disease Classification
ISBI(2023)
Abstract
Recently, studies have applied advanced machine learning methods, e.g., convolutional neural network (CNN) and transformer architecture, to extract features from functional connectivity networks (FCNs) for brain disease analysis and classification. However, CNN based methods usually extract local features from FCN, ignoring global representation of FCN. Transformer based methods typically extract global features of FCN but are challenging to capture local information of the brain network. To address this problem, in this paper we propose Con-Trans, a novel learning framework that integrates both convolutional operation and transformer for brain disease classification, with functional magnetic resonance imaging (fMRI) data. Experimental results on 174 subjects with resting-state fMRI (rs-fMRI) data from the Alzheimer’s Disease Neuroimaging Initiative (ADNI) demonstrate the effectiveness of the proposed Con-Trans method.
MoreTranslated text
Key words
Functional connectivity network,Convolutional neural network,Transformer,Classification,Brain disease
AI Read Science
Must-Reading Tree
Example
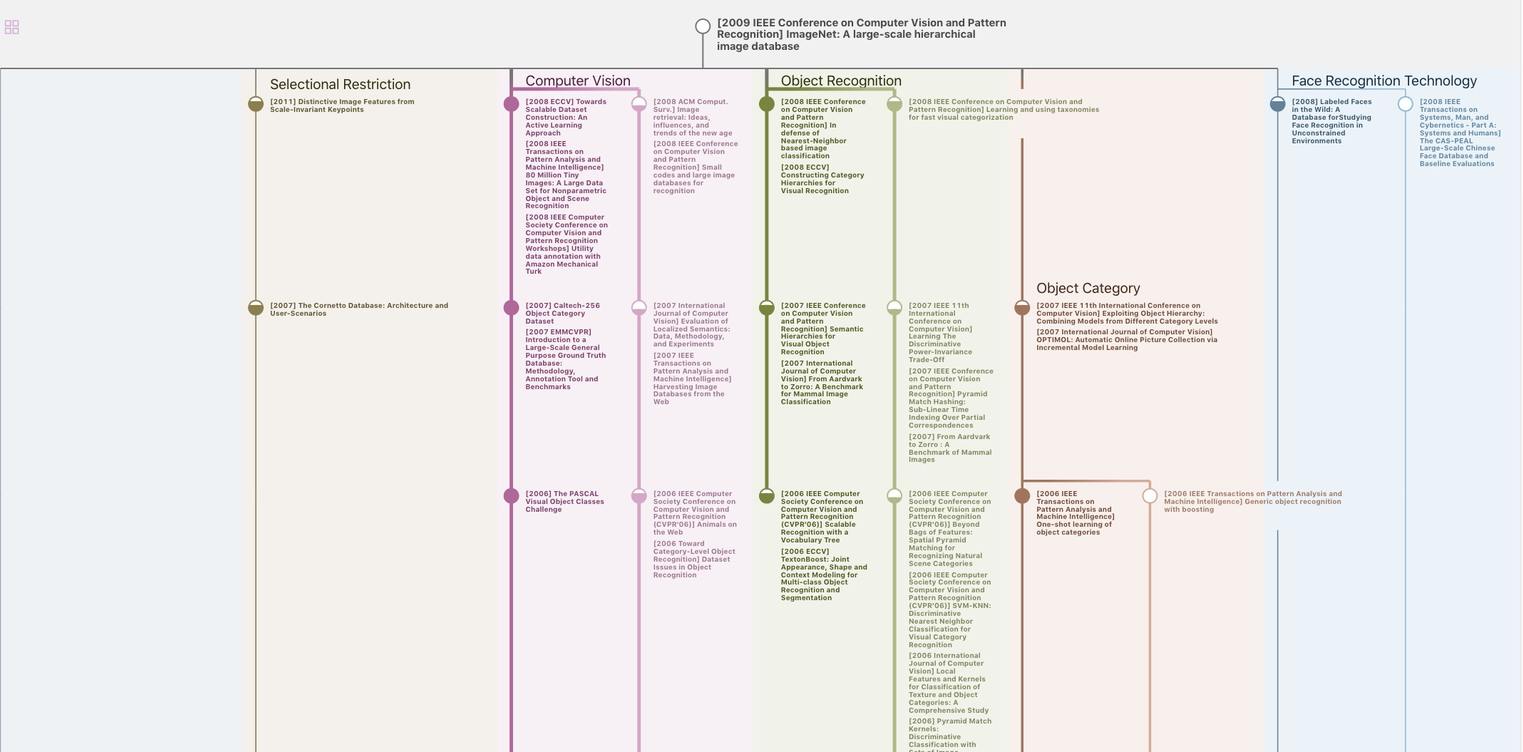
Generate MRT to find the research sequence of this paper
Chat Paper
Summary is being generated by the instructions you defined