Rescon-Net: Transfer Learning Based Nested Model for Cerebrovascular Segmentation with Aneurysm.
ISBI(2023)
Abstract
In the field of cerebral vascular segmentation, existing models have achieved excellent results in normal subjects. However, such models may not be satisfactory in cerebral vessels with aneurysms or malformation in emergency cases, particularly, when the vessels are small, thus resulting in poor segmentation results. We propose a transfer learning strategy based pre-trained model termed ReSCon-Net, using publicly available dataset for cerebrovascular segmentation with aneurysms or malformation in emergency cases. In details, the transfer learning strategy takes advantage of pre-trained models using a large volume of dataset from normal subjects and provides a solution for relatively small clinical datasets of patients. This can be achieved by fine-tuning the parameters in the last layers of the proposed model termed ReSCon-Net, which consists of three blocks: ResMul, DeRes, and REAM. The first two blocks aim to address inadequate extraction of weak vessels by using different convolution sizes to achieve multiscale features; REAM block can enhance the vessel edges by obtaining the probability of a voxel weight corresponding to reverse.
MoreTranslated text
Key words
3D U-Net, multiscale convolution, residual block, transfer learning, attention mechanism
AI Read Science
Must-Reading Tree
Example
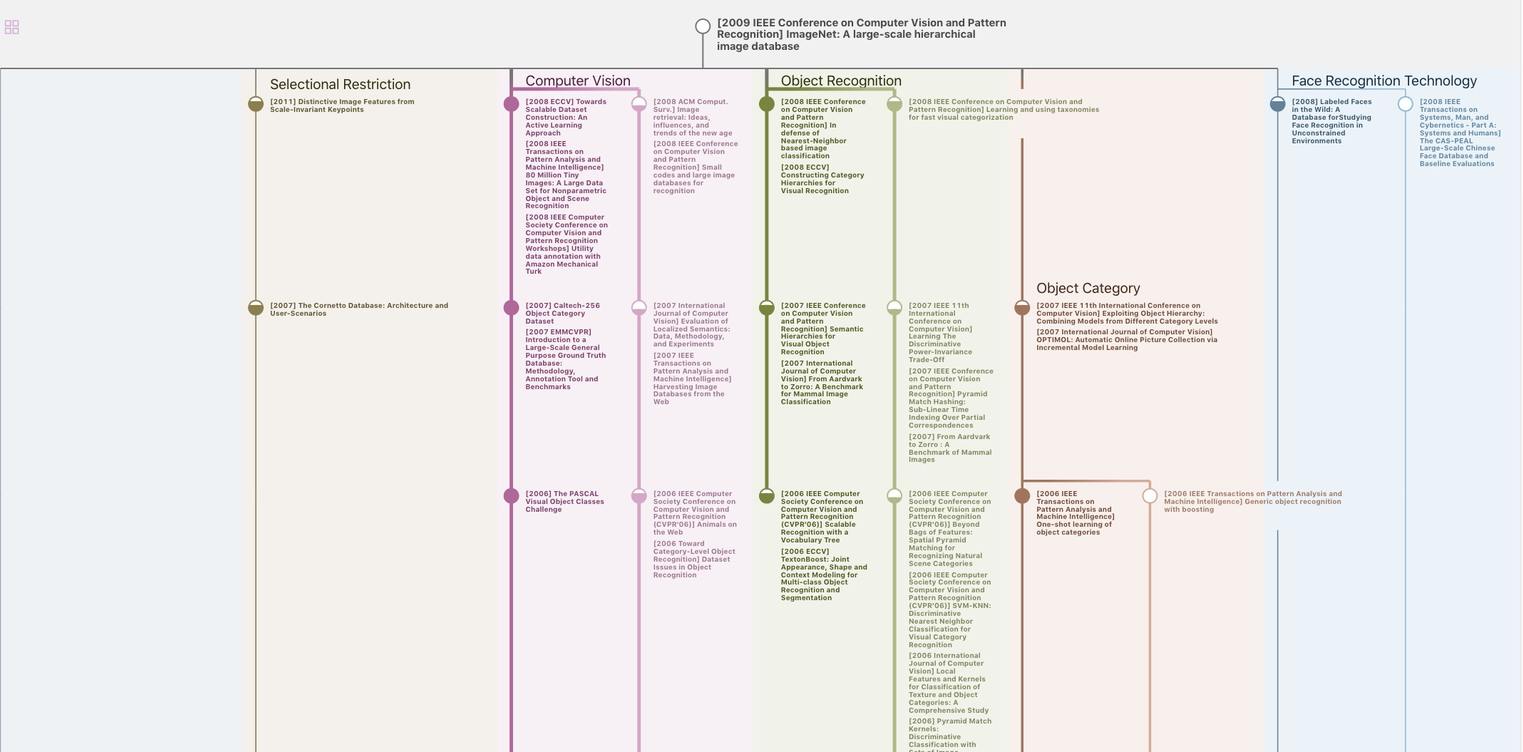
Generate MRT to find the research sequence of this paper
Chat Paper
Summary is being generated by the instructions you defined