Tiny Federated Learning with Bayesian Classifiers.
ISIE(2023)
Abstract
Tiny machine learning (TinyML) represents an emerging research direction that aims to realize machine learning on Internet of Things (IoT) devices. The current TinyML research seems to focus on supporting the deployment of deep learning models on microprocessors, while the models themselves are trained on high performance computers or clouds. However, in the resource/time constrained IoT contexts, it is more desirable to perform data analytics and learning tasks directly on edge devices for crucial benefits such as increased energy efficiency, reduced latency as well as lower communication cost.To address the above challenge, this paper proposes a tiny federated learning algorithm for enabling learning of Bayesian classifiers based on distributed tiny data storage, referred to as TFL-BC. In TFL-BC, Bayesian learning is executed in parallel across multiple edge devices using local (tiny) training data and subsequently the learning results from local devices are aggregated via a central node to obtain the final classification model. The results of experiments conducted on a set of benchmark datasets demonstrate that our algorithm can produce final aggregated models that outperform single tiny Bayesian classifiers and that the result of tiny federated learning (of Bayesian classifier) is independent of the number of data partitions used for generating the distributed local training data.
MoreTranslated text
Key words
tiny machine learning,federated learning,Bayesian classifier,edge computing
AI Read Science
Must-Reading Tree
Example
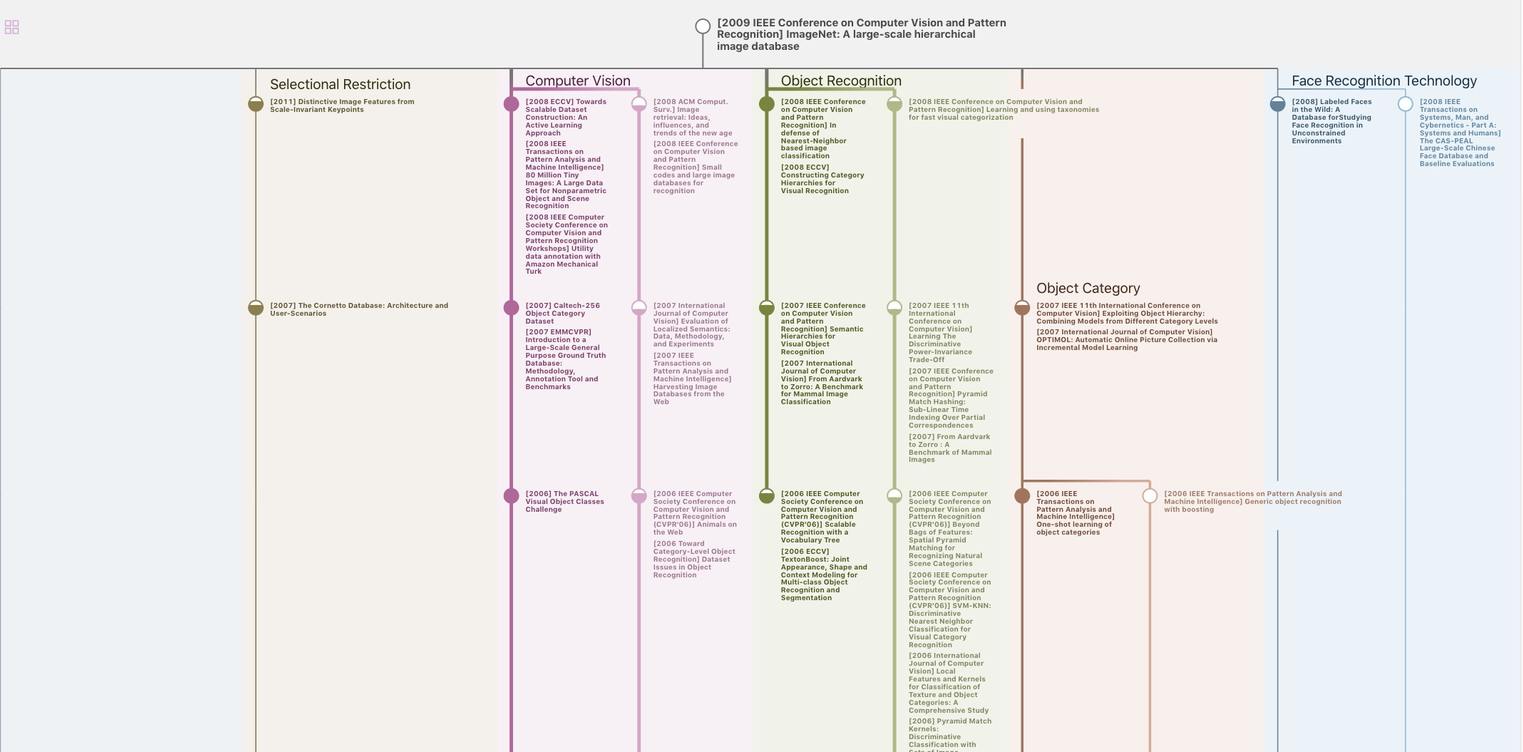
Generate MRT to find the research sequence of this paper
Chat Paper
Summary is being generated by the instructions you defined