Addressing Predicate Overlap in Scene Graph Generation with Semantic Granularity Controller
ICME(2023)
摘要
Semantic overlap between predicates (e.g., riding versus on) occurs inevitably when describing a scene. However, most existing Scene Graph Generation (SGG) works sidestep it by modeling the semantic overlap at category-level and assigning merely one-hot target to each sample, which hurt the performance on other reasonable predicates. In this paper, we argue that semantic overlap between predicates tends to vary in different abstract patterns, and a subject-object pair should retain multiple reasonable predicates. To this end, we make an early attempt to reformulate SGG as a partial multi-label learning problem and accordingly propose a model-agnostic Semantic Granularity Controller (SGC). SGC consists of a pattern-specific controller, partial multi-label learning, and controllable inference. The former two solve semantic confusion during training, while the latter makes the semantic granularity of prediction controllable. Extensive experiments demonstrate that SGC can improve the performance of SGG and guide the model to predict coarse/fine-grained predicates.
更多查看译文
关键词
Scene graph generation, Label confusion, Predicate overlap, Partial multi-label learning
AI 理解论文
溯源树
样例
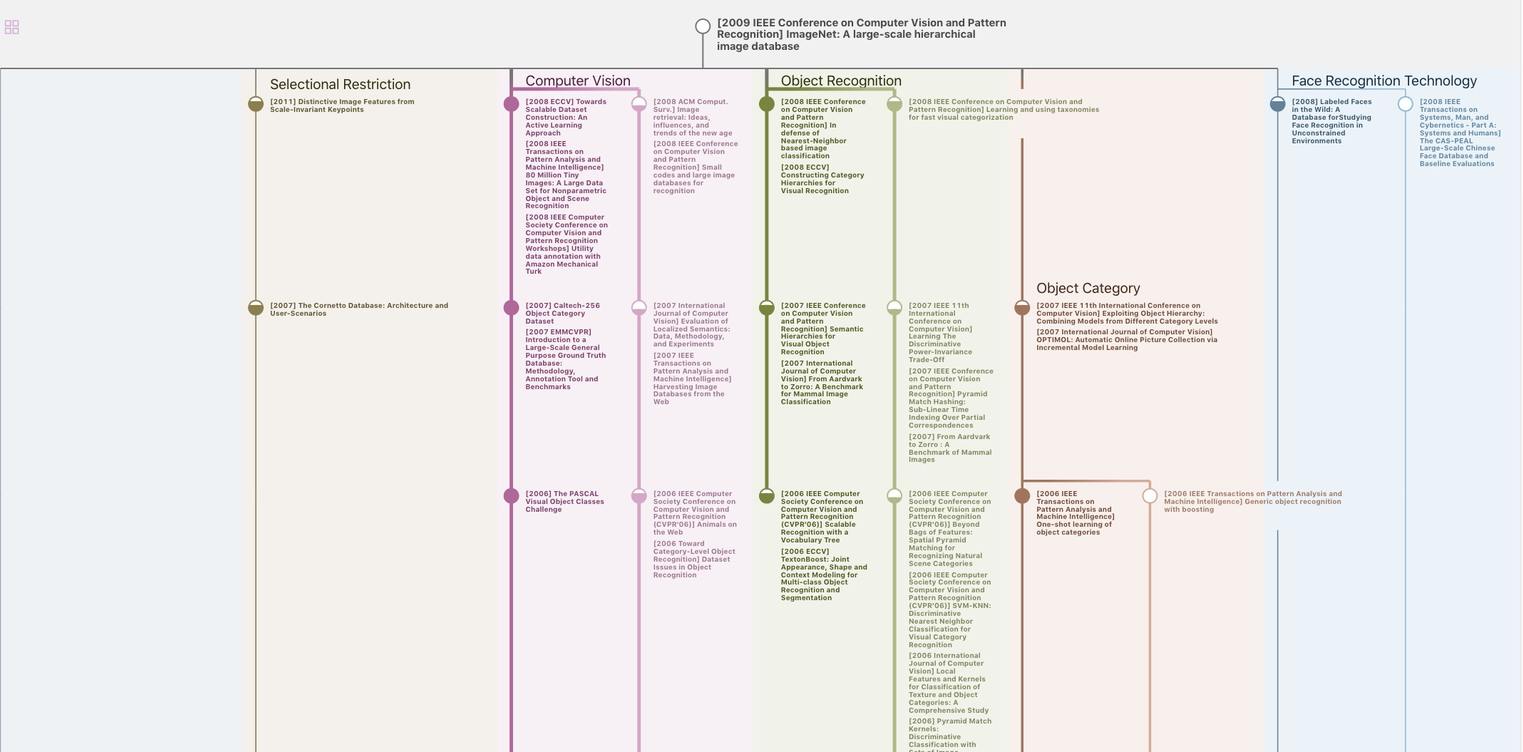
生成溯源树,研究论文发展脉络
Chat Paper
正在生成论文摘要