Improving robustness of learning-based adaptive video streaming in wildly fluctuating networks
ICME(2023)
摘要
With the development of wireless technology, the fifth-generation mobile communication network (5G) can offer ultra-high bandwidth to support high-quality video streaming services. However, such a network shows much wild fluctuation especially in the case of mmWave 5G, making it challenging to ensure a robust video streaming service. In this paper, we propose a robust adaptive bitrate algorithm based on deep reinforcement learning, named MAP-DRL. In "robust", we mean that MAP-DRL provides high quality of experience (QoE) streaming services under various network conditions and different QoE preferences. In particular, to avoid the gradient explosion caused by network fluctuation, we introduce several reinforcement learning designs into MAP-DRL, such as advantage function normalization and reward scaling. To fully utilize the limited environment feedback, we process environmental information via a multi-type data manner and design the corresponding network structures for each type of them. The experimental results on real-world network trace datasets demonstrate that MAP-DRL can succeed in improving the average QoE by 29% versus heterogeneous networks and user preferences as compared with the representative benchmarks.
更多查看译文
关键词
Adaptive Video Streaming,Deep Reinforcement Learning,Wildly Fluctuating Networks
AI 理解论文
溯源树
样例
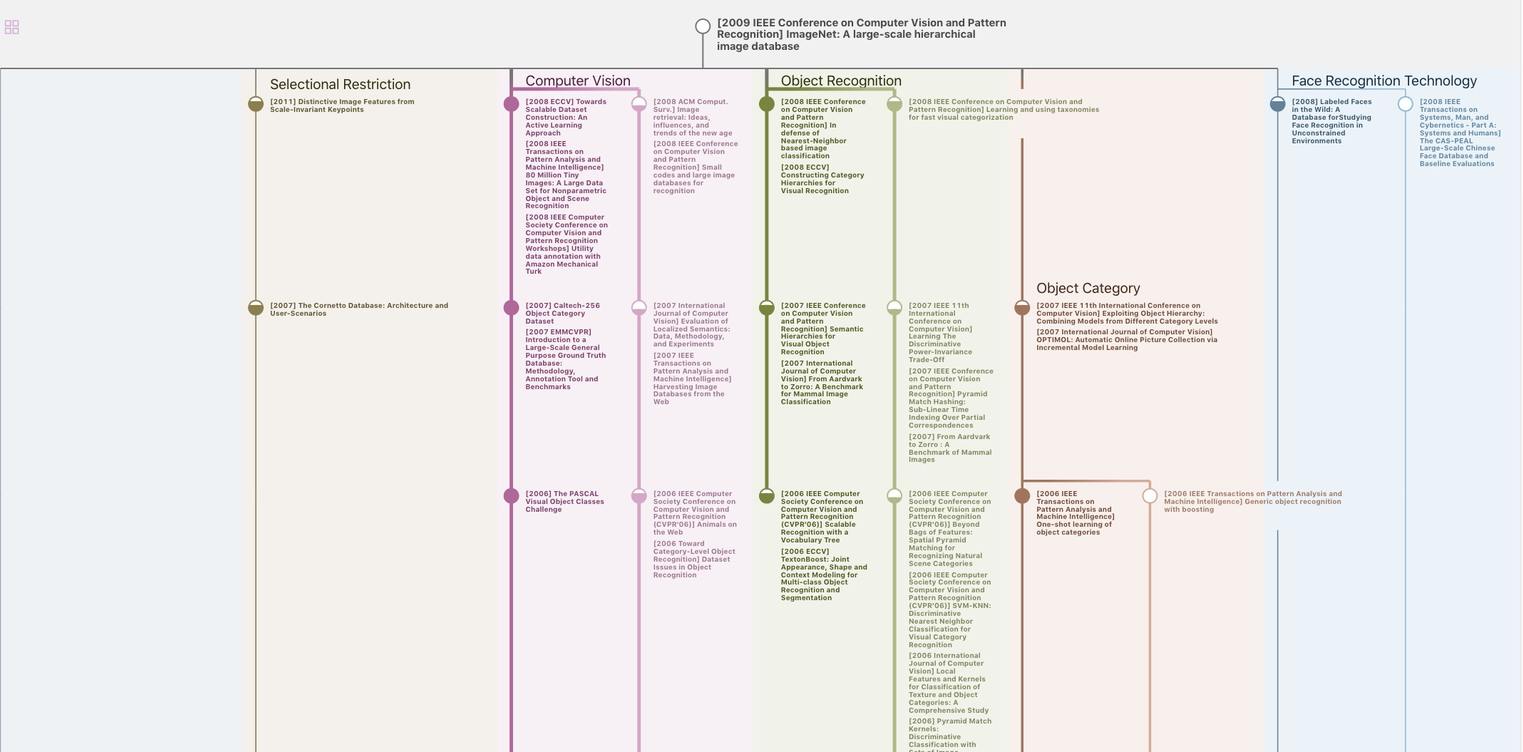
生成溯源树,研究论文发展脉络
Chat Paper
正在生成论文摘要