Compact Intertemporal Coupling Network for Remote Sensing Change Detection.
ICME(2023)
摘要
Change detection of multi-temporal remote sensing images is in full flourishing accompanied by the popularity and prosperity of deep learning. The prominent challenge lies in the crude distribution of the newly constructed and demolished changes, the interferences of massive irrelevant objects, and the spatial-temporal changes from the passage of time. For context modeling, existing solutions waste massive attention on task-irrelevant features, spotlighting insufficiently on the genuinely changed regions. To fill the gap, we propose a compact intertemporal coupling network (CICNet) to derive the change representations. Specifically, to underpin the interaction of spatial-temporal differences in a global perspective, we detach and innovate the solo-head self-attention into a lightweight intertemporal-attention, favorably bridging the intra-level features. In addition, to circumvent the misalignment imposed by spatial sampling, lightweight global channel- and spatial-attentions are globally incorporated for stepwise calibration between localization seduced low-level information and semantics abundant high-level features. Based on a naive backbone (ResNet18/34) without sophisticated structures, our model outperforms other state-of-the-art methods on four datasets in terms of both efficiency and effectiveness.
更多查看译文
关键词
Change detection (CD), remote sensing (RS), intertemporal-attention, multi-level alignment
AI 理解论文
溯源树
样例
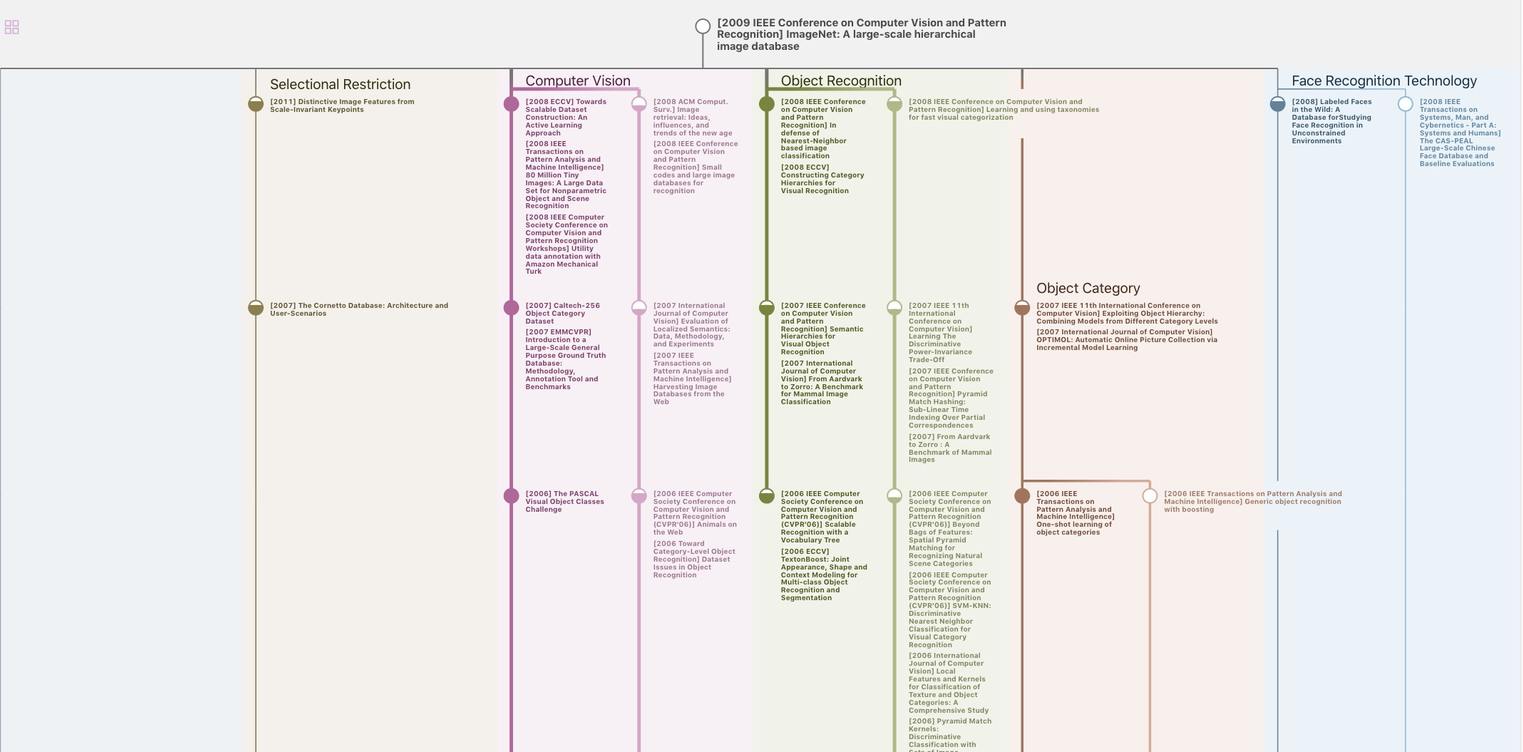
生成溯源树,研究论文发展脉络
Chat Paper
正在生成论文摘要