Frame-Level Embedding Learning for Few-shot Bioacoustic Event Detection
ICME(2023)
摘要
We propose an effective frame-level embedding learning framework for few-shot bioacoustic event detection (FSBED). First, the duration of different animal calls varies greatly, so we innovatively propose a frame-level embedding learning scheme, which can obtain adaptive event receptive fields with more accurate frame-level units. Next, we develop a transfer learning-based approach to deal with the mismatch between training and testing data. Finally, we use the idea of semi-supervised learning to solve the problem of too little labeled data in few-shot learning. By incorporating these several sets of techniques, our overall system ranked first place in the FSBED task of Detection and Classification of Acoustic Scenes and Events (DCASE) Challenge 2022.
更多查看译文
关键词
DCASE, few-shot bioacoustic event detection, frame-level embedding learning, transfer learning
AI 理解论文
溯源树
样例
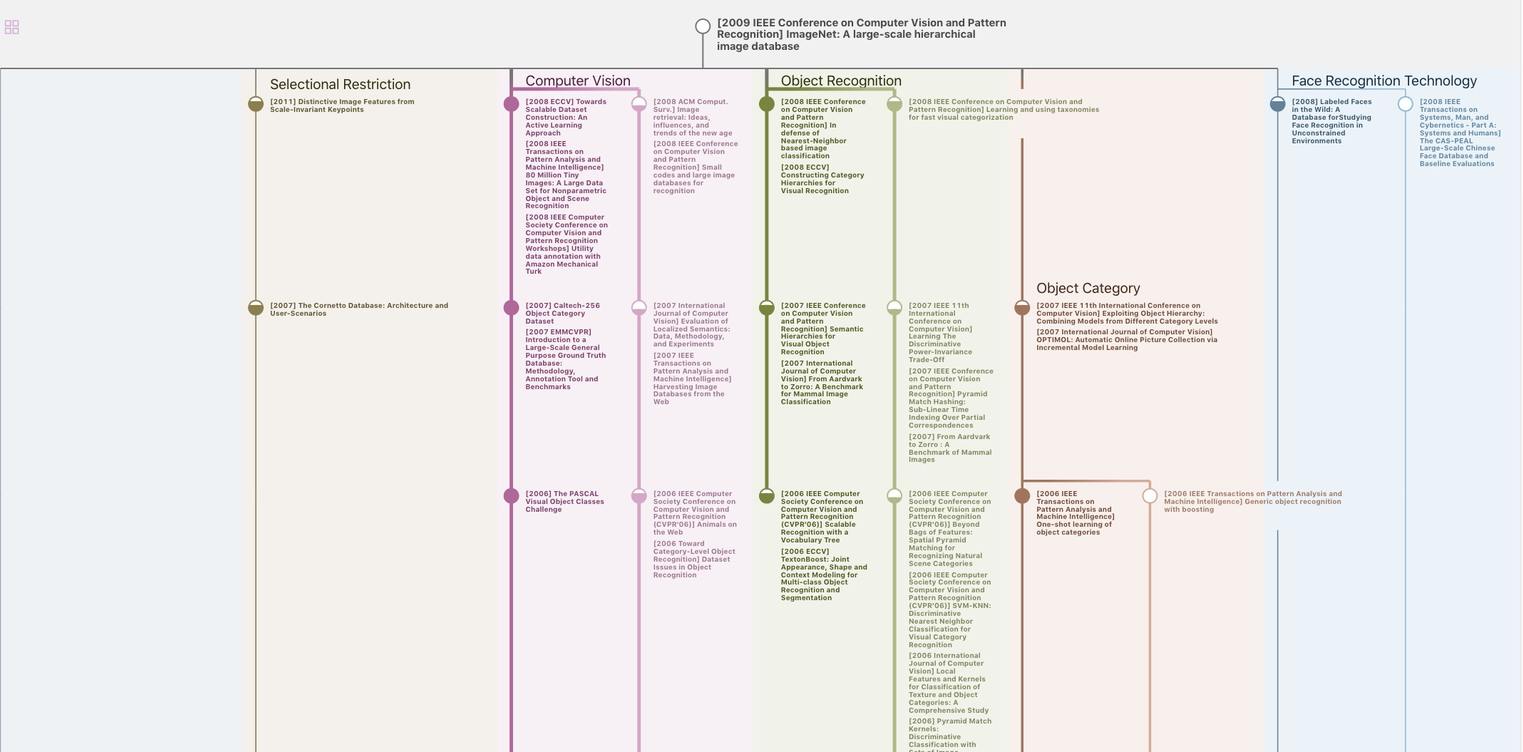
生成溯源树,研究论文发展脉络
Chat Paper
正在生成论文摘要