M-GCN: Multi-scale Graph Convolutional Network for 3D Point Cloud Classification.
ICME(2023)
摘要
Point clouds are among the popular geometric representations for 3D vision applications. 3D point clouds are irregular and flexible, thus processing and summarizing information over these unordered data points are very challenging. Although a number of previous works attempt to analyze point clouds and achieve promising performances, their performances still lack efficient topological information. In this paper, we propose a novel multi-scale graph convolutional network (M-GCN), which is designed to extract local geometric features based on multiscale feature fusion. The extracted local topological information across scales can enrich the representation power of point clouds more effectively. Experiments on ModelNet40 show that local graph neural networks built with various scale point features and edge features can achieve state-of-the-art performance on challenging classification benchmarks of 3D point clouds.
更多查看译文
关键词
Graph Neural Network, Point Clouds, Feature Aggregation, Classification
AI 理解论文
溯源树
样例
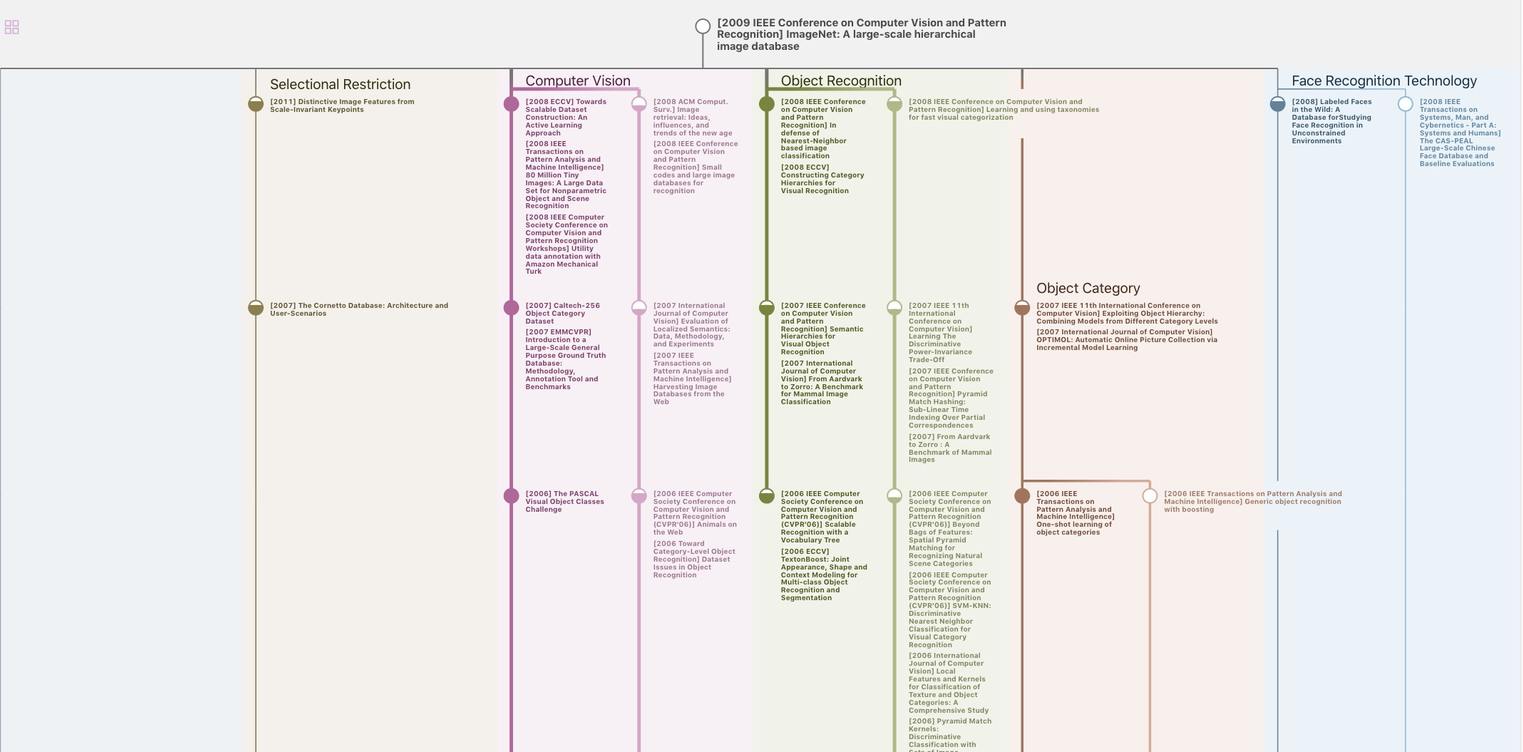
生成溯源树,研究论文发展脉络
Chat Paper
正在生成论文摘要