Feature Sniffer: A Stealthy Inference Attacks Framework on Split Learning
ARTIFICIAL NEURAL NETWORKS AND MACHINE LEARNING, ICANN 2023, PT VII(2023)
摘要
Split learning, a novel privacy-preserving distributed machine learning framework, is proposed to overcome the resource limitations issue of devices in federated learning. Previous studies have explored the possibility of inference attacks on split learning. However, existing methods suffer from unrealistic threat models and poor robustness against defensive techniques. Remarkably, we propose a novel and general framework to perform inference attacks stealthily and reveal the privacy vulnerability of split learning at the convergence stage. In our framework, the malicious server distills knowledge on an auxiliary dataset and transfers the identity information of clients' data to the auxiliary feature space to sniff out the private data. The attack is behind the scenes and hard to detect. Empirically, we consider image classification as the desired task in split learning and evaluate the effectiveness of our method on common image classification datasets. Extensive experiments still obtain SOTA results in the face of strict differential privacy. The code is available at https://github.com/Rostar-github/FSA.
更多查看译文
关键词
Split learning,Neural network models,Transfer learning
AI 理解论文
溯源树
样例
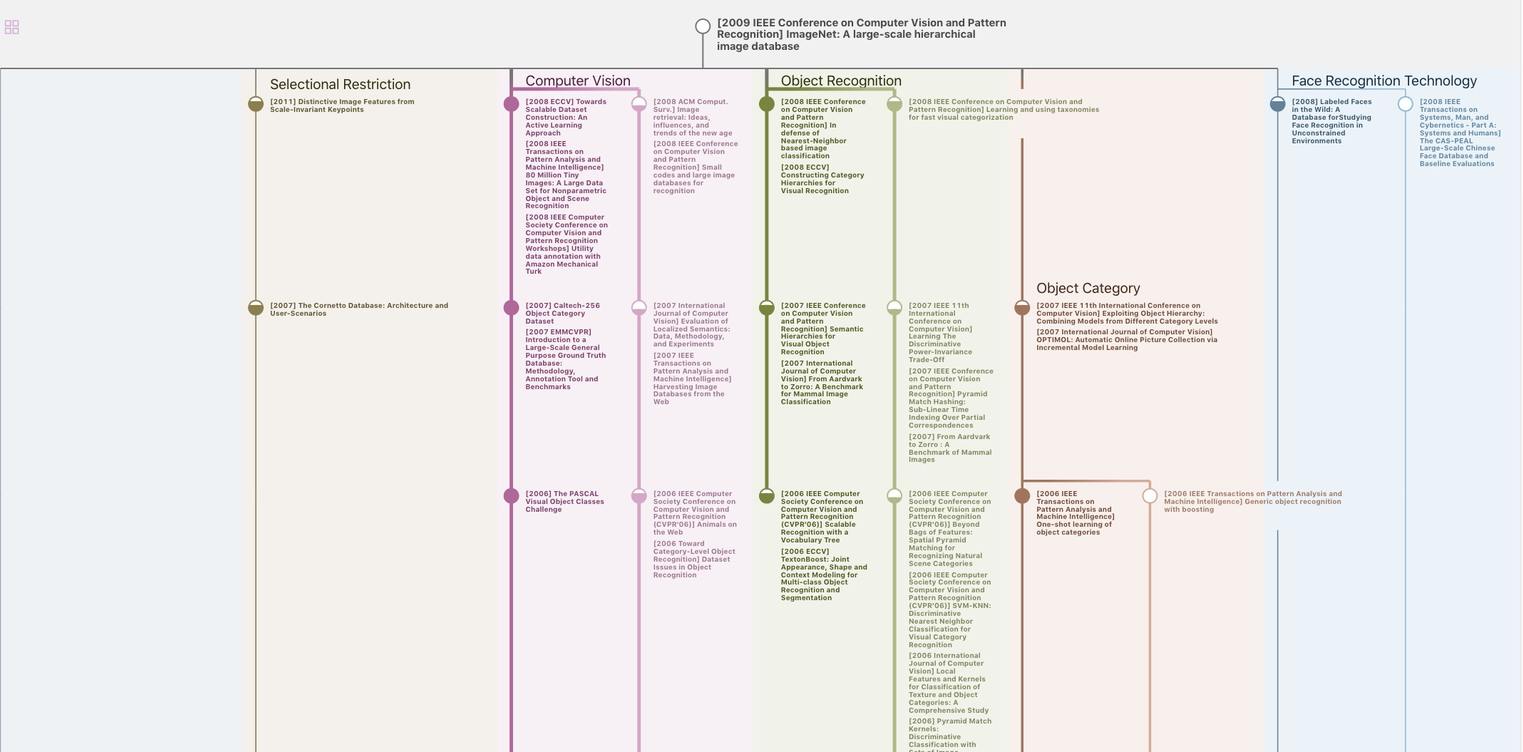
生成溯源树,研究论文发展脉络
Chat Paper
正在生成论文摘要