Gradient Adjusted and Weight Rectified Mean Teacher for Source-Free Object Detection
ARTIFICIAL NEURAL NETWORKS AND MACHINE LEARNING, ICANN 2023, PT VII(2023)
摘要
Source-free object detection (SFOD) aims at adapting object detectors to the unlabeled target domain without access to the labeled source domain. Recent SFOD methods are developed based on the Mean Teacher framework, which consists of a student and a teacher model for self-training. Despite the great success, existing methods suffer from the challenges of missing detections and fitting to incorrect pseudo labels. To overcome these challenges, we propose a Gradient Adjusted and Weight Rectified Mean Teacher framework with two novel training strategies for SFOD, i.e., Negative Gradient Adjustment (NGA) and Source Weight Rectification (SWR). The proposed Negative Gradient Adjustment suppresses the negative gradients caused by missing detections, while the Source Weight Rectification enhances the robustness by rectifying errors of pseudo labels. Additionally, weak-strong consistency data augmentation is introduced for stronger detector performance. Extensive experiments on four benchmarks demonstrate that our proposed method outperforms the existing works for SFOD.
更多查看译文
关键词
Source-free Object Detection,Negative Gradient Adjustment,Source Weight Rectification
AI 理解论文
溯源树
样例
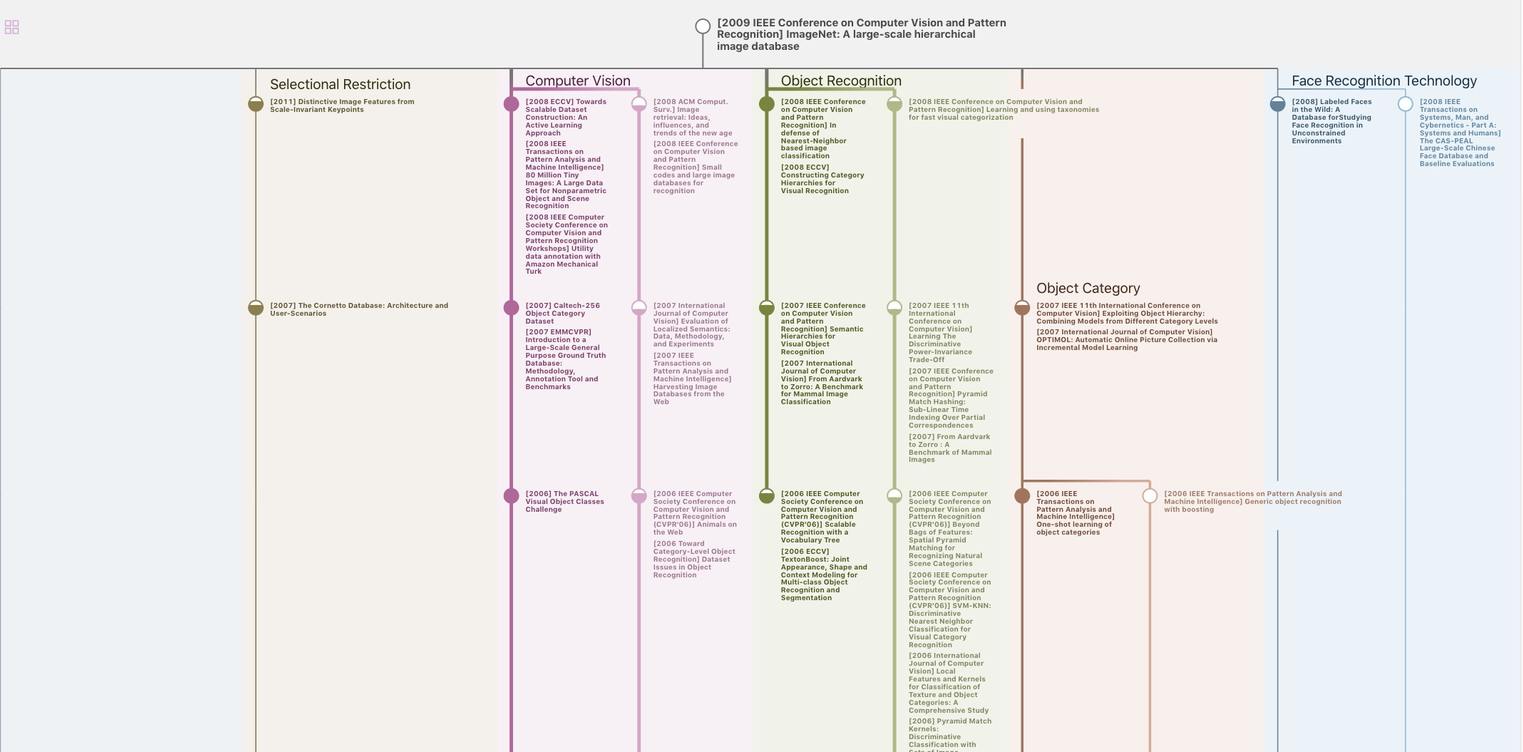
生成溯源树,研究论文发展脉络
Chat Paper
正在生成论文摘要