Multi-task Pre-training for Lhasa-Tibetan Speech Recognition
ARTIFICIAL NEURAL NETWORKS AND MACHINE LEARNING, ICANN 2023, PT IX(2023)
Abstract
Compared to mainstream languages such as Chinese and English, Tibetan speech corpus is limited. Pre-training technology can improve the speech recognition performance for low-resource language by using multiple languages corpus, which involves initially training a neural network on the multi-language dataset, followed by fine-tuning the trained model on low-resource language. In this paper, a multi-task serial pre-training method is proposed to address the limited resources in Tibetan speech recognition. By designing the number and order of tasks in the pre-training process, better recognition performance can be achieved. The experiments on the Lhasa-Tibetan speech recognition task show that our proposed method is significantly superior to the baseline model, achieving a Tibetan word error rate of 4.12%, which is a 9.34% reduction compared to the baseline model and 1.06% lower compared to the existing pre-training model.
MoreTranslated text
Key words
Lhasa-Tibetan speech recognition,Multi-task,Serial Pre-training
AI Read Science
Must-Reading Tree
Example
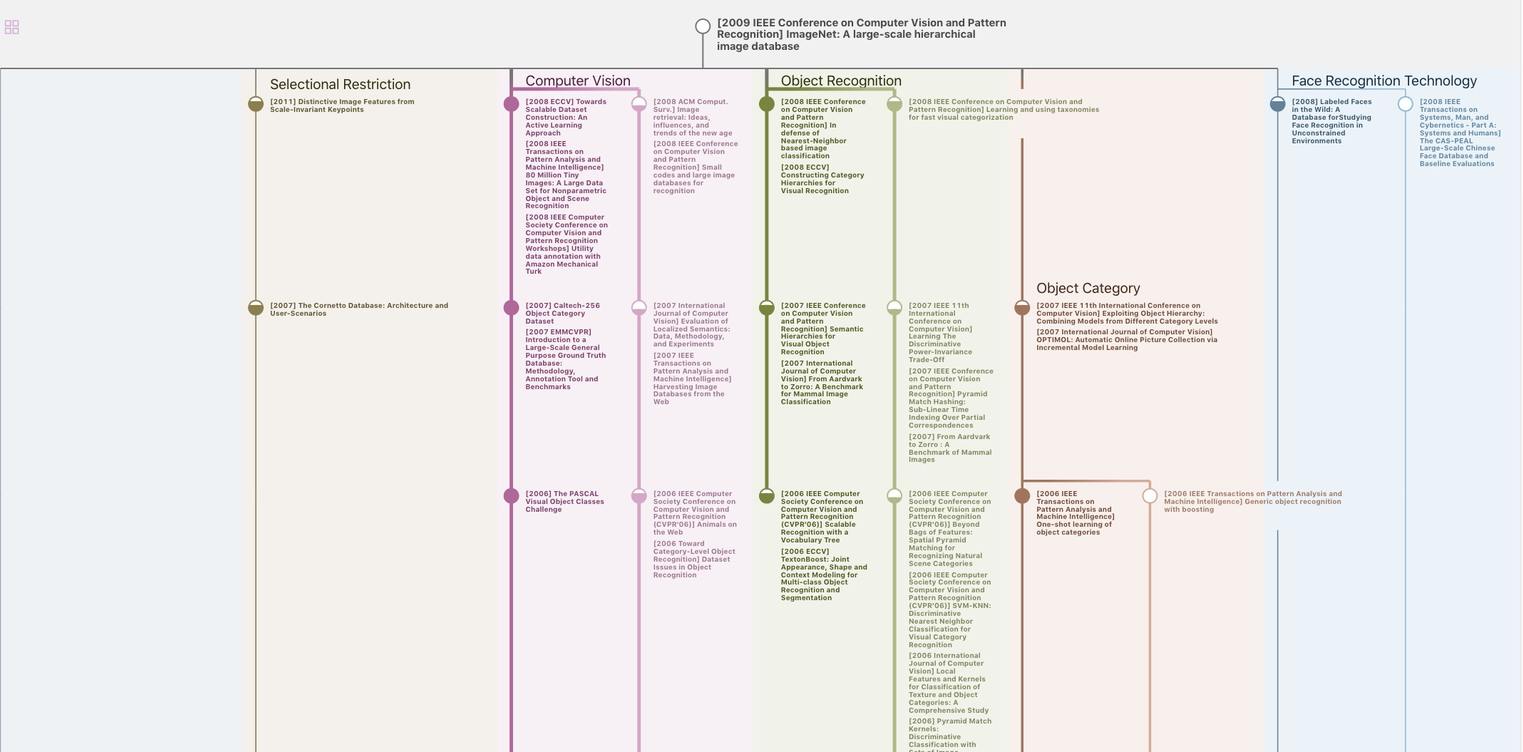
Generate MRT to find the research sequence of this paper
Chat Paper
Summary is being generated by the instructions you defined