Linear Transformer-GAN: A Novel Architecture to Symbolic Music Generation
ARTIFICIAL NEURAL NETWORKS AND MACHINE LEARNING, ICANN 2023, PT VII(2023)
摘要
Long-structured music generation that can be compared to human compositions remains an unresolved area of research. Since their introduction, the Transformer model and its variations, which rely on self-attention, have gained popularity in generating long-structured music. However, these models employ the teacher-forcing approach during training, which causes an exposure bias problem. Consequently, the generative model is incapable of producing music that consistently adheres to music theory. To address this issue, we propose a new Linear Transformer-GAN structure that generates high-quality music using a discriminator that has been trained to detect exposure bias. The Linear Transformer, a new and efficient variation of transformers, is creatively integrated with a generative adversarial network (GAN) to form our proposed model. In order to overcome the limitations of discrete domain data in GAN, we use the Policy Gradient and present a new discriminator structure that evaluates the current sequence reward based on several dimensions of music information. We use both the cross-entropy loss of different information dimensions and a music-theoretic mechanism to train the discriminator. Our experiments demonstrate that the proposed model generates music more consistent with music theory and is perceived as more pleasurable by listeners. This conclusion is supported by objective metrics and human evaluation. Overall, our approach offers a promising solution to the exposure bias problem in long-structured music generation and provides a more effective means of generating music that adheres to established music theory principles.
更多查看译文
关键词
Adversarial learning,Transformer,Music generation
AI 理解论文
溯源树
样例
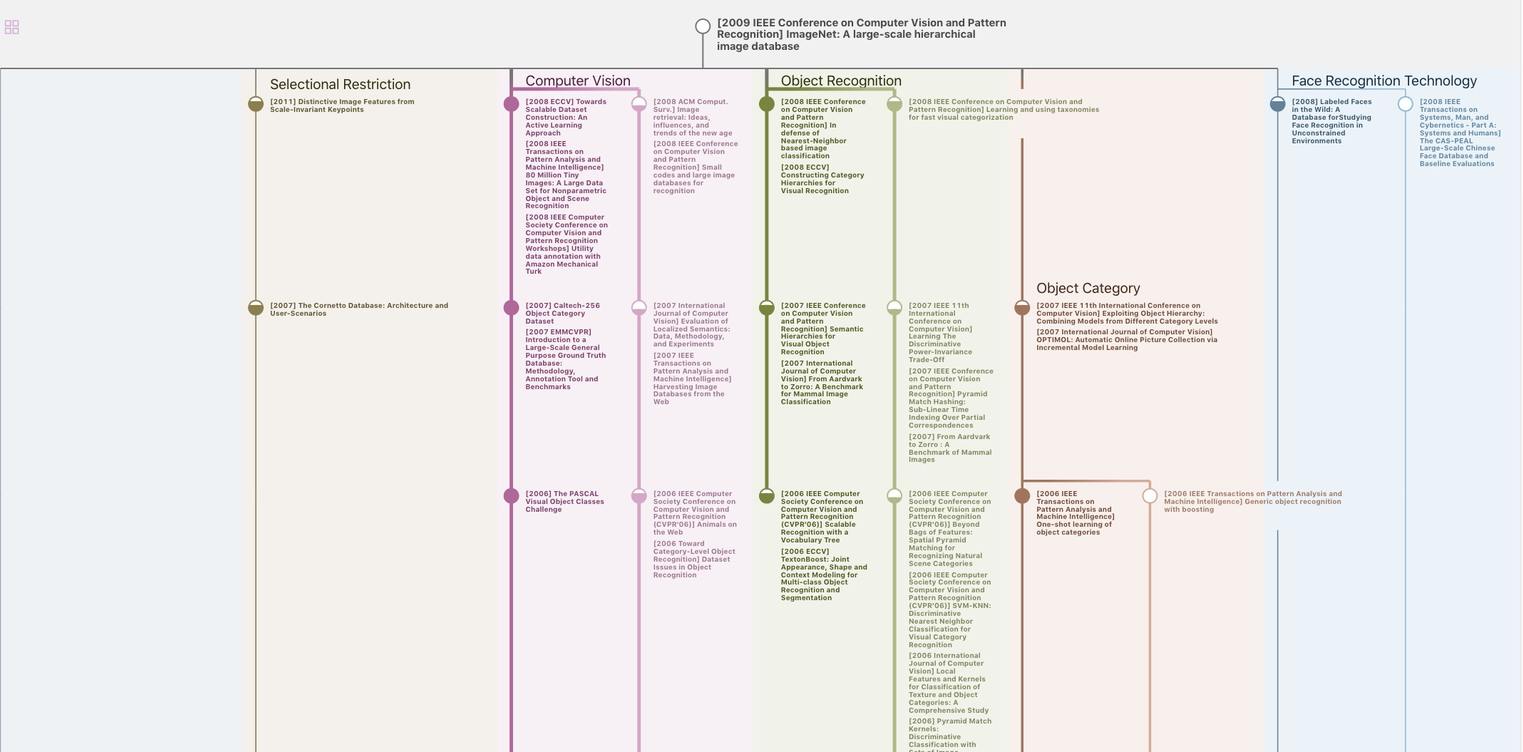
生成溯源树,研究论文发展脉络
Chat Paper
正在生成论文摘要