Vulnerability Analysis of Continuous Prompts for Pre-trained Language Models
ARTIFICIAL NEURAL NETWORKS AND MACHINE LEARNING, ICANN 2023, PT IX(2023)
摘要
Prompt-based learning has recently emerged as a promising approach for handling the increasing complexity of downstream natural language processing (NLP) tasks, achieving state-of-the-art performance without using hundreds of billions of parameters. However, this paper investigates the general vulnerability of continuous prompt-based learning in NLP tasks, and uncovers an important problem: the predictions of continuous prompt-based models can be easily misled by noise perturbations. To address this issue, we propose a learnable attack approach that generates noise perturbations with the goal of minimizing their L-2-norm in order to attack the primitive, harmless successive prompts in a way that researchers may not be aware of. Our approach introduces a new loss function that generates small and impactful perturbations for each different continuous prompt. Even more, our approach shows that learnable attack perturbations with an L-2-norm close to zero can severely degrade the performance of continuous prompt-based models on downstream tasks. We evaluate the performance of our learnable attack approach against two continuous prompt-based models on three benchmark datasets and the results demonstrate that the noise and learnable attack methods can effectively attack continuous prompts, with some tasks exhibiting an F1-score close to 0.
更多查看译文
关键词
Prompt-based Learning,Adversarial Attack,Pretrained Language Models
AI 理解论文
溯源树
样例
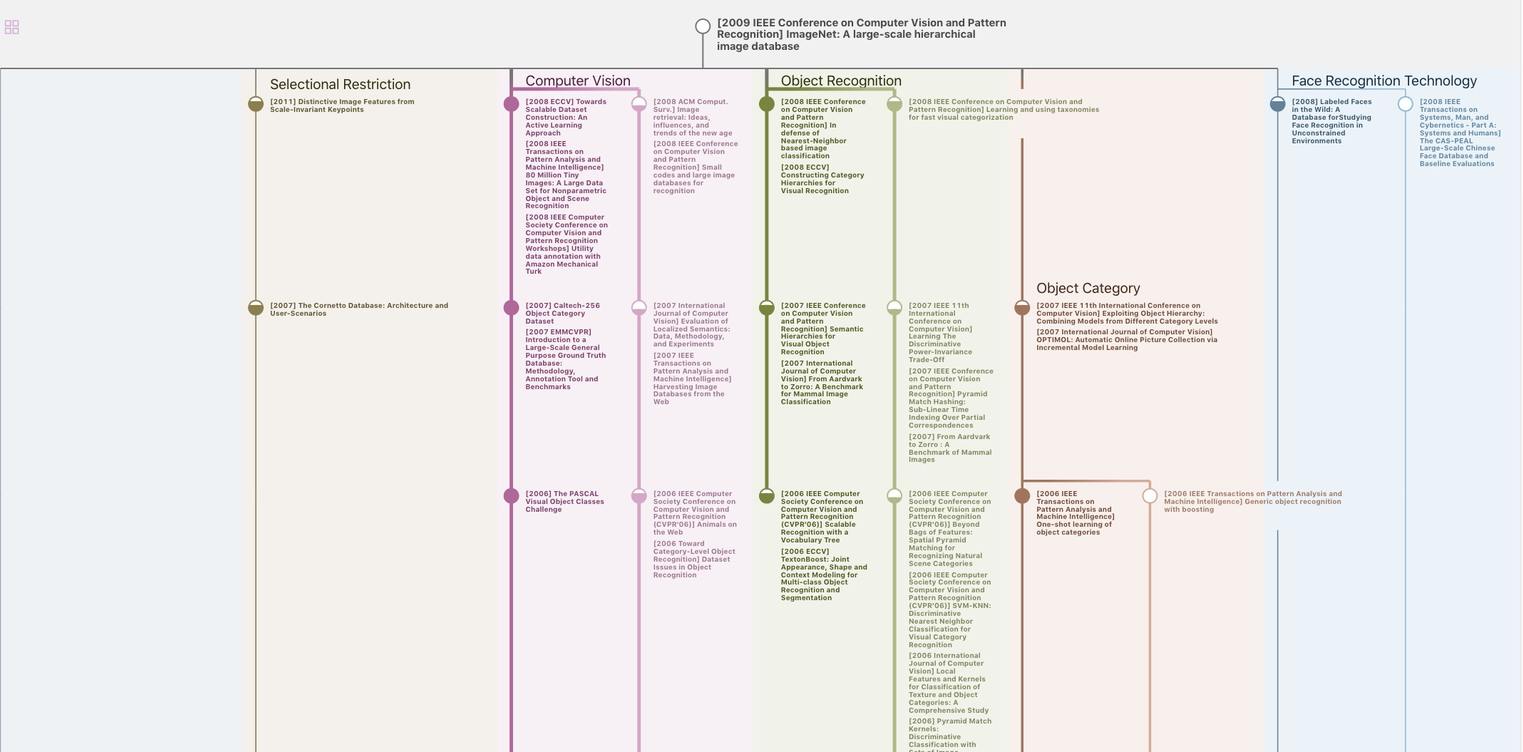
生成溯源树,研究论文发展脉络
Chat Paper
正在生成论文摘要