Model Abstraction and Conditional Sampling with Score-Based Diffusion Models
QUANTITATIVE EVALUATION OF SYSTEMS, QEST 2023(2023)
摘要
We propose an approach to build and sample surrogate stochastic models leveraging state-of-the-art score-based diffusion approaches, either abstracting a known stochastic process or learning directly the model from data. In particular, we propose a method for efficient conditional sampling from such surrogate models, enforcing logical and consistency constraints on generated samples in a soft fashion. As a preliminary case study, we consider a surrogate SIR model, in both its ergodic and non-ergodic formulations. Using the aforementioned method, we are able to sample trajectories from such models that exhibit desirable features having low probability in the unconstrained models, allowing us to explore epidemiologically relevant scenarios. Although the proposed approach is still a work-in-progress, it has significant potential for applications in epidemiology and other fields. The method is also efficient in the sense that retraining is not needed to generate samples satisfying different constraints.
更多查看译文
关键词
Model abstraction,Score-based diffusion model,Conditional generative model
AI 理解论文
溯源树
样例
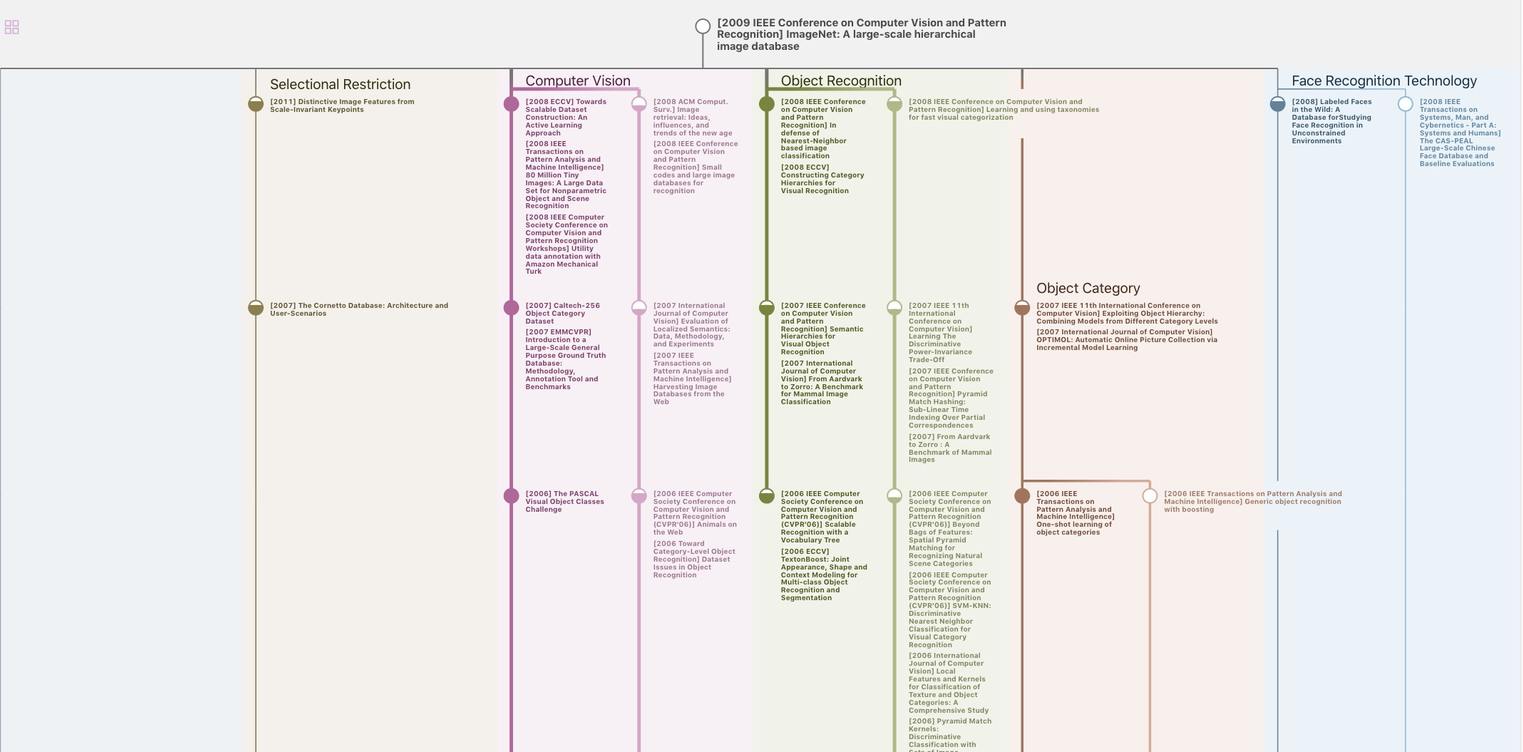
生成溯源树,研究论文发展脉络
Chat Paper
正在生成论文摘要