Asynchronous Execution of Heterogeneous Tasks in ML-Driven HPC Workflows
JOB SCHEDULING STRATEGIES FOR PARALLEL PROCESSING, JSSPP 2023(2023)
摘要
Heterogeneous scientific workflows consist of numerous types of tasks that require execution on heterogeneous resources. Asynchronous execution of those tasks is crucial to improve resource utilization, task throughput and reduce workflows' makespan. Therefore, middleware capable of scheduling and executing different task types across heterogeneous resources must enable asynchronous execution of tasks. In this paper, we investigate the requirements and properties of the asynchronous task execution of machine learning (ML)-driven high-performance computing (HPC) workflows. We model the degree of asynchronicity permitted for arbitrary workflows and propose key metrics that can be used to determine qualitative benefits when employing asynchronous execution. Our experiments represent relevant scientific drivers, we perform them at scale on Summit, and we show that the performance enhancements due to asynchronous execution are consistent with our model.
更多查看译文
关键词
hpc,workflow,adaptability,machine learning,artificial intelligence
AI 理解论文
溯源树
样例
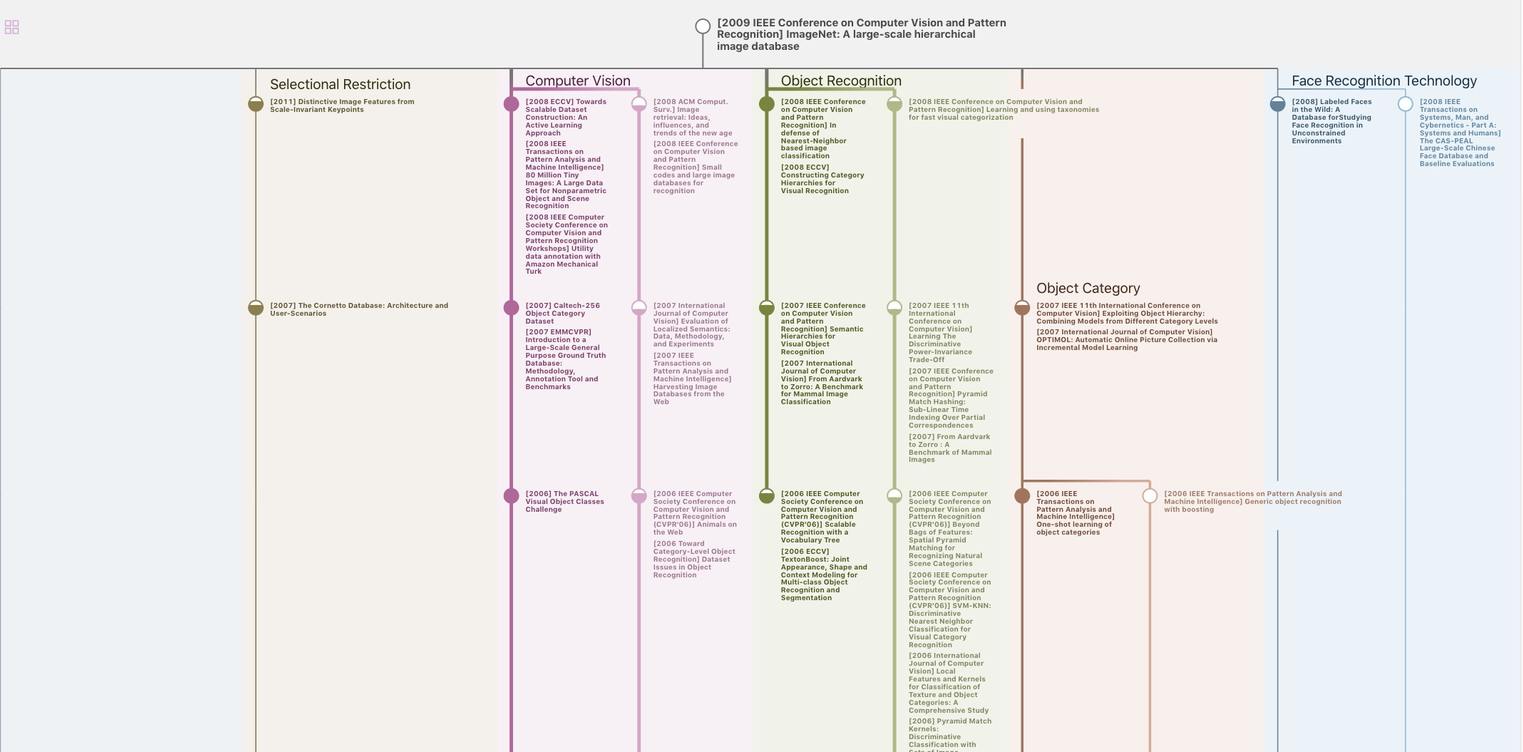
生成溯源树,研究论文发展脉络
Chat Paper
正在生成论文摘要