Using Machine Learning to Recommend Personalized Modular Treatments for Common Mental Health Disorders.
ICDH(2023)
摘要
So far, initial treatment recommendations for internet-based cognitive behavioral therapy (iCBT) decision support were mostly high-level or static. Personalized treatment recommendations could pave the way toward better treatment outcomes and adaptive treatments by leveraging information from past patients. We explore the disadvantages of multi-class recommendation and propose a modular approach using multi-label classification for treatment recommendations. Our machine learning-based treatment recommender composes treatment programs from a set of modules. It achieves a 79.02% F1-score on historically successful treatments, significantly outperforming the existing system by around 4% while offering other advantages such as interpretability and robustness. Using our recommendation as an initial starting point, clinicians can adjust the modular treatments to provide a more personalized treatment.
更多查看译文
关键词
Personalized treatment,Machine learning,Treatment recommendation,Internet-based cognitive behavioral therapy,Modular treatments,Common mental health disorders
AI 理解论文
溯源树
样例
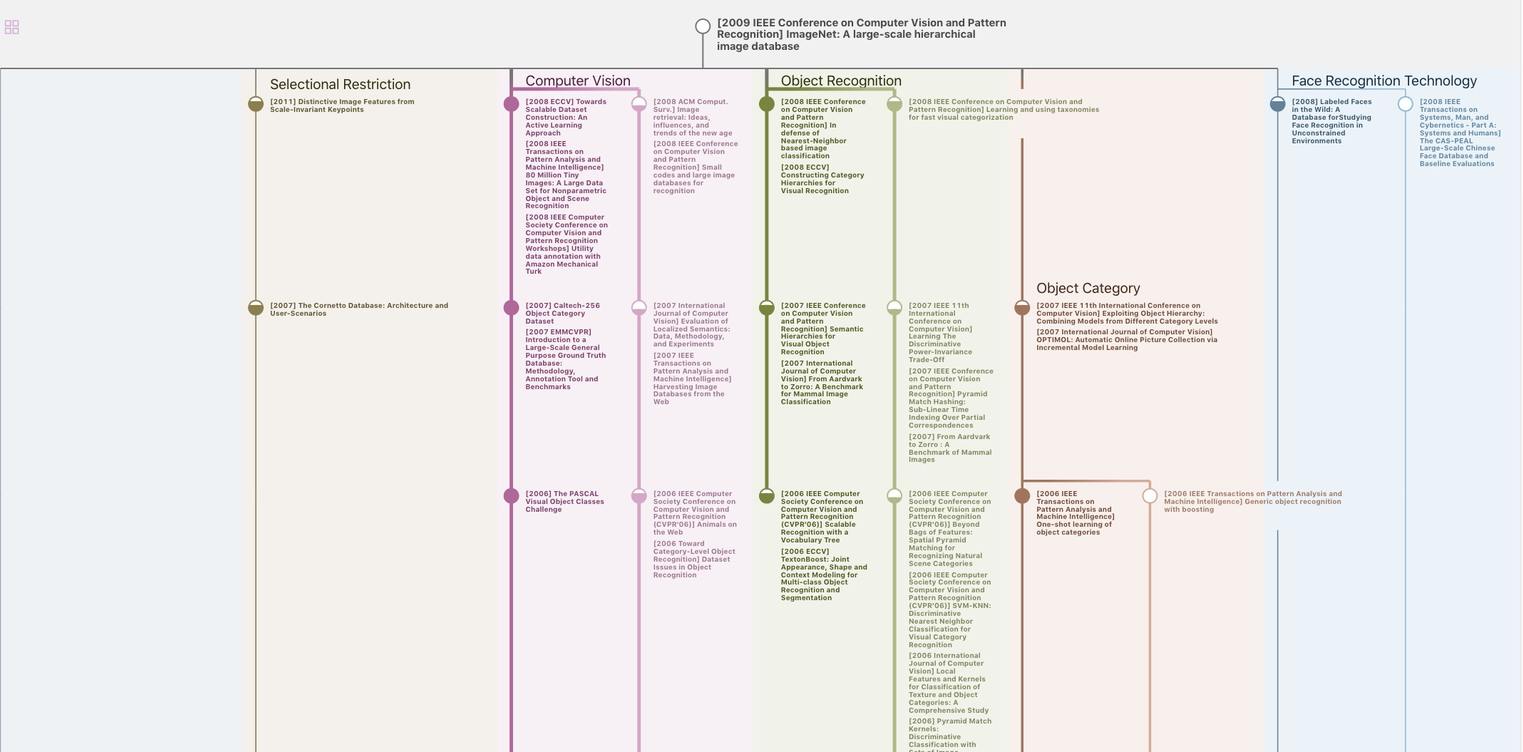
生成溯源树,研究论文发展脉络
Chat Paper
正在生成论文摘要