Mining Sequential Patterns with Timelines from Digital Health Data.
ICDH(2023)
摘要
Descriptive pattern mining is a useful tool in expansion of knowledge. One such area of descriptive pattern mining is that of sequential pattern mining. In sequential mining, items maintain an order of occurrence. In this paper, we present a digital health solution for mining sequential patterns from real-life healthcare data. Specifically, it is a non-trivial extension to the sequential mining algorithm PrefixSpan. Through an association of time, we find improved relevance of a pattern overall significance relative to a focal point. This is particularly useful in the medical domain, where significance of information varies depending on the time of its occurrence. For example, consider a time of being diagnosed with a disease. A condition occurring 16 years prior to the time of diagnosis provides less information than the same condition occurring 2 years prior to diagnosis. In traditional sequential mining, both conditions would equally contribute to support, despite their unequal value in describing causes of diagnosis. To resolve such issue, we provide an inclusion of two additional user-defined parameters to incorporate time within itemsets-namely, a timeline interval (describing the length of an interval, of which itemsets of different intervals are treated separately by their difference in time to a focal point), as well as a maximal window (denoting the maximal interval that disallows for any greater time difference than such interval). With timelines associated to itemsets, relevance of itemsets have improved interpretability for domain experts.
更多查看译文
关键词
services, digital health, data science, data mining, sequences, timelines, patterns, sequential patterns, PrefixSpan, focal point, descriptive data mining, medical data
AI 理解论文
溯源树
样例
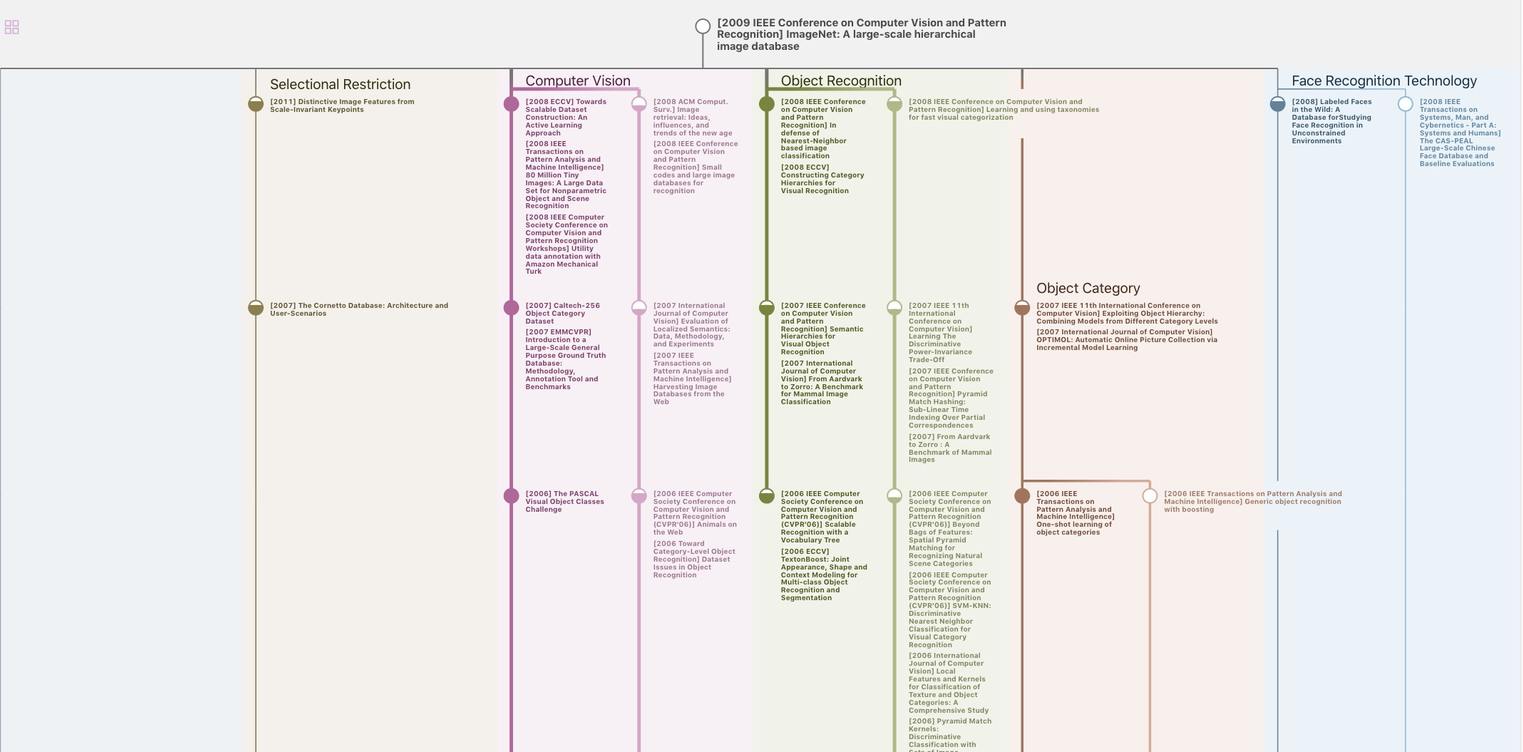
生成溯源树,研究论文发展脉络
Chat Paper
正在生成论文摘要