Few-Shot Information Operation Detection Using Active Learning Approach.
SBP-BRiMS(2023)
Abstract
Previous research suggested that supervised machine learning can be utilized to detect information operations (IO) on social media. Most of the related research assumes that the new data will always be available in the exact timing that models set to be updated. In practice, however, the detection and attribution of IO accounts is time-consuming. There is thus a mismatch between the performance assessment procedures in existing work and the real-world problem they seek to solve. We bridge this gap by demonstrating how active learning approaches can extend the application of classifiers by reducing their dependence on new data. We evaluate the performance of an existing classifier when it gets updated according to five active learning strategies. Using state-sponsored information operation Twitter data, the results show that if querying from Twitter is possible, the best active learning strategy requires 5–10 times less tweets than the original model while only showing 1–3% reduction in the average monthly F1 scores across countries and prediction tasks. If querying from Twitter is not possible, the corresponding active learning strategy requires 5–10 times less tweets while showing 1–9% reduction in the average monthly F1 scores. Depending on the country, a hand-full to few hundred new ground-truth examples would suffice to achieve a reasonable performance.
MoreTranslated text
Key words
detection,learning,few-shot
AI Read Science
Must-Reading Tree
Example
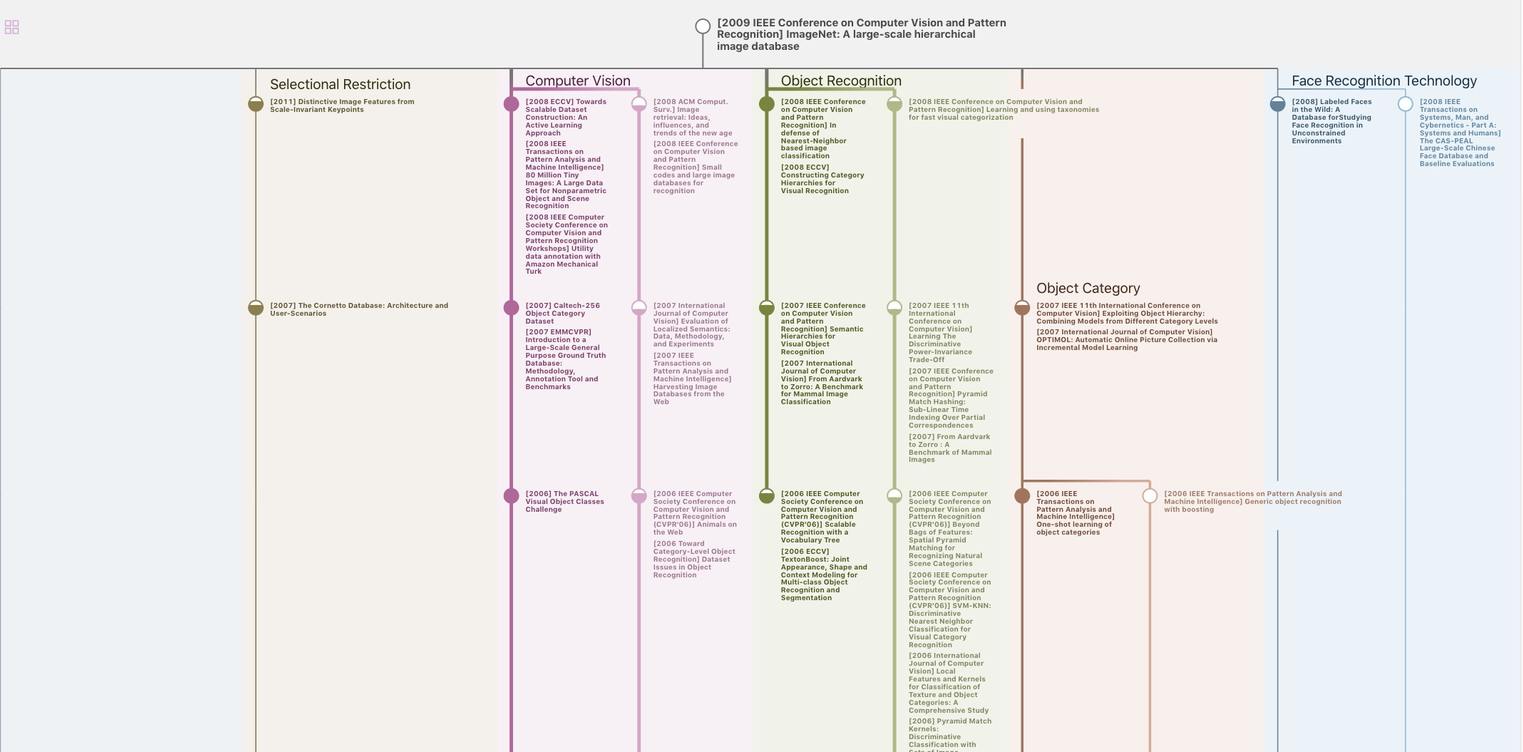
Generate MRT to find the research sequence of this paper
Chat Paper
Summary is being generated by the instructions you defined