An Experimental Study of the Sample Complexity of Domain Adaptation
SIU(2023)
摘要
In this study, we experimentally investigate the sample complexity of semi supervised domain adaptation with deep neural networks. Sweeping the hyper-parameters of domain adaptation neural networks relying on the MMD distance measure and the number of training samples in a controlled manner, we study the test accuracy of the target samples. Both labeled and unlabeled samples from the source and the target domains are used as inputs to the neural network in the experiments. Our experimental findings suggest that the minimum number of samples required for guaranteeing a fixed experimental target accuracy level increases quadratically with both the number and the dimension of the MMD layers in the network used for aligning the source and the target domains. We observe that this relationship is in harmony with some well-known theoretical bounds in the classical deep learning literature. Meanwhile, we also investigate the optimal weighting strategy between the classification loss functions of the source and the target samples, concluding that increasing the weight of the target loss improves the performance as the number of target samples increases.
更多查看译文
关键词
Domain adaptation,deep neural networks,sample complexity,MMD distance,target accuracy
AI 理解论文
溯源树
样例
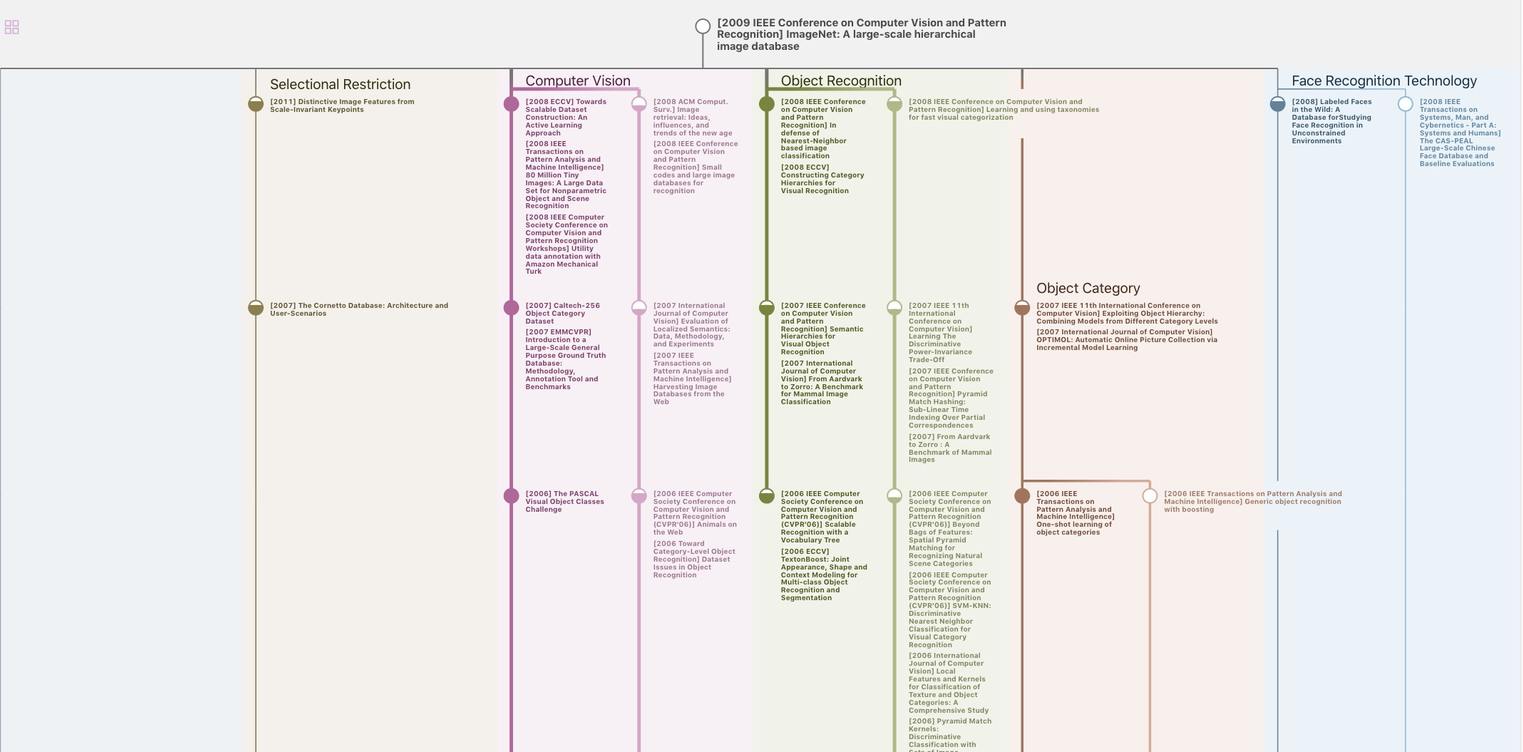
生成溯源树,研究论文发展脉络
Chat Paper
正在生成论文摘要