The Impact of State Merging on Predictive Accuracy in Probabilistic Tree Automata: Dietze's Conjecture Revisited
FUNDAMENTALS OF COMPUTATION THEORY, FCT 2023(2023)
摘要
Dietze's conjecture concerns the problem of equipping a tree automaton M with weights to make it probabilistic, in such a way that the resulting automaton N predicts a given corpus C as accurately as possible. The conjecture states that the accuracy cannot increase if the states in M are merged with respect to an equivalence relation similar to so that the result is a smaller automaton M-similar to. Put differently, merging states can never improve predictions. This is under the assumption that both M and M similar to are bottom-up deterministic and accept every tree in C. We prove that the conjecture holds, using a construction that turns any probabilistic version N-similar to of M-similar to into a probabilistic version N of M, such that N assigns at least as great a weight to each tree in C as N-similar to does.
更多查看译文
关键词
Tree automata,Statistical ML,Probability distributions
AI 理解论文
溯源树
样例
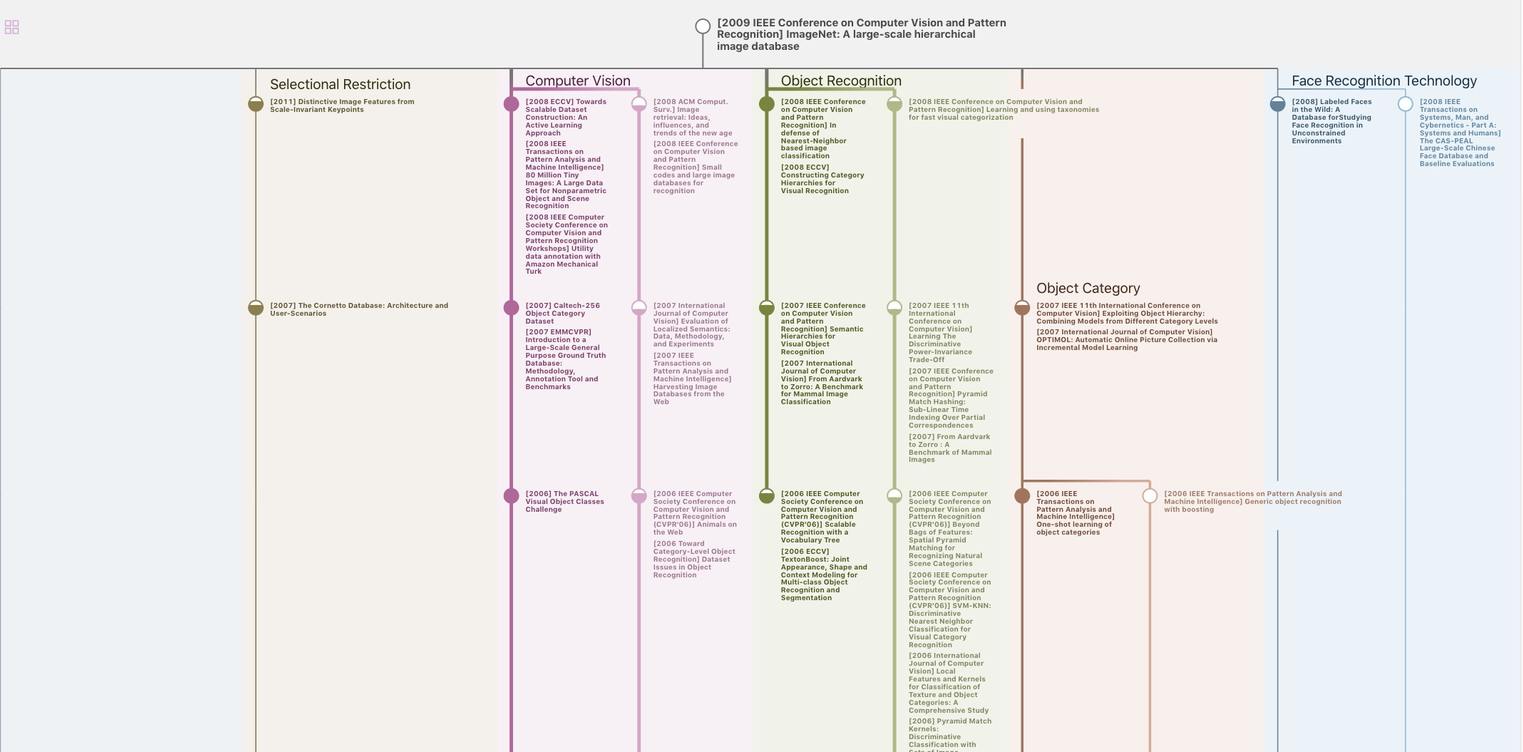
生成溯源树,研究论文发展脉络
Chat Paper
正在生成论文摘要