An Effective Resource Deployment Scheme for IoTs Devices with Federated Learning.
ICCC(2023)
摘要
With the significant improvement of the computing capability of Internet of Things (IoTs) devices, it has become possible for IoT devices to use federated learning to improve the system performance, especially for Industrial IoT. In this paper, we study the resource deployment issues of IoT devices with federated learning, considering the precision, the deployment cost, the energy cost and the performance loss by delay for system model training. Based on precision models developed from existing research, we intend to make a good trade-off between the system precision and the deployment cost. By analyzing the total cost of the deployed IoT system, we prove that the resource deployment problem is convex with respect to the deployed resources, local iteration counts and the scale of collected data. Then, we propose an effective resource deployment scheme, which can minimize the system cost with a precision guarantee. Simulation results show that the proposed scheme can effectively reduce the total deployment cost of the IoT system while guaranteeing the system precision.
更多查看译文
关键词
Federated learning,IoT,Resource deployment optimization
AI 理解论文
溯源树
样例
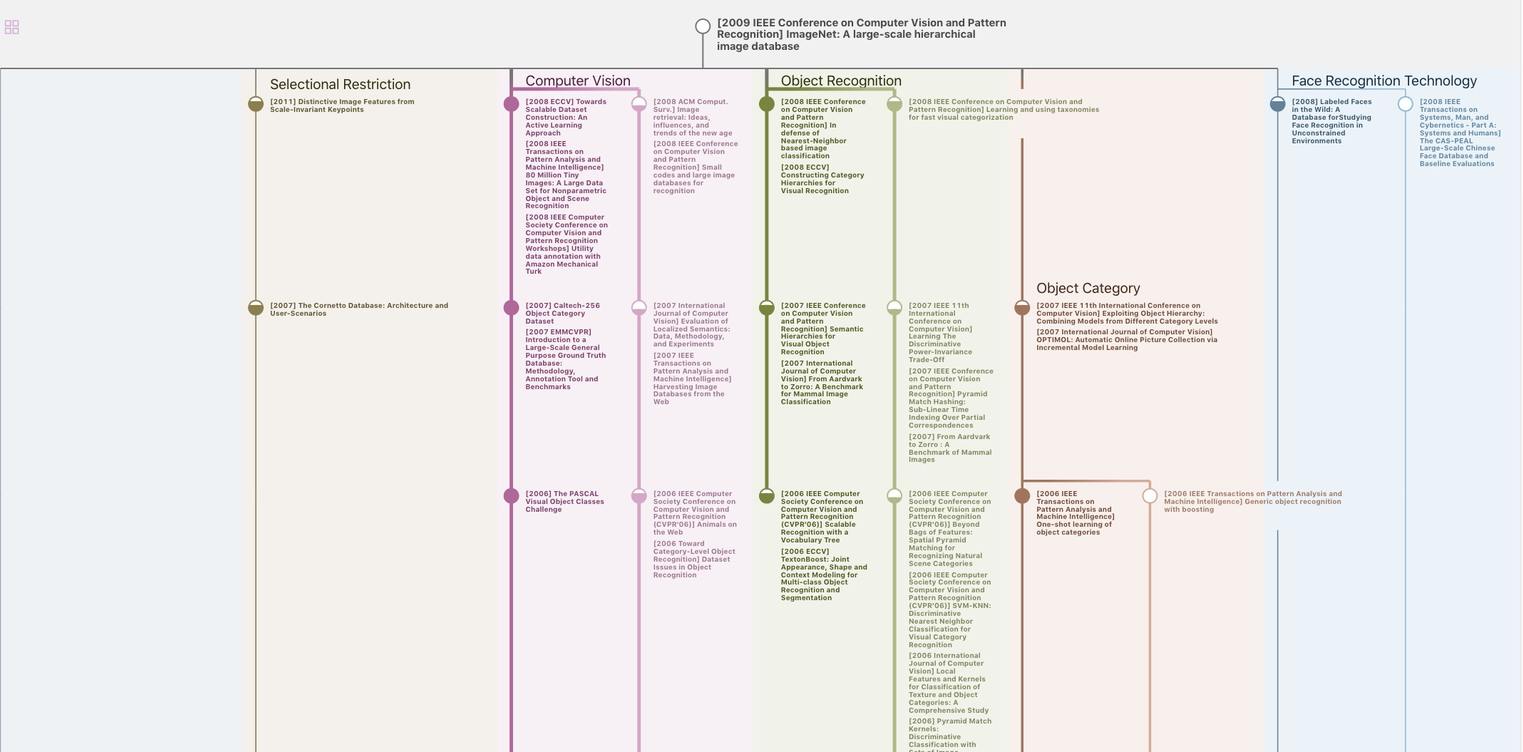
生成溯源树,研究论文发展脉络
Chat Paper
正在生成论文摘要