Throughput Enhancement in Hybrid Vehicular Networks Using Deep Reinforcement Learning.
ISCC(2023)
摘要
Cooperative intelligent transportation systems are now being widely investigated along with the emergence of vehicular communication. Services such as collective perception require a robust communication system with high throughput and reliability. However, a single communication technology is unlikely to support the required throughput, especially under mobility and coverage constraints. Thus, we propose in this paper a hybrid vehicular communication architecture that leverages multiple Radio Access Technologies (RATs) to enhance the communication throughput. We developed a Deep Reinforcement Learning (DRL) algorithm to select the optimal hybrid transmission strategy according to the channel quality parameters. We assess the effectiveness of our hybrid transmission strategy by a simulation scenario that shows about 20% throughput enhancement and a 10% reduction of channel busy ratio.
更多查看译文
关键词
Hybrid vehicular network,ITS-G5,C-V2X,RAT selection,Reinforcement learning,Throughput
AI 理解论文
溯源树
样例
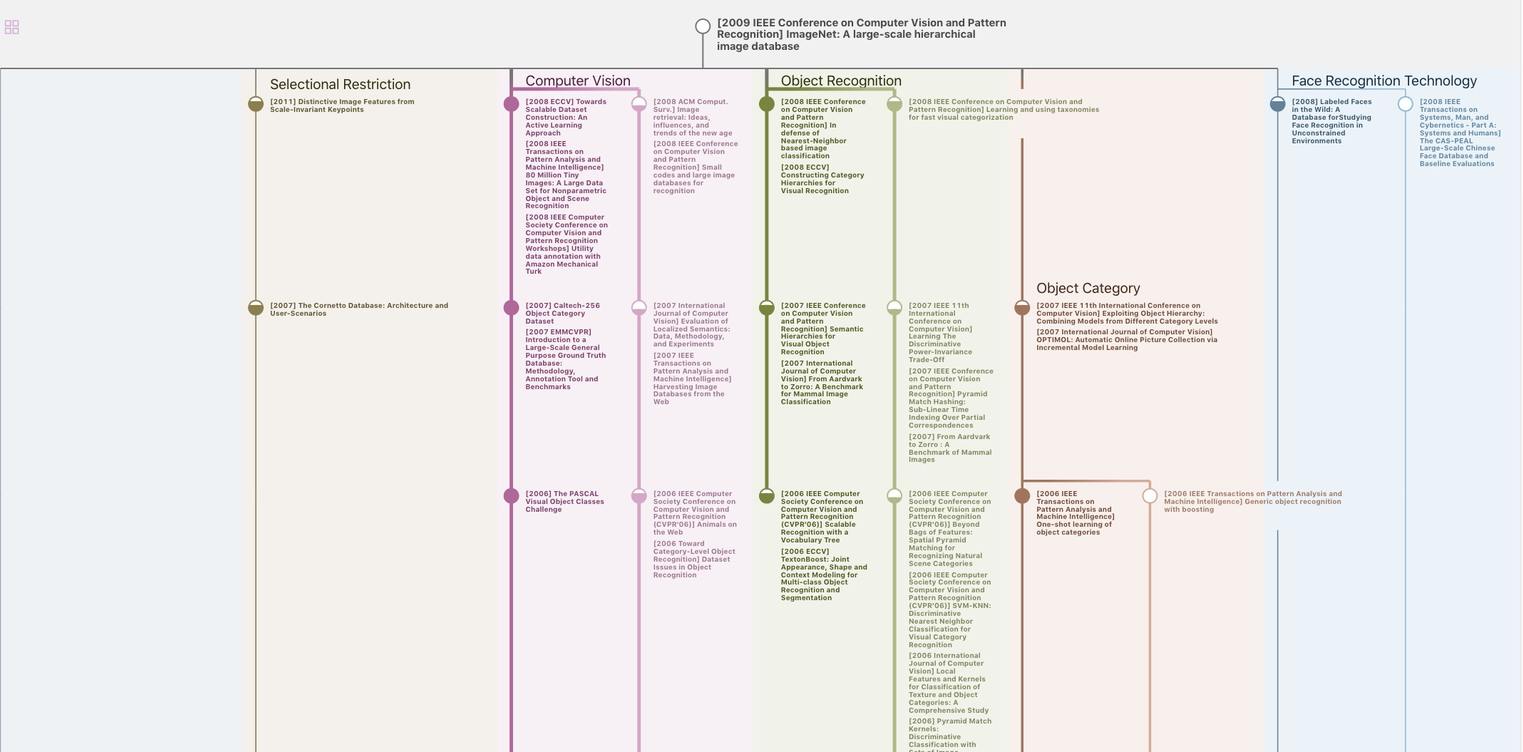
生成溯源树,研究论文发展脉络
Chat Paper
正在生成论文摘要