Balancing Robustness and Covertness in NLP Model Watermarking: A Multi-Task Learning Approach.
ISCC(2023)
摘要
The popularity of ChatGPT demonstrates the immense commercial value of natural language processing (NLP) technology. However, NLP models are vulnerable to piracy and redistribution, which harms the economic interests of model owners. Existing NLP model watermarking schemes struggle to balance robustness and covertness. Robust watermarking require embedding more information, which compromises their covertness; conversely, covert watermarking are challenging to embed more information, which affects their robustness. This paper proposes an NLP model watermarking framework that uses multi-task learning to address the conflict between robustness and covertness in existing schemes. Specifically, a covert trigger set is established to implement remote verification of the watermark model, and a covert auxiliary network is designed to enhance the watermark model's robustness. The proposed watermarking framework is evaluated on two benchmark datasets and three mainstream NLP models. The experiments validate the frame-work's excellent covertness, robustness, and low false positive rate.
更多查看译文
关键词
Natural language processing,NLP model security,Black-box watermarking,White-box watermarking
AI 理解论文
溯源树
样例
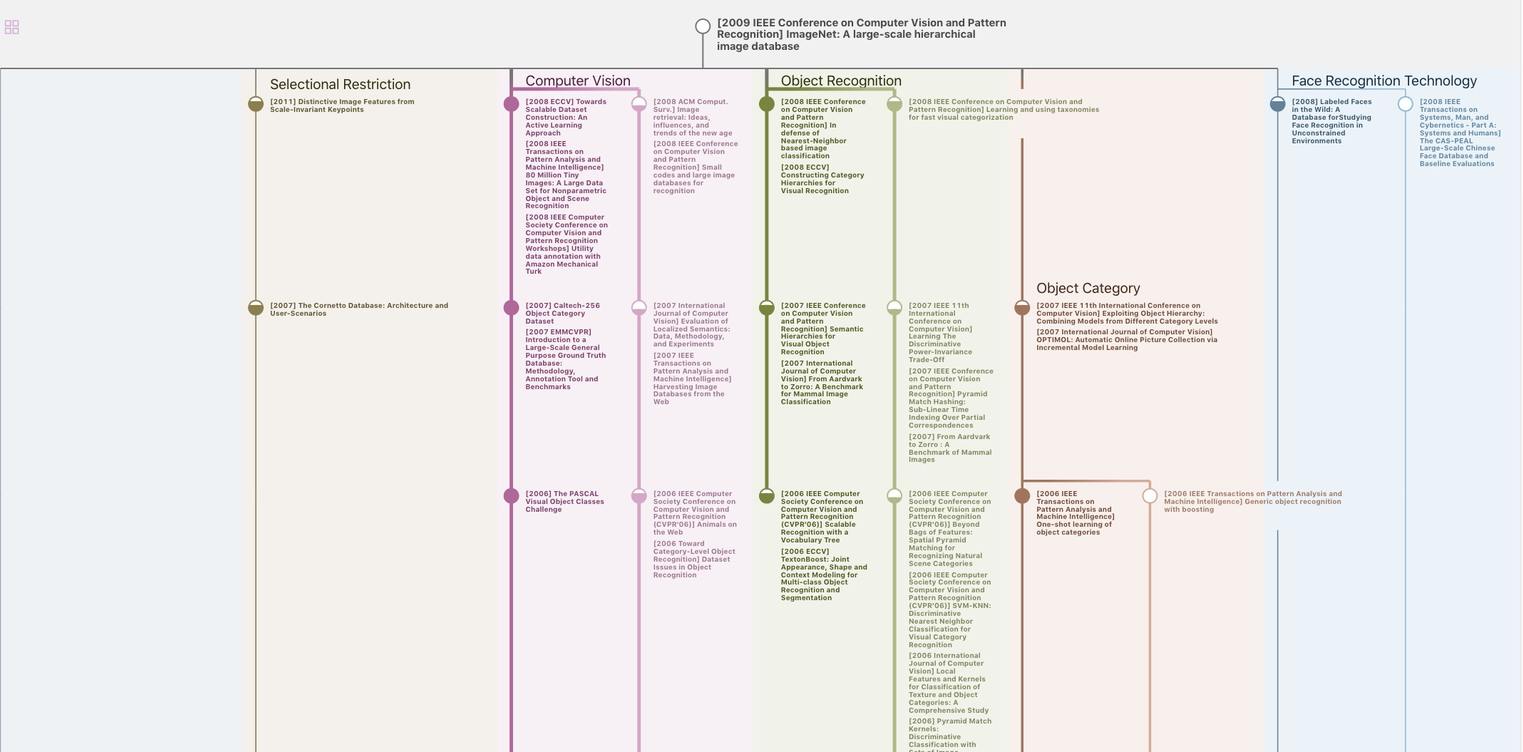
生成溯源树,研究论文发展脉络
Chat Paper
正在生成论文摘要