Mercury: Fast and Optimal Device Placement for Large Deep Learning Models
PROCEEDINGS OF THE 52ND INTERNATIONAL CONFERENCE ON PARALLEL PROCESSING, ICPP 2023(2023)
Abstract
The rapidly expanding neural network models are becoming increasingly challenging to run on a single device. Hence, model parallelism over multiple devices is critical to guaranteeing the efficiency of training large models. Recent proposals either have long processing time or poor performance. Therefore, we propose Mercury, a fast framework for optimizing device placement for large models. Mercury employs a simple but efficient model parallelization strategy in the baseline measurement, and generates placement policies through a series of scheduling algorithms. We conduct experiments to deploy and evaluate Mercury on numerous large models. The results show that Mercury not only reduces the placement policy generation time by 26.4% but also improves the model throughput by 218.5% compared to the most advanced methods.
MoreTranslated text
Key words
Model Parallelism,Optimization,Scheduling
AI Read Science
Must-Reading Tree
Example
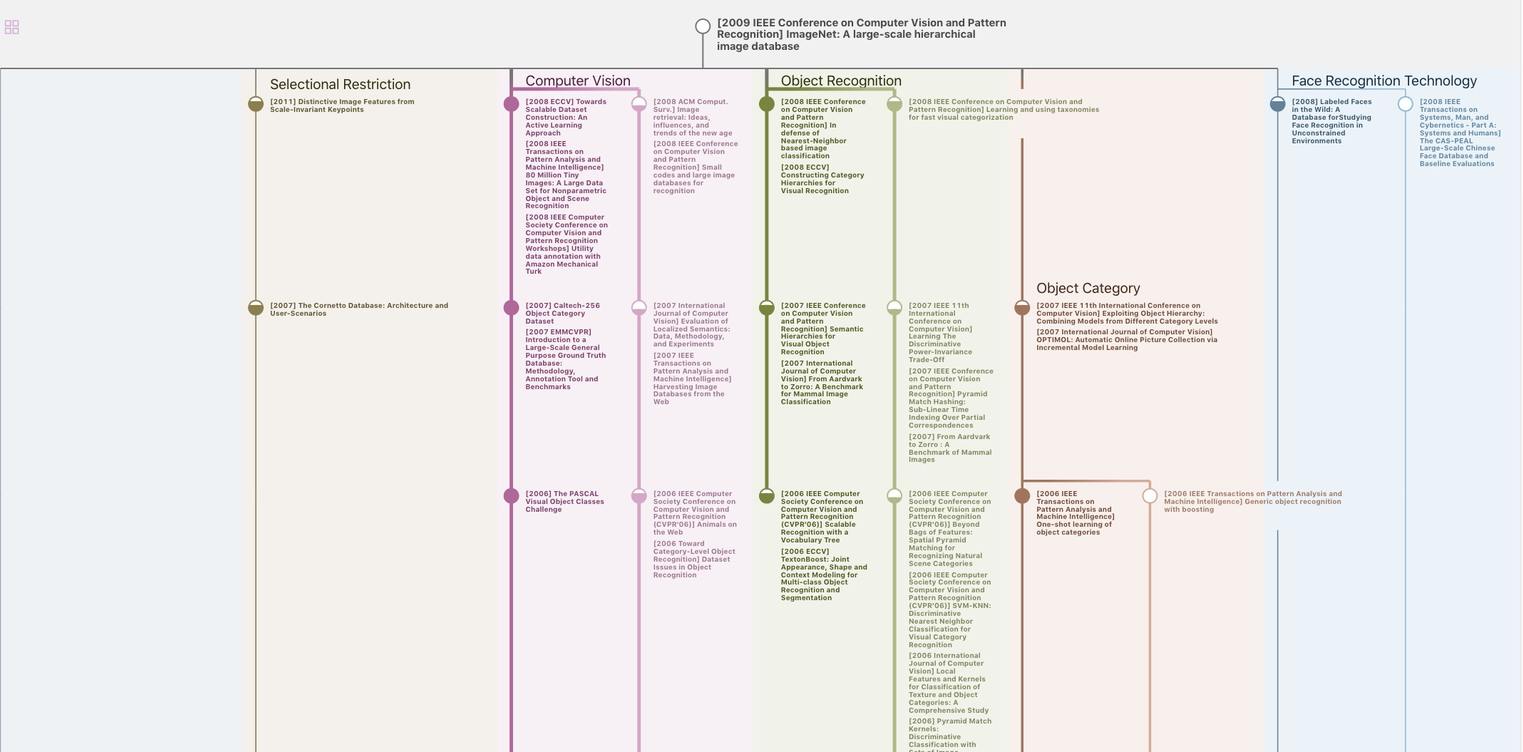
Generate MRT to find the research sequence of this paper
Chat Paper
Summary is being generated by the instructions you defined