Prediction of MOOCs Dropout Based on IQPSO-PLSTM Model.
ICMAI(2023)
摘要
In recent years, Massive Open Online Courses (MOOCs) have become a new way of learning for more and more learners, but the high dropout rate of the MOOCs platform has hindered the further development of MOOCs. Therefore, the research on the dropout prediction is necessary. Previous research on the dropout prediction problem has focused on machine learning. With the rapid development of deep learning, various deep models have been applied to the dropout prediction problem, and have achieved better results than machine learning. However, in the student dropout prediction problem, different student learning behaviors have different effects on the dropout outcome, but the traditional deep models do not consider the variability of different learning behaviors. To solve this problem, this paper combines an Improved Quantum Particle Swarm Optimization algorithm with a deep learning model and proposes the IQPSO-PLSTM model. It first pre-trains the data by random forest and uses the obtained behavioral feature importance as the initial weights of the features. To optimize the initial feature weights, weight finding is then performed using an Improved Quantum Particle Swarm Optimization algorithm. Finally, the weighted data are modeled by deep learning using Peephole LSTM. Experiments on the KDD CUP 2015 dataset show that the AUC of our model can reach 88.45%, which is higher than any other baseline models.
更多查看译文
AI 理解论文
溯源树
样例
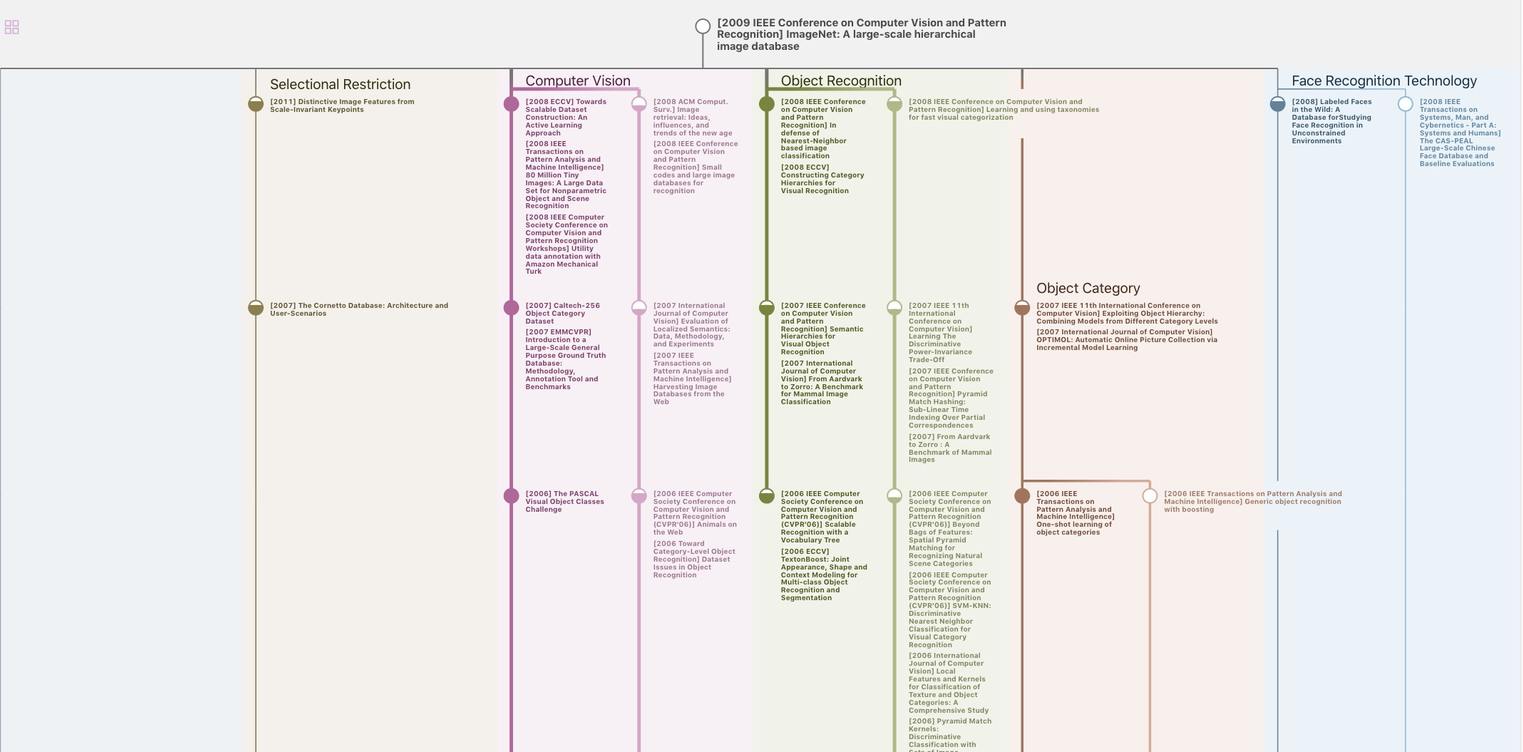
生成溯源树,研究论文发展脉络
Chat Paper
正在生成论文摘要