Learning on Structured Documents for Conditional Question Answering
CHINESE COMPUTATIONAL LINGUISTICS, CCL 2023(2023)
摘要
Conditional question answering (CQA) is an important task in natural language processing that involves answering questions that depend on specific conditions. CQA is crucial for domains that require the provision of personalized advice or making context-dependent analyses, such as legal consulting and medical diagnosis. However, existing CQA models struggle with generating multiple conditional answers due to two main challenges: (1) the lack of supervised training data with diverse conditions and corresponding answers, and (2) the difficulty to output in a complex format that involves multiple conditions and answers. To address the challenge of limited supervision, we propose LSD (Learning on Structured Documents), a self-supervised learning method on structured documents for CQA. LSD involves a conditional question generation method and a contrastive learning objective. The model is trained with LSD on massive unlabeled structured documents and is fine-tuned on labeled CQA dataset afterwards. To overcome the limitation of outputting answers with complex formats in CQA, we propose a pipeline that enables the generation of multiple answers and conditions. Experimental results on the ConditionalQA dataset demonstrate that LSD outperforms previous CQA models in terms of accuracy both in providing answers and conditions.
更多查看译文
AI 理解论文
溯源树
样例
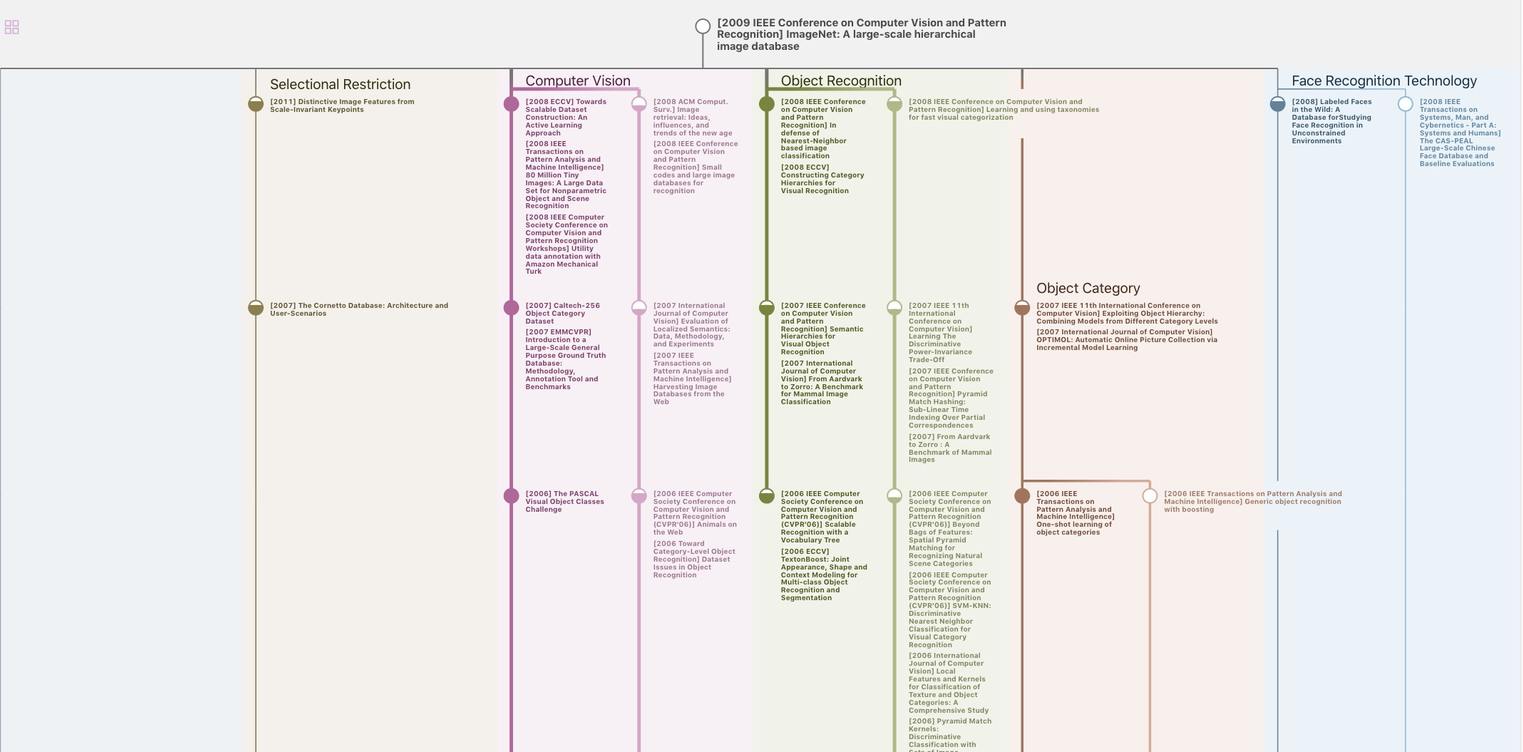
生成溯源树,研究论文发展脉络
Chat Paper
正在生成论文摘要