EQUALS: A Real-world Dataset for Legal Question Answering via Reading Chinese Laws
PROCEEDINGS OF THE 19TH INTERNATIONAL CONFERENCE ON ARTIFICIAL INTELLIGENCE AND LAW, ICAIL 2023(2023)
摘要
Legal Question Answering (LQA) is a promising artificial intelligence application with high practical value. Aprofessional and effective legal question answering (QA) agent can assist in reducing the workload of lawyers and judges, and help to achieve judicial accessibility. However, the NLP community lacks a large-scale LQA dataset with high quality, making it difficult to develop practical data-driven LQA agents. To tackle this bottleneck, this work presents EQUALS, a well-annotated real-world dataset for lEgal QUestion Answering via reading Chinese LawS. EQUALS contains 6,914 {question, article, answer} triplets as well as a pool of articles of laws that covers 10 different collections of Chinese Laws. Questions and the corresponding answers in EQUALS are collected from a professional law consultation forum. More importantly, the exact spans of law articles are annotated by senior law students as the answers. In this way, we could assure the quality and professionalism of EQUALS. Furthermore, thiswork proposes a QA framework that encompasses a law article retrieval module and a machine reading comprehension module for extracting accurate answers from the law article. We conduct thorough experiments with representative baselines on EQUALS, and the results indicate that EQUALS is a challenging question answering task. To the best of our knowledge, EQUALS is the largest real-world LQA dataset which shall significantly promote the research of LQA tasks. The work has been open-sourced and is available at: https://github.com/andongBlue/EQUALS.
更多查看译文
关键词
Legal Dataset,Legal Question Answering,Question Answering Framework
AI 理解论文
溯源树
样例
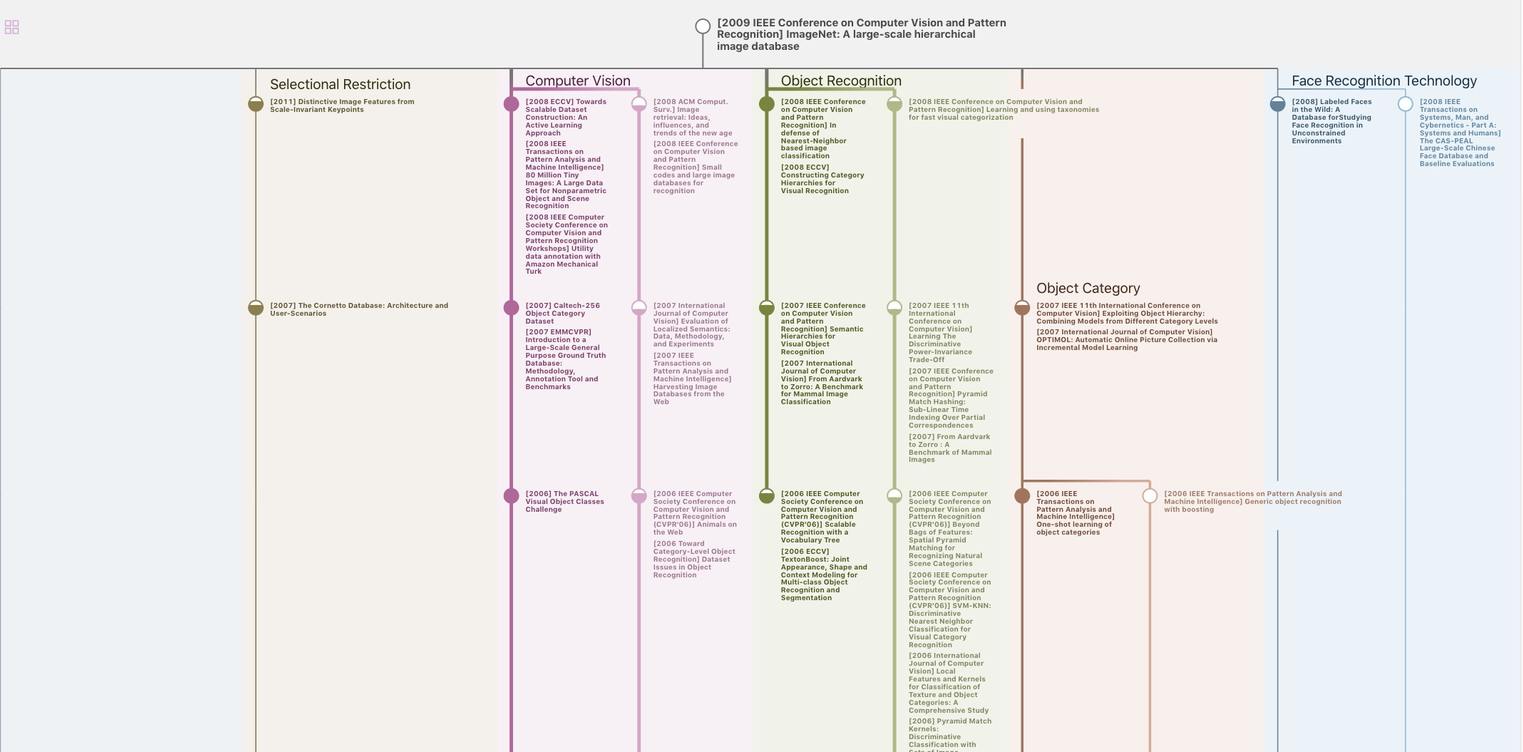
生成溯源树,研究论文发展脉络
Chat Paper
正在生成论文摘要