Predicting Future Location Categories of Users in a Large Social Platform.
ICWSM(2023)
摘要
Understanding the users' patterns of visiting various location categories can help online platforms improve content personalization and user experiences. Current literature on predicting future location categories of a user typically employs features that can be traced back to the user, such as spatial geo-coordinates and demographic identities. Moreover, existing approaches commonly suffer from cold-start and generalization problems, and often cannot specify when the user will visit the predicted location category. In a large social platform, it is desirable for prediction models to avoid using user-identifiable data, generalize to unseen and new users, and be able to make predictions for specific times in the future. In this work, we construct a neural model, LocHabits, using data from Snapchat. The model omits user-identifiable inputs, leverages temporal and sequential regularities in the location category histories of Snapchat users and their friends, and predicts the users' next-hour location categories. We evaluate our model on several real-life, large-scale datasets from Snapchat and FourSquare, and find that the model can outperform baselines by 14.94% accuracy. We confirm that the model can (1) generalize to unseen users from different areas and times, and (2) fall back on collective trends in the cold-start scenario. We also study the relative contributions of various factors in making the predictions and find that the users' visitation preferences and most-recent visitation sequences play more important roles than time contexts, same-hour sequences, and social influence features.
更多查看译文
关键词
future location categories,large social platform,users
AI 理解论文
溯源树
样例
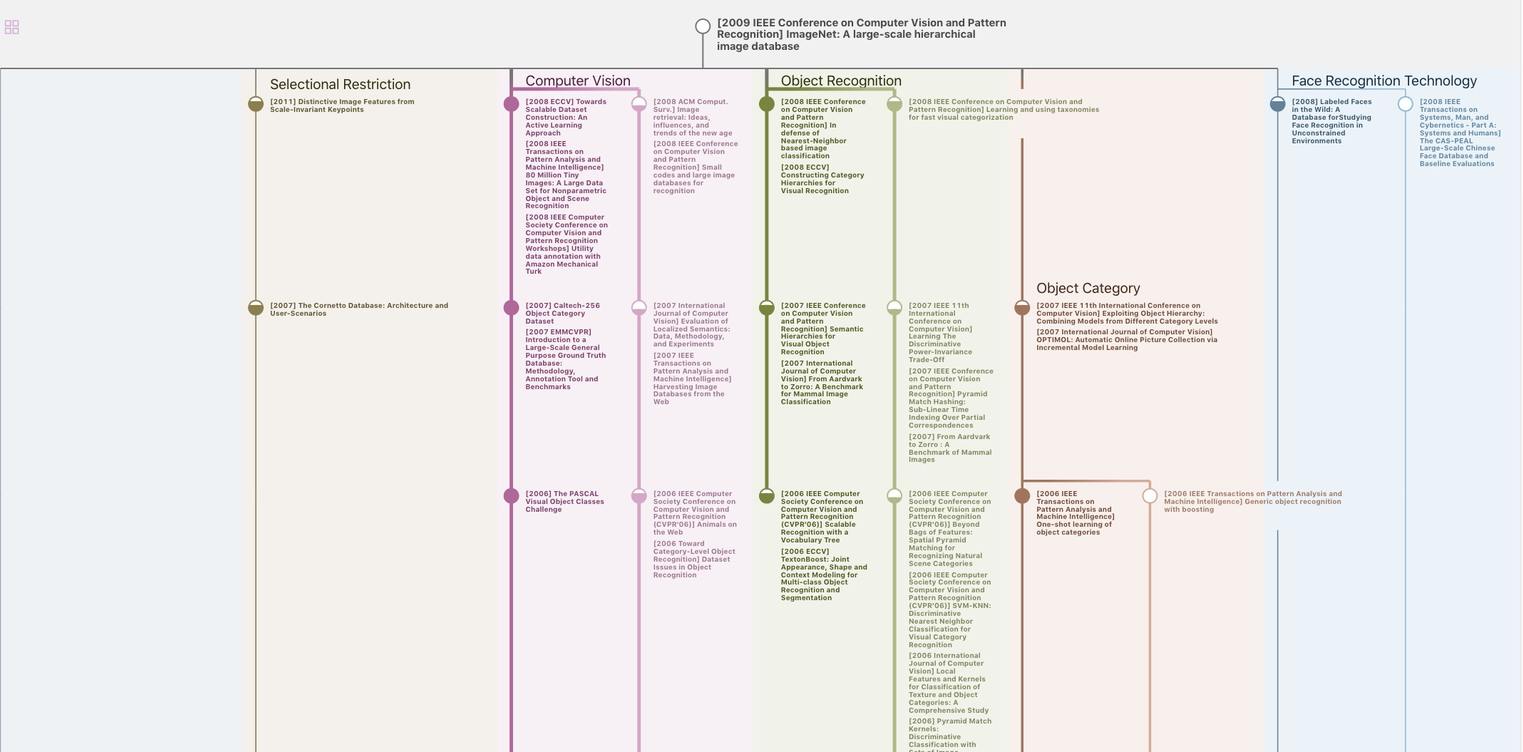
生成溯源树,研究论文发展脉络
Chat Paper
正在生成论文摘要