Achieving Certified Robustness for Brain-Inspired Low-Dimensional Computing Classifiers
INFOCOM Workshops(2023)
摘要
Brain-inspired hyperdimensional computing (HDC) in machine learning applications has been achieving great success in terms of energy efficiency and low latency. The proposal of low-dimensional computing (LDC) classification model not only improves the inference accuracy of existing HDC-based models but also gets rid of the ultra-high dimension in them. However, the security part of LDC model to adversarial perturbations has not been touched. In this paper, we adopt the bounding technique, interval bound propagation (IBP), to train a LDC classification model that is provably robust against
$L_{\infty}$
norm-bounded adversarial attacks. Specifically, we propagate the
$L_{\infty}$
norm-bounded bounding box around the original input through layers of LDC model using interval arithmetic. After propagation, the worst case prediction logits can be computed based on the upper bound and the lower bound of the output bounding box. By minimizing the loss between the worst case prediction and the true label, the predicted label could be kept invariant over all possible adversarial perturbations within
$L_{\infty}$
norm-bounded ball. We evaluate the algorithm on both MNIST and fashion MNIST datasets. The experiment results corroborate that our trained models with IBP exhibit robustness against strong projected gradient descent (PGD) attacks and memory errors.
更多查看译文
关键词
Low-dimensional computing,adversarial attack,certified robustness,interval bound propagation
AI 理解论文
溯源树
样例
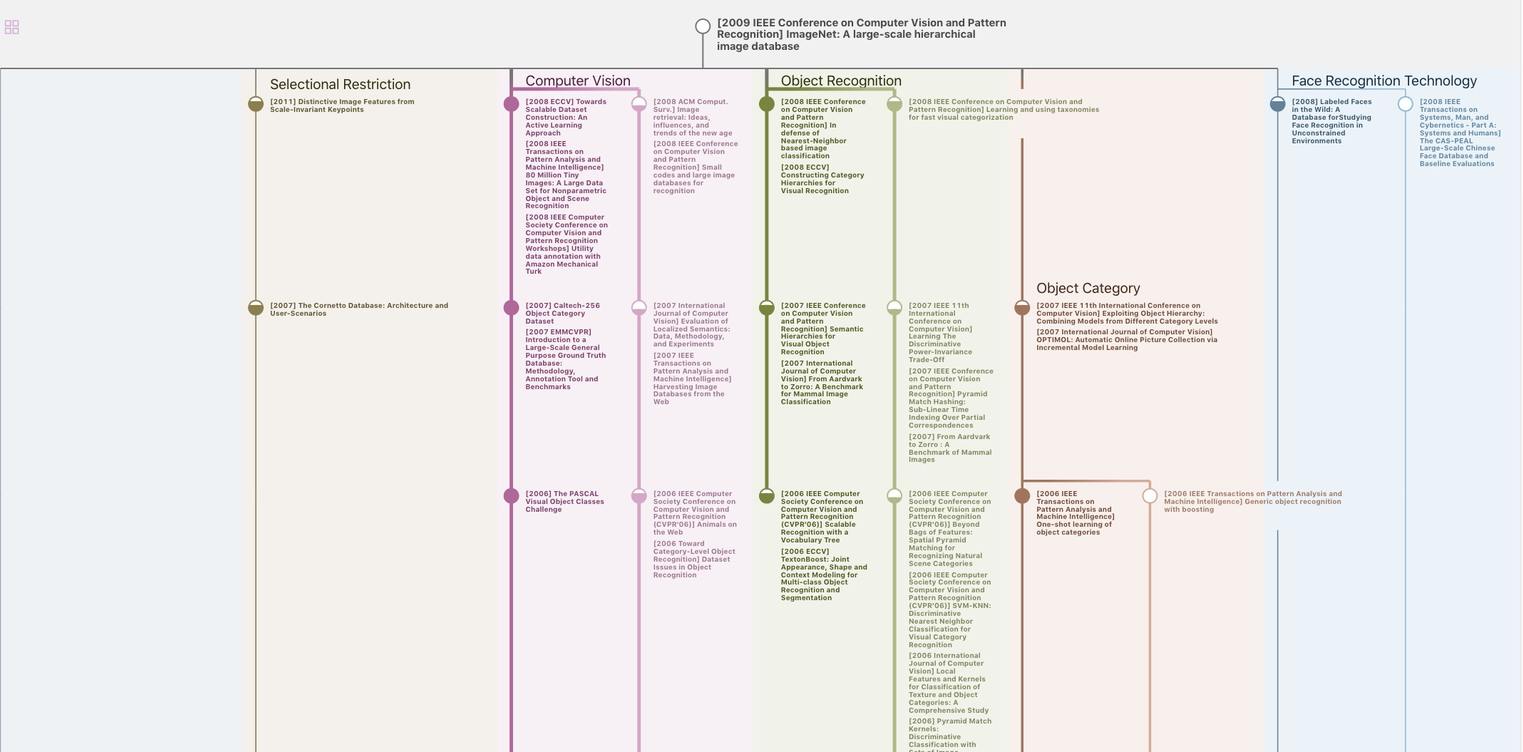
生成溯源树,研究论文发展脉络
Chat Paper
正在生成论文摘要