DAGC: Data-Aware Adaptive Gradient Compression.
INFOCOM(2023)
摘要
Gradient compression algorithms are widely used to alleviate the communication bottleneck in distributed ML. However, existing gradient compression algorithms suffer from accuracy degradation in Non-IID scenarios, because a uniform compression scheme is used to compress gradients at workers with different data distributions and volumes, since workers with larger volumes of data are forced to adapt to the same aggressive compression ratios as others. Assigning different compression ratios to workers with different data distributions and volumes is thus a promising solution. In this study, we first derive a function from capturing the correlation between the number of training iterations for a model to converge to the same accuracy, and the compression ratios at different workers; This function particularly shows that workers with larger data volumes should be assigned with higher compression ratios
1
to guarantee better accuracy. Then, we formulate the assignment of compression ratios to the workers as an n-variables chi-square nonlinear optimization problem under fixed and limited total communication constrain. We propose an adaptive gradient compression strategy called DAGC, which assigns each worker a different compression ratio according to their data volumes. Our experiments confirm that DAGC can achieve better performance facing highly imbalanced data volume distribution and restricted communication.
更多查看译文
关键词
Distributed Machine Learning,Non-IID,Data-aware Adaptive Gradient Compression
AI 理解论文
溯源树
样例
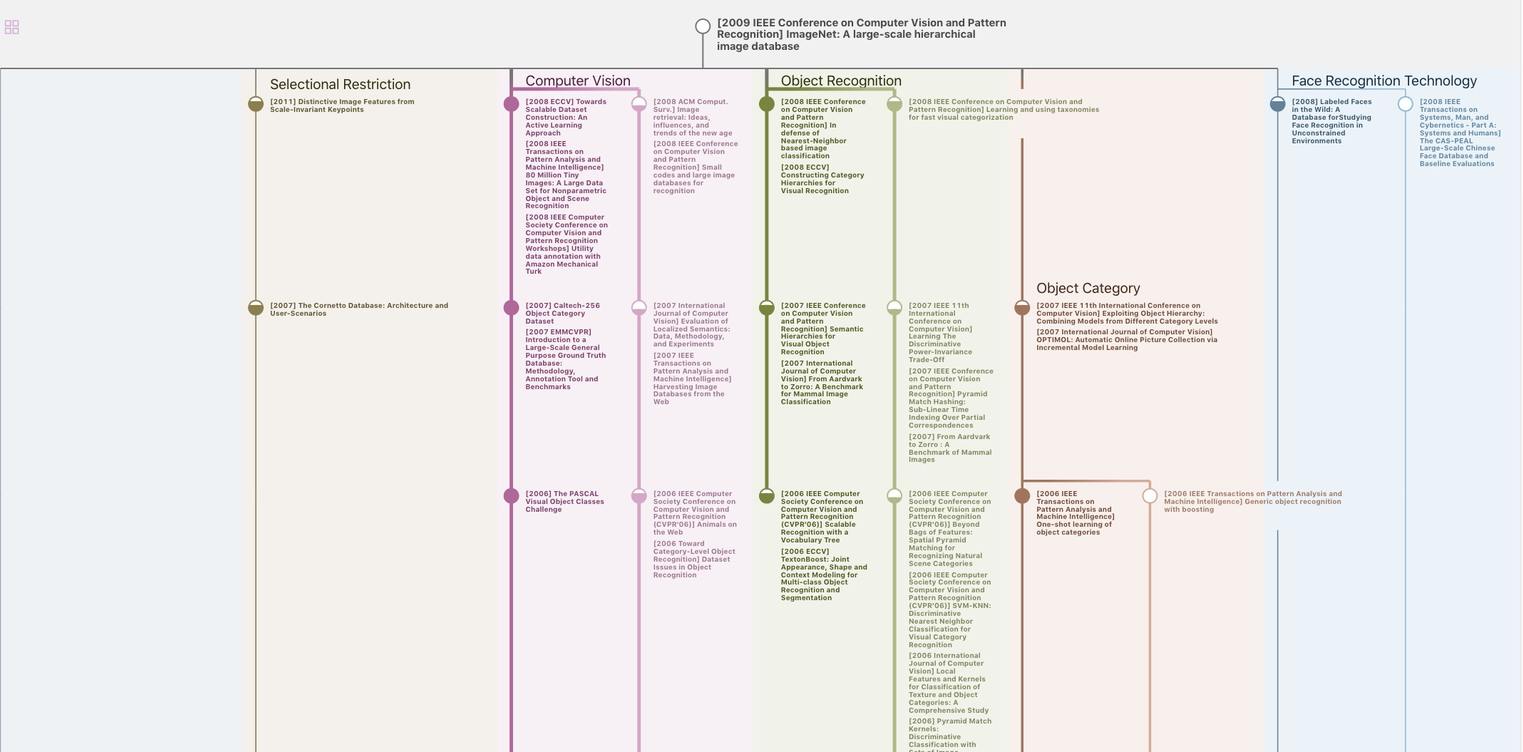
生成溯源树,研究论文发展脉络
Chat Paper
正在生成论文摘要