Combines Contrastive Learning and Primary Capsule Encoder for Target Sentiment Classification.
WISA(2023)
Abstract
Target Sentiment Classification (TSC) aims to judge the sentiment polarity of the specific target appearing in a sentence. Most of the existing TSC algorithms use Recurrent Neural Network (RNN) to encode and model sentences, which can mine the semantic features of sentences, but there are still some shortcomings. RNN cannot fully capture long-distance semantic information, nor can it perform parallel processing calculations. At present, some research attempts to solve the problems of RNN as an encoder, but the generalization ability and prediction ability of these models are low, and there are certain limitations. In view of the above problems, this paper proposes a PC-SCL model that combines contrastive learning and primary capsule encoder. The primary capsule encoder network is designed to extract the hidden state of the word vector of the embedding layer, which can parallel calculate and fully extract and integrate the sentiment features between context and target. In addition, the model uses supervised contrastive learning, which enables the model to extract feature representations more accurately, and improves the generalization and prediction capabilities of the model. The model is tested on three general datasets, and the experimental results prove the effectiveness of the proposed model.
MoreTranslated text
Key words
target sentiment classification,primary capsule encoder,contrastive learning
AI Read Science
Must-Reading Tree
Example
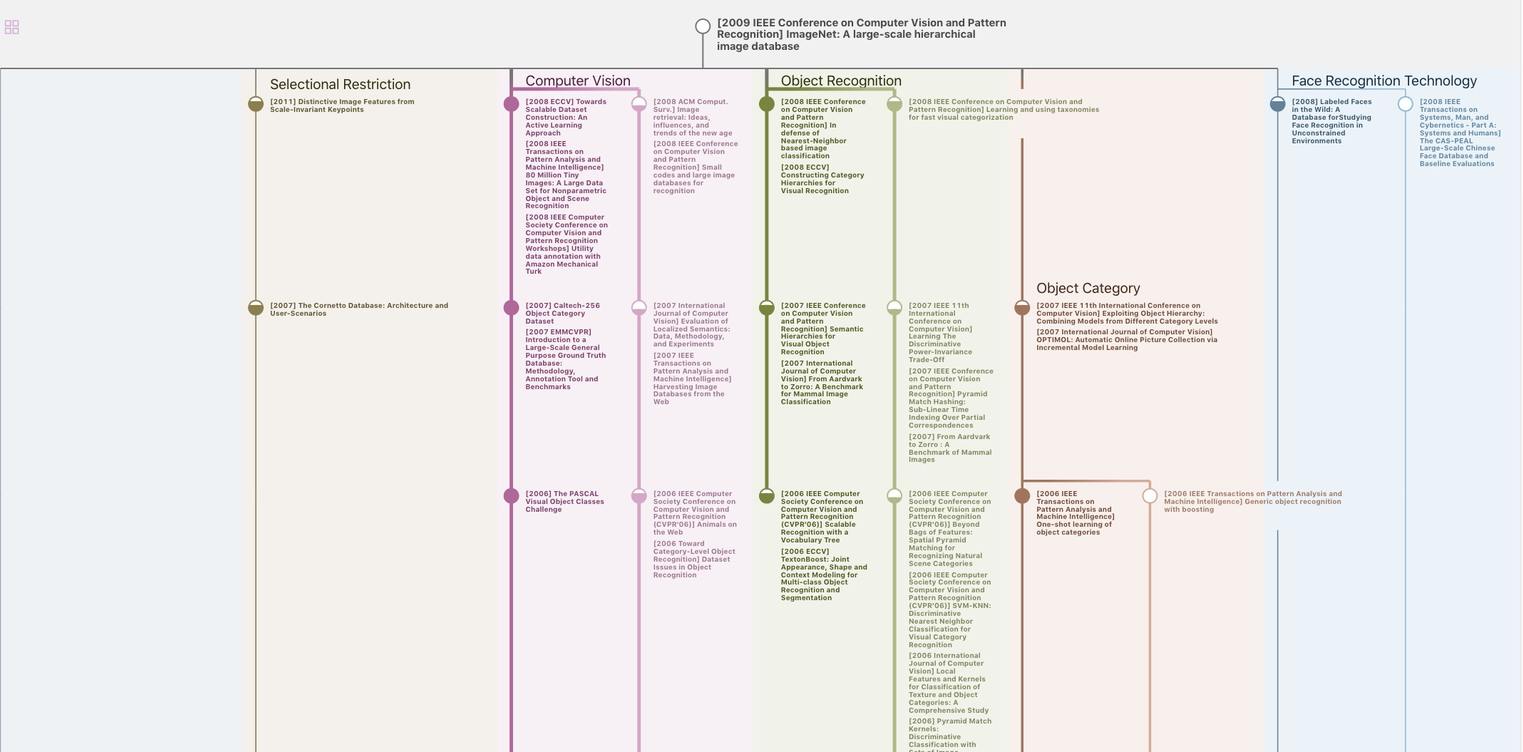
Generate MRT to find the research sequence of this paper
Chat Paper
Summary is being generated by the instructions you defined