Evaluating Augmented Reality, Deep Learning and Paper-Based Assistance Systems in Industrial Manual Assembly.
APMS (1)(2023)
摘要
Augmented reality or deep learning-based methods are increasingly being investigated for use in manufacturing tasks. As manual assembly tasks become more complex and change rapidly, these new technologies offer additional training, cognitive load reduction and error prevention. To realize their potential in industrial applications, technologically simple implementations and real-world experiments are needed. This study aims to explore the potential of deep learning-based object recognition as a driver for modular assembly assistance systems. The proposed deep learning framework and a state-of-the-art augmented reality head-mounted display will be compared for their potential to prevent errors and reduce training time in a real-world assembly task. To ensure that the results are transferable to industrial applications, experiments are conducted on the assembly of a complex product over a full working day of 8 h with a subject size of n = 20. It is found that both the proposed deep learning framework and the augmented reality display drastically reduce the error rate for certain types of errors.
更多查看译文
关键词
augmented reality,deep learning,manual,assembly,paper-based
AI 理解论文
溯源树
样例
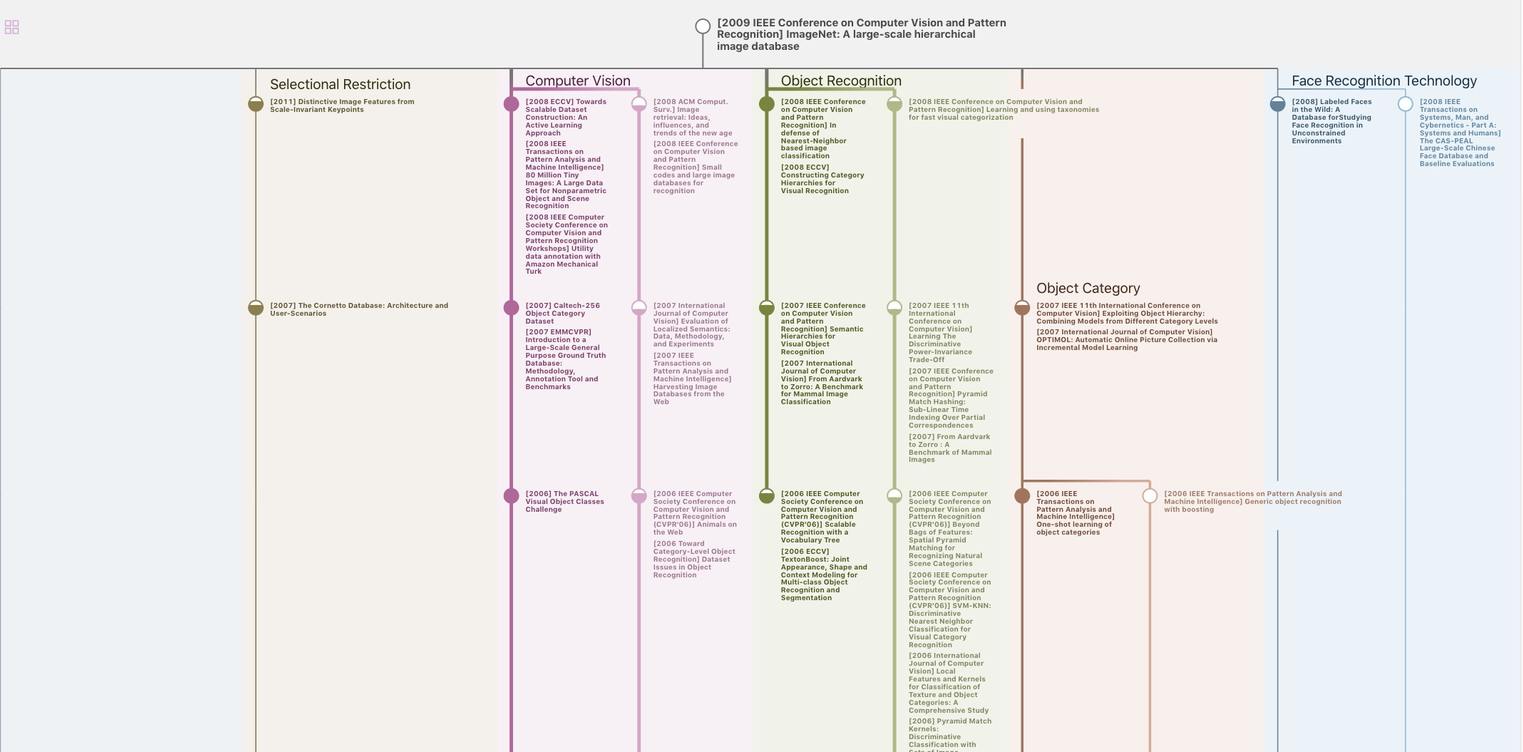
生成溯源树,研究论文发展脉络
Chat Paper
正在生成论文摘要