Evaluating The Effects of Calibrated Popularity Bias Mitigation: A Field Study
PROCEEDINGS OF THE 17TH ACM CONFERENCE ON RECOMMENDER SYSTEMS, RECSYS 2023(2023)
摘要
Despite their proven various benefits, Recommender Systems can cause or amplify certain undesired effects. In this paper, we focus on Popularity Bias, i.e., the tendency of a recommender system to utilize the effect of recommending popular items to the user. Prior research has studied the negative impact of this type of bias on individuals and society as a whole and proposed various approaches to mitigate this in various domains. However, almost all works adopted offline methodologies to evaluate the effectiveness of the proposed approaches. Unfortunately, such offline simulations can potentially be rather simplified and unable to capture the full picture. To contribute to this line of research and given a particular lack of knowledge about how debiasing approaches work not only offline, but online as well, we present in this paper the results of user study on a national broadcaster movie streaming platform in Norway, i.e., TV 2, following the A/B testing methodology. We deployed an effective mitigation approach for popularity bias, called Calibrated Popularity (CP), and monitored its performance in comparison to the platform's existing collaborative filtering recommendation approach as a baseline over a period of almost four months. The results obtained from a large user base interacting in real-time with the recommendations indicate that the evaluated debiasing approach can be effective in addressing popularity bias while still maintaining the level of user interest and engagement.
更多查看译文
关键词
Recommender Systems,Popularity Bias,Long-tail,Online Evaluation,A/B Testing
AI 理解论文
溯源树
样例
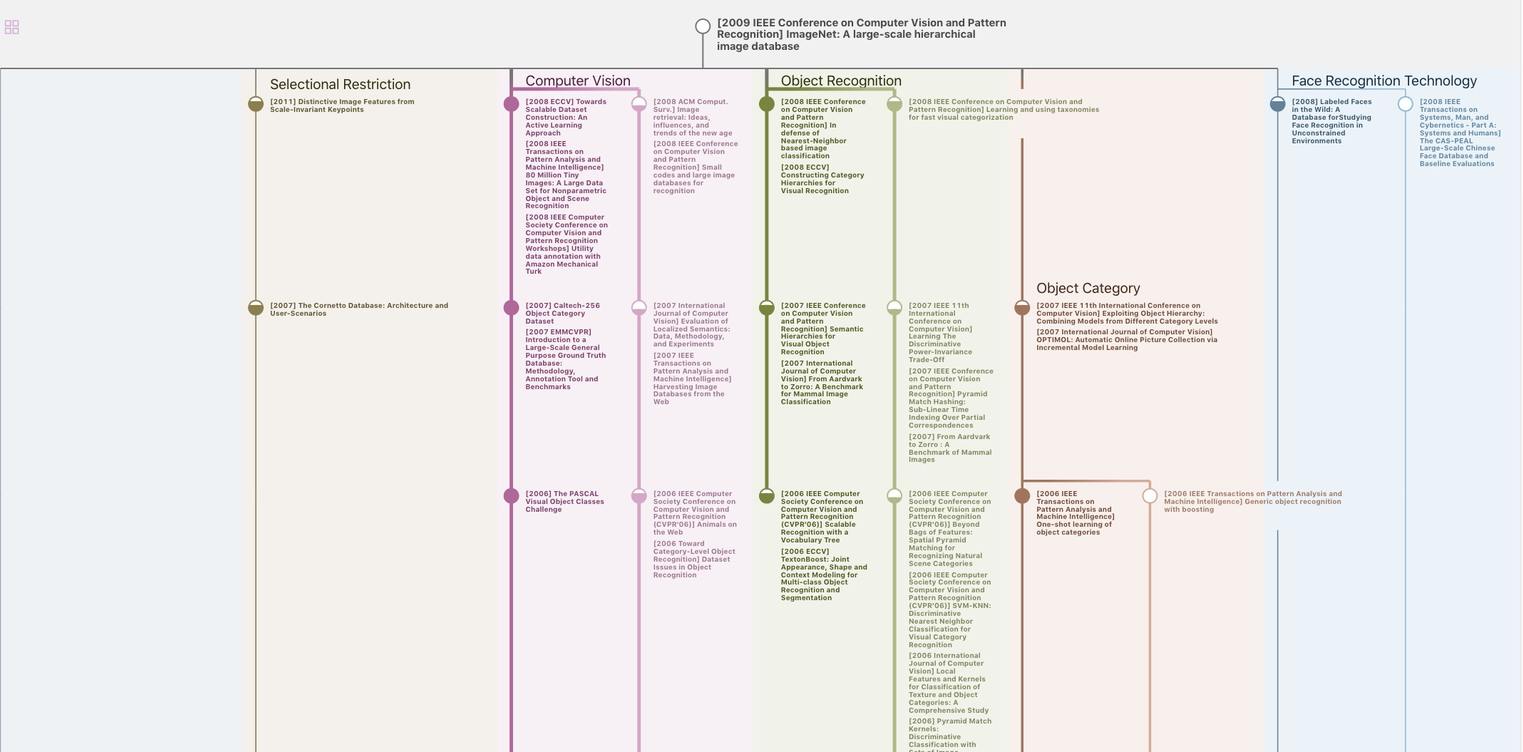
生成溯源树,研究论文发展脉络
Chat Paper
正在生成论文摘要